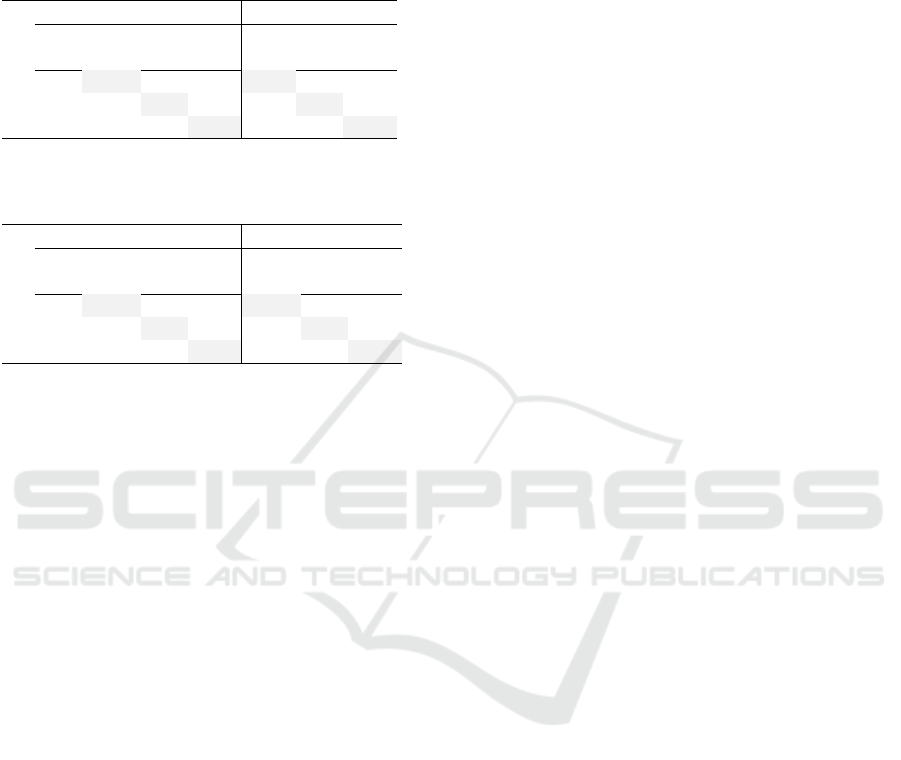
SUAS based on Naive Bayes. In this case, both SUAS
provided the same results.
Table 6: Confusion matrix of FGeomNB (left) and Ge-
omNB (right) assessment methods.
FGeomNB GeomNB
Real class
Assigned Class Assigned Class
C1 C2 C3 C1 C2 C3
C1 156 2 0 158 0 0
C2 0 82 64 0 47 99
C3 0 18 128 0 1 145
Table 7: Confusion matrix of FNB (left) and NB (right)
assessment methods.
FNB NB
Real class
Assigned Class Assigned Class
C1 C2 C3 C1 C2 C3
C1 157 1 0 157 1 0
C2 95 10 41 95 10 41
C3 38 3 105 38 3 105
In summary, it is found that the proposed network
based on the geometric distribution achieved good
correct allocations for the data used in this study. The
network surpassed previously proposed networks in
the literature such as Naive Bayes and Fuzzy Naive
Bayes, indicating that they can serve as viable alter-
natives for assessment methods.
6 CONCLUSION
In this paper, a novel approach called the Fuzzy Geo-
metric Naive Bayes Network was introduced to han-
dle multidimensional intervals by modeling them us-
ing geometric distributions. This network served as
the foundation for SUAS specifically designed for
Virtual Reality (VR) simulators, such as SITEG 2.0.
Simulations were conducted using data that fol-
lowed a geometric distribution and compared against
Naive Bayes and Fuzzy Naive Bayes SUAS. The sim-
ulation results demonstrated that the SUAS based on
the geometric distribution has superior discrimina-
tion capabilities, outperforming the traditional Naive
Bayes and Fuzzy Naive Bayes approaches.
Moreover, the Fuzzy Geometric Naive Bayes Net-
work proposed in this study can also be effectively
utilized for datasets that involve intersections with
values close to zero.
ACKNOWLEDGEMENTS
This research is supported by the National Coun-
cil for Scientific and Technological Development -
CNPq (Grants 305914/2021-9 and 315298/2018-9)
and Fundac¸
˜
ao de Apoio
`
a Pesquisa do Estado da
Para
´
ıba - FAPESQ-PB.
REFERENCES
Cohen, J. (1960). A coefficient of agreement for nominal
scales. Educational and psychological measurement,
20(1):37–46.
Ferreira, J. A. and Moraes, R. M. (2023). Fuzzy-
class: A family of fuzzy and non-fuzzy probabilistic-
based classifiers. Journal of Open Source Software,
8(88):5613.
Landis, J. R. and Koch, G. G. (1977). The measurement of
observer agreement for categorical data. biometrics,
pages 159–174.
Mendenhall, W., Beaver, R. J., and Beaver, B. M. (2012).
Introduction to Probability and Statistics. Cengage
Learning, 14th edition.
Moraes, R. and Machado, L. (2009). Online training eval-
uation in virtual reality simulators using possibilistic
networks. In Proc. Safety Health and Environmental
World Congress, pages 67–71. Citeseer.
Moraes, R., Silva, I. L. A., and Machado, L. (2020). Online
skills assessment in training based on virtual reality
using a novel fuzzy triangular naive bayes network.
In Proc. FLINS, pages 446–454. World Scientific.
Moraes, R. M., Ferreira, J. A., and Machado, L. S. (2021).
A new bayesian network based on gaussian naive
bayes with fuzzy parameters for training assessment
in virtual simulators. International Journal of Fuzzy
Systems, 23(3):849–861.
Moraes, R. M. and Machado, L. S. (2012). A new architec-
ture for assessment of multiple users in collaborative
medical training environments based on virtual real-
ity. In Uncertainty Modeling in Knowledge Engineer-
ing and Decision Making, pages 1119–1124. World
Scientific.
Moraes, R. M. and Machado, L. S. (2015). A fuzzy poisson
naive bayes classifier for epidemiological purposes. In
2015 7th International Joint Conference on Computa-
tional Intelligence (IJCCI), volume 2, pages 193–198.
IEEE.
Moraes, R. M. and Machado, L. S. (2016). A fuzzy bino-
mial naive bayes classifier for epidemiological data.
In 2016 IEEE International Conference on Fuzzy Sys-
tems (FUZZ-IEEE), pages 745–750. IEEE.
Souza, D. F., Valdek, M. C., Moraes, R. M., and Machado,
L. S. (2006). Siteg–sistema interativo de treinamento
em exame ginecol
´
ogico. In VIII Symposium on Virtual
Reality SVR, volume 12.
Zadeh, L. A. (1968). Probability measures of fuzzy events.
Journal of mathematical analysis and applications,
23(2):421–427.
A Novel Fuzzy Geometric Naive Bayes Network for Online Skills Assessment in Training Based on Virtual Reality
401