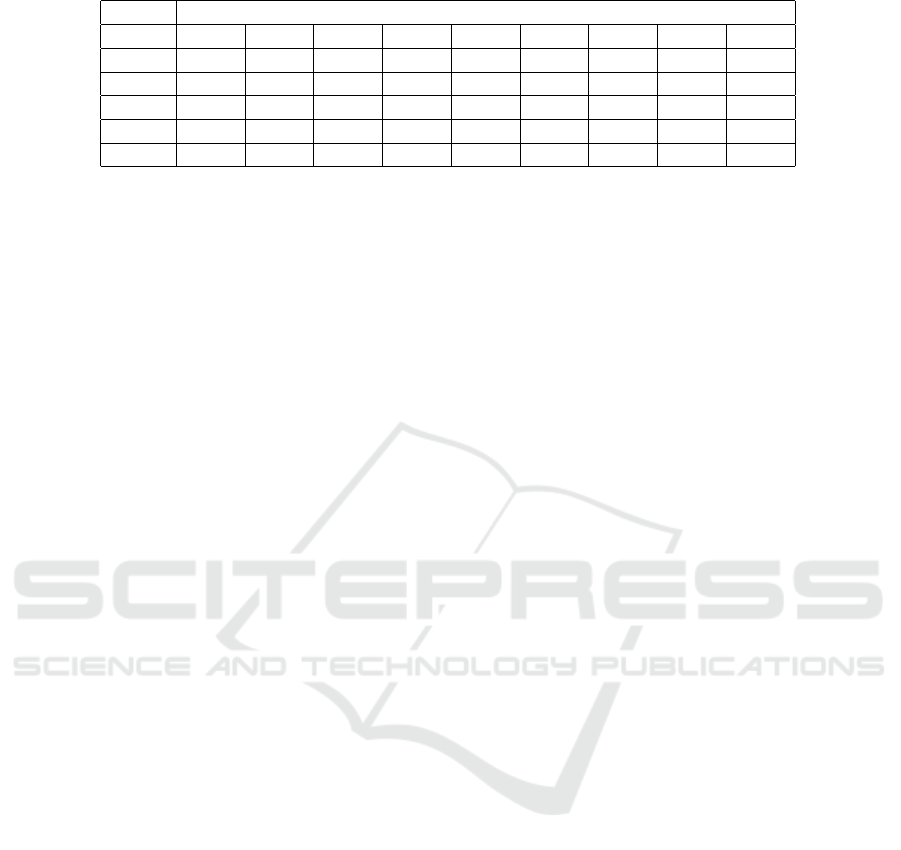
Table 2: Detailed values of experiment 2.
distance [m]
true 0.4 0.6 0.8 1.0 1.2 1.4 1.6 1.8 2.0
light 1 0.338 0.550 0.739 0.878 1.075 1.295 1.572 1.788 2.092
light 2 0.325 0.456 0.740 0.980 1.056 1.382 1.510 1.700 1.871
light 3 0.339 0.444 0.747 1.009 1.163 1.486 1.619 1.636 1.812
light 4 0.323 0.462 0.752 0.924 1.078 1.315 1.494 1.727 1.849
light 5 0.300 0.376 0.791 1.096 1.163 1.418 1.532 1.730 2.010
is the same as in Experiment 1. Based on Figure 7, it
can be observed that the overall inferences are correct.
In addition, according to Table 2 the largest error was
approximately 0.22m, and the average error across all
measurements was within 0.08m.
From these result, It was found that doubling the
interval between data to be acquired approximately
doubles the error.
4.3.3 Experiment 3
The figure and table are omitted because this experi-
ment yielded identical results to Experiment 1. Based
on the findings from this experiment, it was observed
that the trained model generated this time is not re-
liant on light intensity.
5 CONCLUSIONS
This paper presents the results of a deep learning
model for estimating the distance of multiple lighting
devices. In Experiment 1, the maximum error was ap-
proximately 0.1m. This indicates the generation of a
useful learning model. Experiment 2 exhibited an av-
erage error of approximately 0.2m, which was slightly
larger than the error in Experiment 1 but overall dis-
tance estimation remained accurate. Experiment 3 re-
vealed that the learning model did not capture the in-
tensity of light.
Based on the above findings, the model demon-
strated the ability to estimate distances effectively,
providing practical applicability. However, because
the inference results were derived from static data, the
actual sensitivity of the model has yet to be verified.
Therefore, we plan to verify the effectiveness of this
method in real-time scenarios.
This method also presents a challenge due to the
time-consuming nature of preparing training data,
as it requires multiple shots for each illumination.
Therefore, our goal is to develop a method that sim-
plifies the process of preparing training data.
ACKNOWLEDGEMENTS
This work was supported by JSPS Grant-in-Aid for
Scientific Research JP20K12016.
REFERENCES
Ban, R., Kaji, K., and Kawaguchi, N. (2014). Indoor po-
sition estimation method using pedestrian dead reck-
oning in conjunction with magnetic and wifi signal
strength map information. Institute of Electronics,
Information and Communication Engineers Technical
Report, 113:23–28.
Chenavier, F. and Crowley, J. (1992). Position estimation
for a mobile robot using vision and odometry. In
ICRA, volume 89, pages 2588–2593.
Fujihara, M., Nakatani, T., Maekawa, T., and Hara, T.
(2020). Investigation of collaborative indoor position-
ing method for iot devices using gps signal informa-
tion and bluetooth radio wave information. Research
Report on Ageing Society Design (ASD), (32):1–8.
Fushimi, S., Makino, H., and Nagazoe, K. (2009). Floures-
cent light-based position information system for the
visually impaired. In Panasonic Electric Works Tech-
nical Report, volume 57, pages 53–59.
Kobayashi, H. (2019). Cepheid: the infrastructure-less in-
door localization using lighting fixtures’ acoustic fre-
quency fingerprints. In IECON 2019-45th Annual
Conference of the IEEE Industrial Electronics Soci-
ety, volume 1, pages 6842–6847.
Kobayashi, H. (2020). Improvement of the lighting fixtures
based indoor localization method cepheid. In 2020
IEEE Asia-Pacific Conference on Computer Science
and Data Engineering (CSDE), pages 1–6.
Tomonou, M. (2016). Environment recognition for mo-
bile robots -map construction and self-position esti-
mation. Special Issue on “Robotics in the Face of Un-
certainty”, 60:509–514.
Yamano, R. and Kobayashi, H. (2017a). A preliminary re-
search for a new self-localization method using fre-
quency characteristics of illumination light. In Pro-
ceedings of the 60th Joint Automatic Control Confer-
ence, pages FrSP1–2.
Yamano, R. and Kobayashi, H. (2017b). A study on self-
localization method based on individual difference of
A Study on Acquisition of 3D Self-Localization by Fluorescent Lights
289