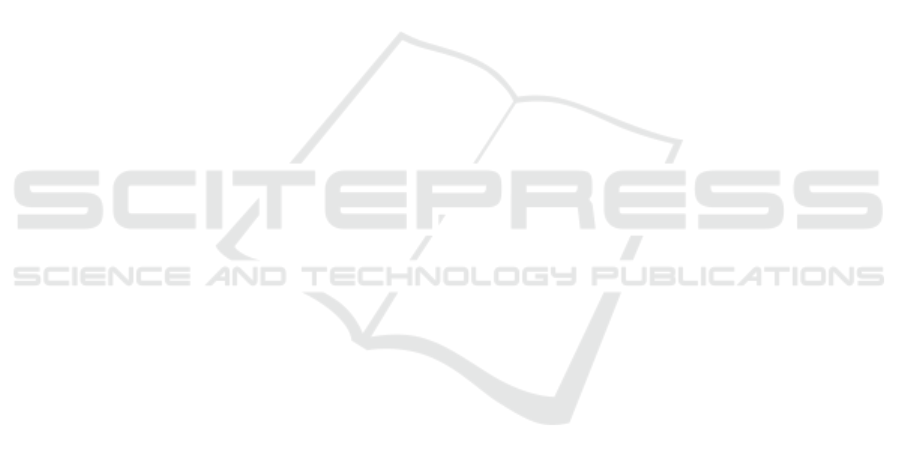
management, highlighting their potential to provide
comprehensive insights and support effective deci-
sion making. Digital Twins offer a promising solu-
tion to the pressing issue of urban traffic congestion
by integrating real-time data, simulation models, and
analytics to create dynamic digital replicas of urban
transport networks. This technology enables Smart
Cities to gain a holistic view of traffic dynamics, op-
timize traffic flow, and develop intelligent strategies
to alleviate congestion. By leveraging Digital Twins,
cities can enhance their transportation systems, im-
prove quality of life for residents, and move closer to
their goals of sustainability and efficiency. Through-
out the paper, authors have advocated for the adop-
tion and further exploration of Digital Twins in traf-
fic management. The integration of Trajectory Min-
ing, Process Mining, and Decision Making techniques
within the Digital Twin framework presents exciting
opportunities to optimize traffic management strate-
gies, predict traffic patterns, evaluate infrastructure
changes, and enhance public safety. By embracing
Digital Twins, Smart Cities can embrace innovative
approaches and leverage advanced technologies to
tackle the complexities of urban traffic congestion. In
summary, Digital Twins have the potential to revolu-
tionize traffic management in Smart Cities, paving the
way for more efficient, sustainable, and livable urban
environments. While challenges and considerations
exist, such as data privacy and security, infrastructure
requirements, and stakeholder engagement, the ben-
efits offered by Digital Twins outweigh these obsta-
cles. It is essential for policymakers, urban planners,
and researchers to recognize the transformative power
of Digital Twins and work collaboratively to harness
this technology’s full potential in shaping the future
of traffic management in Smart Cities. By embracing
Digital Twins, we can build smarter, more connected,
and more efficient urban transportation systems that
enhance the quality of life for residents and pave the
way for a sustainable future.
REFERENCES
Batty, M. (2018). Digital twins.
Beerepoot, I., Di Ciccio, C., Reijers, H. A., Rinderle-Ma,
S., Bandara, W., Burattin, A., Calvanese, D., Chen,
T., Cohen, I., Depaire, B., et al. (2023). The biggest
business process management problems to solve be-
fore we die. Computers in Industry, 146:103837.
Brockhoff, T., Heithoff, M., Koren, I., Michael, J., Pfeiffer,
J., Rumpe, B., Uysal, M. S., Van Der Aalst, W. M. P.,
and Wortmann, A. (2021). Process prediction with
digital twins. In 2021 ACM/IEEE International Con-
ference on Model Driven Engineering Languages and
Systems Companion (MODELS-C), pages 182–187.
Costa, G., Manco, G., and Masciari, E. (2014). Dealing
with trajectory streams by clustering and mathemati-
cal transforms. Journal of Intelligent Information Sys-
tems, 42(1):155–177.
Delgado, A. and Calegari, D. (2023). Process mining for
improving urban mobility in smart cities: Challenges
and application with open data.
Deren, L., Wenbo, Y., and Zhenfeng, S. (2021). Smart city
based on digital twins. Computational Urban Science,
1:1–11.
Ding, Y., Zhang, Z., Chen, K., Ding, H., Voss, S., Heilig,
L., Chen, Y., Chen, X., et al. (2023). Real-time mon-
itoring and optimal resource allocation for automated
container terminals: A digital twin application at the
yangshan port. Journal of Advanced Transportation,
2023.
Djahel, S., Doolan, R., Muntean, G.-M., and Murphy, J.
(2014). A communications-oriented perspective on
traffic management systems for smart cities: Chal-
lenges and innovative approaches. IEEE Communi-
cations Surveys & Tutorials, 17(1):125–151.
Du, H., Leng, S., He, J., and Zhou, L. (2021). Digital twin
based trajectory prediction for platoons of connected
intelligent vehicles. In 2021 IEEE 29th International
Conference on Network Protocols (ICNP), pages 1–6.
IEEE.
d’Ajello, E., Formica, D., Masciari, E., Mattia, G., Anni-
ciello, A., Moscariello, C., Quintarelli, S., and Zac-
carella, D. (2022). Cluster algorithm for social choice.
In Joint European Conference on Machine Learning
and Knowledge Discovery in Databases, pages 227–
237. Springer.
El Saddik, A. (2018). Digital twins: The conver-
gence of multimedia technologies. IEEE multimedia,
25(2):87–92.
Fan, Z., Yang, X., Yuan, W., Jiang, R., Chen, Q., Song, X.,
and Shibasaki, R. (2022). Online trajectory predic-
tion for metropolitan scale mobility digital twin. In
Proceedings of the 30th International Conference on
Advances in Geographic Information Systems, pages
1–12.
Feng, H., Lv, H., and Lv, Z. (2023). Resilience towarded
digital twins to improve the adaptability of transporta-
tion systems. Transportation Research Part A: Policy
and Practice, 173:103686.
Fioretto, S. (2023). Process mining solutions for public ad-
ministration. In European Conference on Advances in
Databases and Information Systems, pages 668–675.
Springer.
Gao, C., Wang, J., Dong, S., Liu, Z., Cui, Z., Ma, N., and
Zhao, X. (2022). Application of digital twins and
building information modeling in the digitization of
transportation: A bibliometric review. Applied Sci-
ences, 12(21):11203.
Jadri
´
c, M., Pa
ˇ
sali
´
c, I. N., and
´
Cuku
ˇ
si
´
c, M. (2020). Pro-
cess mining contributions to discreteevent simulation
modelling. Business Systems Research: International
Digital Twins for Traffic Congestion in Smart Cities: A Novel Solution Using Data Mining Techniques
247