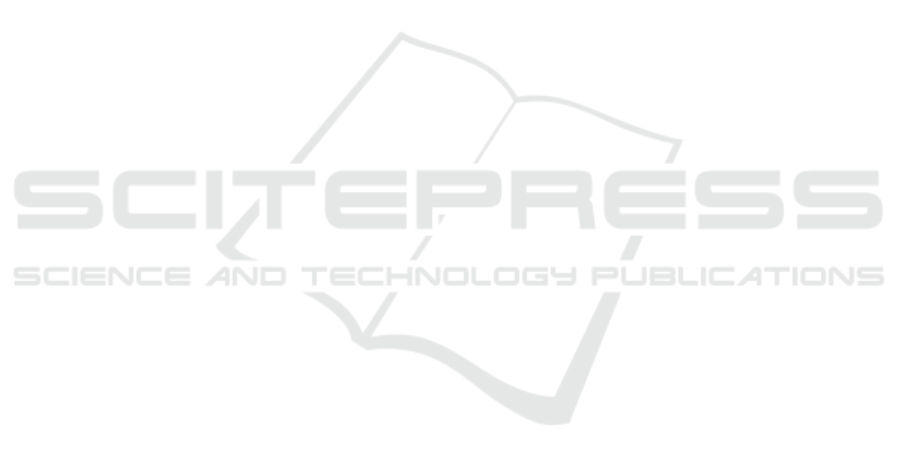
Italian Ministry of University and Research (MUR) in
the framework of the FoReLab and CrossLab projects
(Departments of Excellence).
REFERENCES
(2021). Proposal for a REGULATION OF THE EU-
ROPEAN PARLIAMENT AND OF THE COUN-
CIL LAYING DOWN HARMONISED RULES ON
ARTIFICIAL INTELLIGENCE (ARTIFICIAL IN-
TELLIGENCE ACT) AND AMENDING CERTAIN
UNION LEGISLATIVE ACTS. European Commis-
sion. https://eur-lex.europa.eu/legal-content/EN/TX
T/?uri=CELEX:52021PC0206.
Alcal
´
a-Fdez, J., Fern
´
andez, A., Luengo, J., Derrac, J.,
Garc
´
ıa, S., S
´
anchez, L., and Herrera, F. (2011). Keel
data-mining software tool: data set repository, integra-
tion of algorithms and experimental analysis frame-
work. Journal of Multiple-Valued Logic & Soft Com-
puting, 17.
Antonelli, M., Ducange, P., and Marcelloni, F. (2011). Ge-
netic training instance selection in multiobjective evo-
lutionary fuzzy systems: A coevolutionary approach.
IEEE Transactions on fuzzy systems, 20(2):276–290.
Barredo Arrieta, A., D
´
ıaz-Rodr
´
ıguez, N., Del Ser, J., Ben-
netot, A., Tabik, S., Barbado, A., Garcia, S., Gil-
Lopez, S., Molina, D., Benjamins, R., Chatila, R., and
Herrera, F. (2020). Explainable Artificial Intelligence
(XAI): Concepts, taxonomies, opportunities and chal-
lenges toward responsible AI. Information Fusion,
58:82–115.
Bechini, A., B
´
arcena, J. L. C., Ducange, P., Marcelloni, F.,
and Renda, A. (2022). Increasing Accuracy and Ex-
plainability in Fuzzy Regression Trees: An Experi-
mental Analysis. In 2022 IEEE International Con-
ference on Fuzzy Systems (FUZZ-IEEE), pages 1–8.
IEEE.
Bertsimas, D. and Dunn, J. (2017). Optimal classification
trees. Machine Learning, 106:1039–1082.
Bertsimas, D. and Dunn, J. (2019). Machine learning un-
der a modern optimization lens. Dynamic Ideas LLC
Charlestown, MA.
Bertsimas, D., Dunn, J., and Wang, Y. (2021). Near-optimal
nonlinear regression trees. Operations Research Let-
ters, 49(2):201–206.
Breiman, L., Friedman, J., Olshen, R., and Stone, C. (1984).
Cart. Classification and regression trees.
Cath, C., Wachter, S., Mittelstadt, B., Taddeo, M., and
Floridi, L. (2018). Artificial intelligence and the ‘good
society’: the us, eu, and uk approach. Science and en-
gineering ethics, 24:505–528.
Chaudhuri, P., Lo, W.-D., Loh, W.-Y., and Yang, C.-C.
(1995). Generalized regression trees. Statistica Sinica,
pages 641–666.
Chen, Y.-l., Wang, T., Wang, B.-s., and Li, Z.-j. (2009).
A survey of fuzzy decision tree classifier. Fuzzy Inf.
Eng., 1(2):149–159.
C
´
ozar, J., Marcelloni, F., G
´
amez, J. A., and de la Ossa, L.
(2018). Building efficient fuzzy regression trees for
large scale and high dimensional problems. Journal
of Big Data, 5(1):1–25.
Dunn, J. W. (2018). Optimal trees for prediction and pre-
scription. PhD thesis, Massachusetts Institute of Tech-
nology.
Fontes, C., Hohma, E., Corrigan, C. C., and L
¨
utge, C.
(2022). Ai-powered public surveillance systems: why
we (might) need them and how we want them. Tech-
nology in Society, 71:102137.
High-Level Expert Group on AI (2019). Ethics guidelines
for trustworthy ai. Report, European Commission,
Brussels.
Leikas, J., Johri, A., Latvanen, M., Wessberg, N., and
Hahto, A. (2022). Governing ethical ai transforma-
tion: A case study of auroraai. Frontiers in Artificial
Intelligence, 5:13.
Quinlan, J. R. (1992). Learning with continuous classes.
In 5th Australian joint conference on artificial intelli-
gence, volume 92, pages 343–348. World Scientific.
Renda, A., Ducange, P., Gallo, G., and Marcelloni, F.
(2021). XAI Models for Quality of Experience Pre-
diction in Wireless Networks. In 2021 IEEE Inter-
national Conference on Fuzzy Systems (FUZZ-IEEE),
pages 1–6. IEEE.
Segatori, A., Marcelloni, F., and Pedrycz, W. (2018). On
distributed fuzzy decision trees for big data. IEEE
Transactions on Fuzzy Systems, 26(1):174–192.
Su
´
arez, A. and Lutsko, J. F. (1999). Globally optimal fuzzy
decision trees for classification and regression. IEEE
Transactions on Pattern Analysis and Machine Intel-
ligence, 21(12):1297–1311.
Torsten Hothorn, K. H. and Zeileis, A. (2006). Unbi-
ased recursive partitioning: A conditional inference
framework. Journal of Computational and Graphical
Statistics, 15(3):651–674.
FCTA 2023 - 15th International Conference on Fuzzy Computation Theory and Applications
384