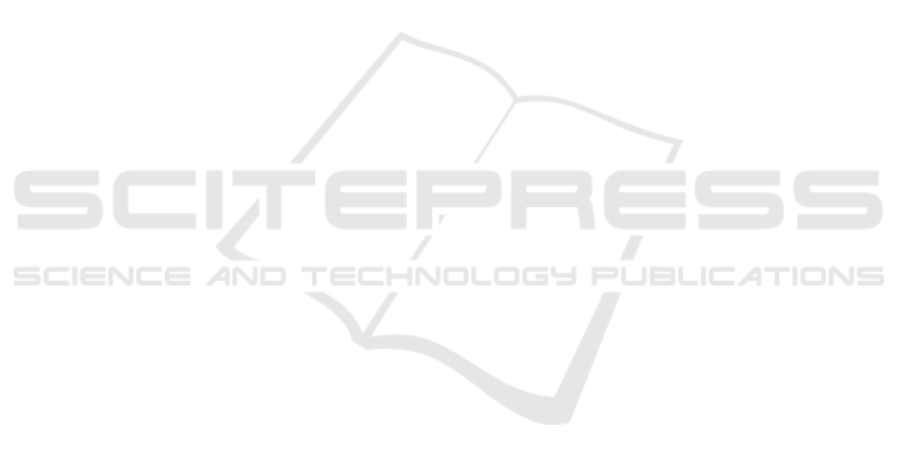
Femminella, M. and Reali, G. (2019). Gossip-based
monitoring of virtualized resources in 5g networks.
In IEEE Conference on Computer Communications
Workshops, INFOCOM Workshops 2019, pages 378–
384. IEEE.
Glossop, C., Panerati, J., Krishnan, A., Yuan, Z., and
Schoellig, A. P. (2022). Characterising the robustness
of reinforcement learning for continuous control using
disturbance injection. In Progress and Challenges in
Building Trustworthy Embodied AI.
He, X., Zhao, K., and Chu, X. (2021). Automl: A sur-
vey of the state-of-the-art. Knowledge-Based Systems,
212:106622.
Hong, C. and Varghese, B. (2019). Resource management
in fog/edge computing: A survey on architectures,
infrastructure, and algorithms. ACM Comput. Surv.,
52(5):97:1–97:37.
Hu, H., Kantardzic, M., and Sethi, T. S. (2020). No free
lunch theorem for concept drift detection in stream-
ing data classification: A review. Wiley Interdisci-
plinary Reviews: Data Mining and Knowledge Dis-
covery, 10(2):e1327.
Jabbari, S., Joseph, M., Kearns, M. J., Morgenstern, J., and
Roth, A. (2016). Fair learning in markovian environ-
ments. CoRR, abs/1611.03071.
Javed, A., Malhi, A., and Främling, K. (2020). Edge
computing-based fault-tolerant framework: A case
study on vehicular networks. In Intl Wireless
Communications and Mobile Computing Conference,
IWCMC 2020. IEEE.
Krajna, A., Brcic, M., Lipic, T., and Doncevic, J. (2022).
Explainability in reinforcement learning: perspective
and position.
Lu, J., Liu, A., Dong, F., Gu, F., Gama, J., and Zhang,
G. (2018). Learning under concept drift: A review.
IEEE Transactions on Knowledge and Data Engineer-
ing, 31(12):2346–2363.
Lwakatare, L. E., Raj, A., Bosch, J., Olsson, H. H., and
Crnkovic, I. (2019). A taxonomy of software engi-
neering challenges for machine learning systems: An
empirical investigation. In Agile Processes in Soft-
ware Engineering and Extreme Programming - 20th
International Conference.
Mendonca, N. C., Jamshidi, P., Garlan, D., and Pahl, C.
(2021). Developing self-adaptive microservice sys-
tems: Challenges and directions. IEEE Software,
38(2):70–79.
Milani, S., Topin, N., Veloso, M., and Fang, F. (2022). A
survey of explainable reinforcement learning.
Mou, Z., Huo, Y., Bai, R., Xie, M., Yu, C., Xu, J., and
Zheng, B. (2022). Sustainable online reinforcement
learning for auto-bidding.
Pahl, C. (2023). Research challenges for machine learning-
constructed software. Serv. Oriented Comput. Appl.,
17(1):1–4.
Pahl, C. and Azimi, S. (2021). Constructing dependable
data-driven software with machine learning. IEEE
Softw., 38(6):88–97.
Pahl, C., Azimi, S., Barzegar, H. R., and El Ioini, N. (2022).
A quality-driven machine learning governance archi-
tecture for self-adaptive edge clouds. In International
Conference on Cloud Computing and Services Science
CLOSER.
Pahl, C., Fronza, I., Ioini, N. E., and Barzegar, H. R. (2019).
A review of architectural principles and patterns for
distributed mobile information systems. In Interna-
tional Conference on Web Information Systems and
Technologies.
Samir, A. and Pahl, C. (2019). DLA: detecting and local-
izing anomalies in containerized microservice archi-
tectures using markov models. In 7th International
Conference on Future Internet of Things and Cloud,
FiCloud 2019, pages 205–213. IEEE.
Samir, A. and Pahl, C. (2021). Autoscaling recovery actions
for container-based clusters. Concurr. Comput. Pract.
Exp., 33(23).
Tokunaga, K., Kawamura, K., and Takaya, N. (2016). High-
speed uploading architecture using distributed edge
servers on multi-rat heterogeneous networks. In IEEE
International Symposium on Local and Metropolitan
Area Networks, LANMAN 2016, pages 1–2. IEEE.
Wan, Z., Xia, X., Lo, D., and Murphy, G. C. (2021). How
does machine learning change software development
practices? IEEE Trans. Software Eng., 47(9):1857–
1871.
Wang, F., Zhang, M., Wang, X., Ma, X., and Liu, J. (2020).
Deep learning for edge computing applications: A
state-of-the-art survey. IEEE Access, 8:58322–58336.
Xu, X., Chen, Y., and Bai, C. (2021). Deep reinforcement
learning-based accurate control of planetary soft land-
ing. Sensors, 21(23).
Zhao, H., Yi, D., Zhang, M., Wang, Q., Xinyue, S., and Zhu,
H. (2019). Multipath transmission workload balanc-
ing optimization scheme based on mobile edge com-
puting in vehicular heterogeneous network. IEEE Ac-
cess, 7:116047–116055.
WEBIST 2023 - 19th International Conference on Web Information Systems and Technologies
364