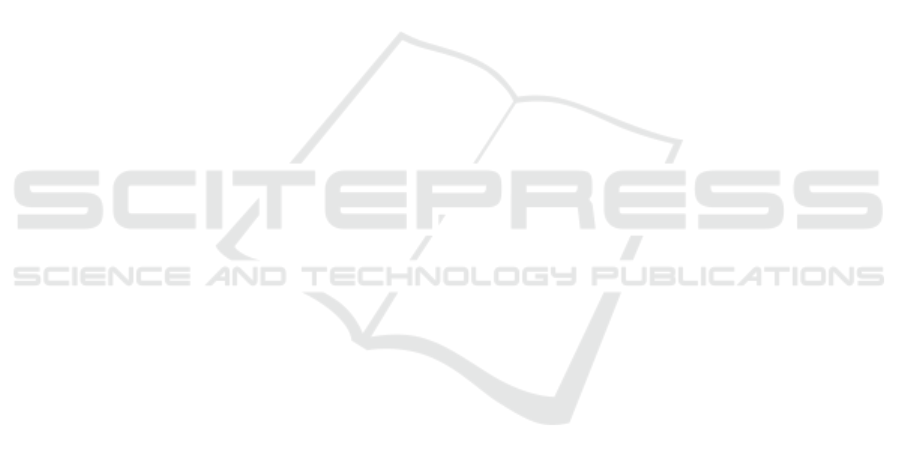
directly from the image information. The modular
and interpretable design of EyeConNet not only al-
lowed us to train it incrementally, keeping the perfor-
mance of the fused network equal to that of their stan-
dalone counterparts, but also allowed seamless inte-
gration with the classic MPC controller, enabling ro-
bust and comparative testing. The developed network
was initially tested in CARLA, then subsequently
tested on real public roads while adhering to safety
requirements. The obtained performance for EyeCon-
Net was satisfactory and in good comparison with
a classical MPC controller. The stable performance
of MPC, when fed with LDVTG outputs, reassures
the stable performance of the perception and planner
modules, thanks to stage-wise training and finetuning
on images obtained from vehicle-specific cameras.
Furthermore, testing during rainy weather and low
visibility conditions, both MPC and EyeConNet were
able to show respectable performance (see Fig. 10)
with minor deterioration, i.e., occasional deactivation
of the control interface due to lack of reference trajec-
tory due to failure of LDVTG. Lastly, a highly com-
petitive runtime of under 50 ms of EyeConNet, on the
one hand, encourages us to consider even more com-
plex models to increase robustness and, on the other
hand, to consider active-closed-loop learning with ei-
ther MPC or driver in the loop. The latter opens up a
lot of interesting research problems, especially in the
area of IL and RL.
ACKNOWLEDGEMENTS
This work was supported by the German Federal Min-
istry of Transport and Digital Infrastructure (BMDV)
within the scope of the project AORTA with the grant
number 01MM20002A.
REFERENCES
Tusimple: Tusimple benchmark. https://github.com/
TuSimple/tusimple-benchmark. Accessed: 2023-03-
15.
Alcala, E., Sename, O., Puig, V., and Quevedo, J. (2020).
Ts-mpc for autonomous vehicle using a learning ap-
proach. IFAC-PapersOnLine, 53(2):15110–15115.
21st IFAC World Congress.
Andersson, J. A. E., Gillis, J., Horn, G., Rawlings, J. B., and
Diehl, M. (2019). CasADi – A software framework
for nonlinear optimization and optimal control. Math-
ematical Programming Computation, 11(1):1–36.
Bansal, M., Krizhevsky, A., and Ogale, A. S. (2019). Chauf-
feurnet: Learning to drive by imitating the best and
synthesizing the worst. In Robotics: Science and Sys-
tems XV, University of Freiburg, Freiburg im Breisgau,
Germany, June 22-26, 2019.
Barron, J. T. (2019). A general and adaptive robust loss
function. CVPR.
Bojarski, M., Testa, D. D., and et al. (2016). End to end
learning for self-driving cars. CoRR, abs/1604.07316.
Chen, J., Li, S. E., and Tomizuka, M. (2022). Interpretable
end-to-end urban autonomous driving with latent deep
reinforcement learning. IEEE Transactions on Intelli-
gent Transportation Systems, 23(6):5068–5078.
Codevilla, F., M
¨
uller, M., L
´
opez, A. M., Koltun, V., and
Dosovitskiy, A. (2018). End-to-end driving via condi-
tional imitation learning. In International Conference
on Robotics and Automation, ICRA, pages 1–9. IEEE.
Codevilla, F., Santana, E., L
´
opez, A. M., and Gaidon, A.
(2019). Exploring the limitations of behavior cloning
for autonomous driving. In International Conference
on Computer Vision, ICCV 2019, pages 9328–9337.
IEEE.
Hecker, S., Dai, D., and Gool, L. V. (2018). End-to-end
learning of driving models with surround-view cam-
eras and route planners. In European Conference on
Computer Vision, ECCV, volume 11211 of Lecture
Notes in Computer Science, pages 449–468. Springer.
Ji, J., Khajepour, A., Melek, W. W., and Huang, Y. (2017).
Path planning and tracking for vehicle collision avoid-
ance based on model predictive control with multicon-
straints. IEEE Transactions on Vehicular Technology,
66(2):952–964.
Kabzan, J., Hewing, L., Liniger, A., and Zeilinger, M. N.
(2019). Learning-based model predictive control for
autonomous racing. IEEE Robotics and Automation
Letters, 4(4):3363–3370.
Kabzan, J., Valls, M. I., and et al. (2020). Amz driverless:
The full autonomous racing system. Journal of Field
Robotics, 37(7):1267–1294.
Kim, M., Lee, D., Ahn, J., Kim, M., and Park, J. (2021).
Model predictive control method for autonomous ve-
hicles using time-varying and non-uniformly spaced
horizon. IEEE Access, 9:86475–86487.
LeCun, Y., Muller, U., and et al. (2005). Off-road obstacle
avoidance through end-to-end learning. In Advances
in Neural Information Processing Systems 18, NIPS,
pages 739–746.
Natan, O. and Miura, J. (2023). End-to-end autonomous
driving with semantic depth cloud mapping and multi-
agent. IEEE Trans. Intell. Veh., 8(1):557–571.
Paden, B.,
ˇ
C
´
ap, M., and et al. (2016). A survey of motion
planning and control techniques for self-driving urban
vehicles. IEEE Transactions on Intelligent Vehicles,
1.
Pomerleau, D. (1988). ALVINN: an autonomous land ve-
hicle in a neural network. In Touretzky, D. S., editor,
Advances in Neural Information Processing Systems
1, [NIPS Conference, Denver, Colorado, USA, 1988],
pages 305–313. Morgan Kaufmann.
Qin, Z., Wang, H., and Li, X. (2020). Ultra fast structure-
aware deep lane detection. In European Conference
on Computer Vision, pages 276–291. Springer.
Learning Based Interpretable End-to-End Control Using Camera Images
483