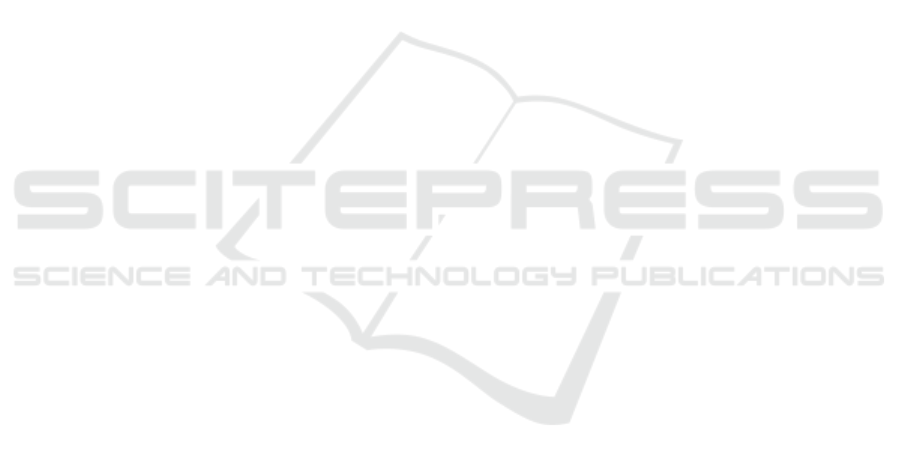
6 CONCLUSION
Understanding the landscape of research institutions
is a challenging task for researchers. Current solu-
tions do not fulfill the requirements imposed by the
resulting exploratory search process. Through several
interviews we derived key requirements for a possi-
ble solution that we prototypically sketched out as a
minimal web application serving as proof of concept.
Our proposed application is able to provide extensive
information for a rather vague exploratory objective.
Further, it enables users to gain insights into the de-
sired search space by semantically linking related en-
tities and aggregating relevant data points into insight-
ful analytical views. However, the approach is limited
by the quality and availability of relevant data and is
restricted to data of one research institution. Since
accumulating large amounts of data and presenting
them in an exhaustive yet concise manner is inher-
ently complex, the degree to which such a task can
be simplified is limited and still requires the user to
invest a certain amount of time and effort in order to
reach the desired goal. That being said, we do believe
that having relevant data semantically linked and eas-
ily accessible within one application helps alleviate
the necessary time and effort investment to achieve
an exhaustive overview of a particular research land-
scape. In future work, we aim to implement the pro-
posed application as a minimum viable product that
we can then systematically evaluate. To further en-
hance the proposed approach, we subsequently plan
to extend the initial implementation based on the user
feedback.
REFERENCES
Athukorala, K., Głowacka, D., Jacucci, G., Oulasvirta, A.,
and Vreeken, J. (2016). Is exploratory search dif-
ferent? a comparison of information search behav-
ior for exploratory and lookup tasks. Journal of the
Association for Information Science and Technology,
67(11):2635–2651.
Brainard, J. (2020). Scientists are drowning in covid-19
papers. can new tools keep them afloat. Science,
13(10):1126.
Dong, Y., Ma, H., Shen, Z., and Wang, K. (2017). A cen-
tury of science: Globalization of scientific collabora-
tions, citations, and innovations. In Proceedings of
the 23rd ACM SIGKDD International Conference on
Knowledge Discovery and Data Mining, KDD ’17,
page 1437–1446, New York, NY, USA. Association
for Computing Machinery.
Grether, L. and Witschel, H. F. (2022). Studying inter-
action patterns for knowledge graph exploration. In
Proceedings of the 14th International Joint Confer-
ence on Knowledge Discovery, Knowledge Engineer-
ing and Knowledge Management - KDIR, pages 257–
264. INSTICC, SciTePress.
Hearst, M. A. and Stoica, E. (2009). NLP support for
faceted navigation in scholarly collection. In Pro-
ceedings of the 2009 Workshop on Text and Citation
Analysis for Scholarly Digital Libraries (NLPIR4DL),
pages 62–70, Suntec City, Singapore. Association for
Computational Linguistics.
Jaradeh, M. Y., Oelen, A., Farfar, K. E., Prinz, M., D’Souza,
J., Kismih
´
ok, G., Stocker, M., and Auer, S. (2019).
Open research knowledge graph: Next generation in-
frastructure for semantic scholarly knowledge. In
Proceedings of the 10th International Conference on
Knowledge Capture, K-CAP ’19, page 243–246, New
York, NY, USA. Association for Computing Machin-
ery.
Palagi, E., Gandon, F., Giboin, A., and Troncy, R. (2017).
A survey of definitions and models of exploratory
search. In Proceedings of the 2017 ACM Workshop
on Exploratory Search and Interactive Data Analyt-
ics, ESIDA ’17, page 3–8, New York, NY, USA. As-
sociation for Computing Machinery.
Schneider, P., Schopf, T., Vladika, J., Galkin, M., Simperl,
E., and Matthes, F. (2022). A decade of knowledge
graphs in natural language processing: A survey. In
Proceedings of the 2nd Conference of the Asia-Pacific
Chapter of the Association for Computational Lin-
guistics and the 12th International Joint Conference
on Natural Language Processing (Volume 1: Long
Papers), pages 601–614, Online only. Association for
Computational Linguistics.
Shen, Z., Ma, H., and Wang, K. (2018). A web-scale system
for scientific knowledge exploration. In Proceedings
of ACL 2018, System Demonstrations, pages 87–92,
Melbourne, Australia. Association for Computational
Linguistics.
Sinha, A., Shen, Z., Song, Y., Ma, H., Eide, D., Hsu, B.-
J. P., and Wang, K. (2015). An overview of microsoft
academic service (mas) and applications. In Proceed-
ings of the 24th International Conference on World
Wide Web, WWW ’15 Companion, page 243–246,
New York, NY, USA. Association for Computing Ma-
chinery.
Toney, A. and Dunham, J. (2022). Multi-label classifica-
tion of scientific research documents across domains
and languages. In Proceedings of the Third Workshop
on Scholarly Document Processing, pages 105–114,
Gyeongju, Republic of Korea. Association for Com-
putational Linguistics.
Wang, K., Shen, Z., Huang, C., Wu, C.-H., Dong, Y.,
and Kanakia, A. (2020). Microsoft Academic Graph:
When experts are not enough. Quantitative Science
Studies, 1(1):396–413.
White, R. W. and Roth, R. A. (2009). Exploratory Search:
Beyond the Query-Response Paradigm, pages 1–98.
Springer International Publishing, Cham.
KMIS 2023 - 15th International Conference on Knowledge Management and Information Systems
270