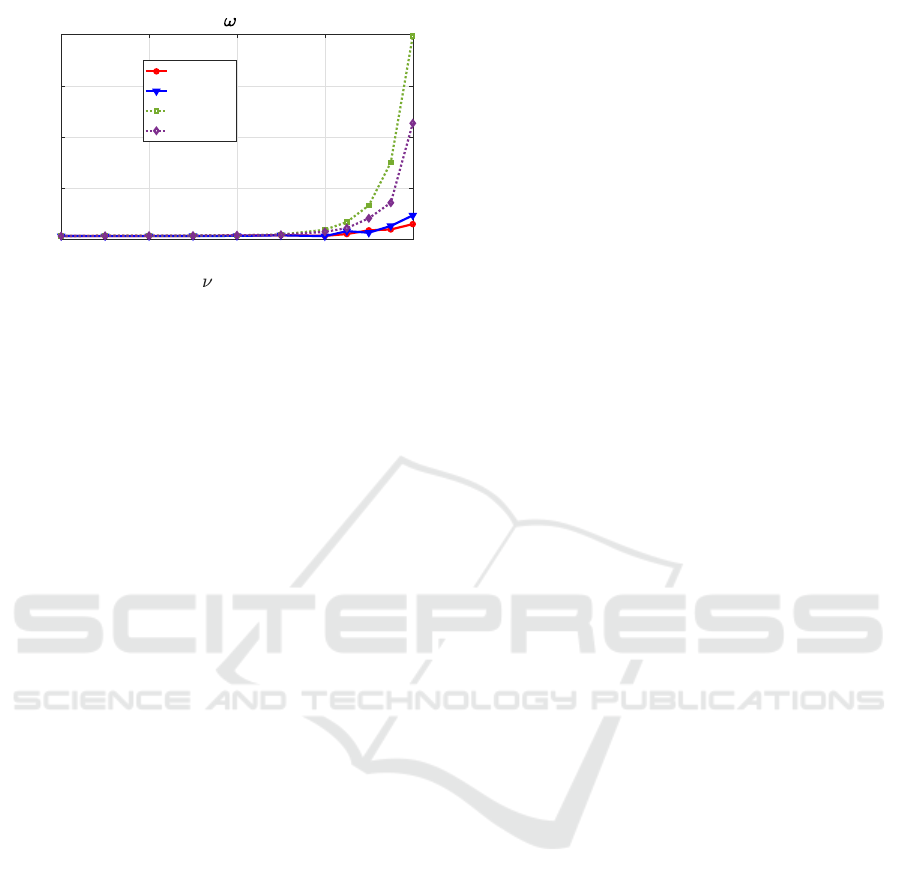
-4 -3 -2 -1 0
[log
10
]
0.48
0.5
0.52
0.54
0.56
STD, = 10
-4
LSUCZ 1
LSUCZ 2
LSUO
LSUP
Figure 3: STD of state estimates for ω = 10
−4
∗ 1
ℓ
x
and
various ν, ν = 10
−K
∗ 1
ℓ
y
, K ∈ [0;4].
ible compared to the previous LSUO and LSUP vari-
ants. It outperforms them from the point of view esti-
mation errors for higher observation noises.
The further research will focus on the more
detailed analysis including the posterior vol-
umes and using the the proposed LSUCZ fil-
ter in the task of a Bayesian transfer learning
schema (Kukli
ˇ
sov
´
a Pavelkov
´
a et al., 2022).
ACKNOWLEDGEMENTS
This research has been supported by GA
ˇ
CR grant 23-
04676J.
REFERENCES
Althoff, M. and Rath, J. J. (2021). Comparison of guaran-
teed state estimators for linear time-invariant systems.
Automatica, 130:109662.
Combastel, C. (2015). Zonotopes and Kalman observers:
Gain optimality under distinct uncertainty paradigms
and robust convergence. Automatica, 55:265 – 273.
Combastel, C. (2016). An extended zonotopic and Gaussian
Kalman filter merging set-membership and stochastic
paradigms: Toward non-linear filtering and fault de-
tection. Annual Reviews in Control, 42:232–243.
Ge, X., Han, Q., and Wang, Z. (2019). A dynamic
event-triggered transmission scheme for distributed
set-membership estimation over wireless sensor net-
works. IEEE Transactions on Cybernetics, 49(1):171–
183.
Jirsa, L., Pavelkov
´
a, L. K., and Quinn, A. (2019). Bayesian
filtering for states uniformly distributed on a parallelo-
topic support. In 2019 IEEE International Symposium
on Signal Processing and Information Technology (IS-
SPIT), pages 1–6. IEEE.
K
´
arn
´
y, M., B
¨
ohm, J., Guy, T. V., Jirsa, L., Nagy, I., Ne-
doma, P., and Tesa
ˇ
r, L. (2006). Optimized Bayesian
Dynamic Advising: Theory and Algorithms. Springer,
London.
Kotz, S. and Dorp, J. R. (2004). Beyond beta: Other con-
tinuous families of distributions with bounded support
and applications. World Scientific.
Kukli
ˇ
sov
´
a Pavelkov
´
a, L., Jirsa, L., and Quinn, A. (2022).
Fully probabilistic design for knowledge fusion be-
tween Bayesian filters under uniform disturbances.
Knowledge based systems, 238:107879.
Pan, Z., Luan, X., and Liu, F. (2022). Set-membership state
and parameter estimation for discrete time-varying
systems based on the constrained zonotope. Interna-
tional journal of control.
Pavelkov
´
a, L. and Jirsa, L. (2018). Approximate recursive
Bayesian estimation of state space model with uni-
form noise. In Proceedings of the 15th ICINCO con-
ference, pages 388–394.
Raghuraman, V. and Koeln, J. P. (2022). Set operations and
order reductions for constrained zonotopes. Automat-
ica, 139:110204.
Rego, B. S., Raffo, G. V., Scott, J. K., and Raimondo, D. M.
(2020a). Guaranteed methods based on constrained
zonotopes for set-valued state estimation of nonlinear
discrete-time systems. Automatica, 111:108614.
Rego, B. S., Raimondo, D. M., and Raffo, V, G. (2020b).
Set-based state estimation and fault diagnosis of lin-
ear discrete-time descriptor systems using constrained
zonotopes. IFAC PapersOnLine, 53(2):4291–4296.
Samada, S. E., Puig, V., and Nejjari, F. (2023). Zonotopic
recursive least-squares parameter estimation: Appli-
cation to fault detection. International Journal of
Adaptive Control and Signal Processing, 37(4):993 –
1014.
S
¨
arkk
¨
a, S. (2013). Bayesian Filtering and Smoothing. Cam-
bridge University Press.
Scott, J. K., Raimondo, D. M., Marseglia, G. R., and Braatz,
R. D. (2016). Constrained zonotopes: A new tool for
set-based estimation and fault detection. Automatica,
69:126–136.
Shao, X., Huang, B., and Lee, J. M. (2010). Constrained
Bayesian state estimation–a comparative study and a
new particle filter based approach. Journal of Process
Control, 20(2):143–157.
Sharma, U., Thangavel, S., Mukkula, A. R. G., and Paulen,
R. (2018). Effective recursive parallelotopic bounding
for robust output-feedback control. IFAC Paperson-
line, 51(15):1032–1037.
Simon, D. and Simon, D. L. (2010). Constrained Kalman
filtering via density function truncation for turbofan
engine health estimation. International Journal of
Systems Science, 41:159–171.
ICINCO 2023 - 20th International Conference on Informatics in Control, Automation and Robotics
194