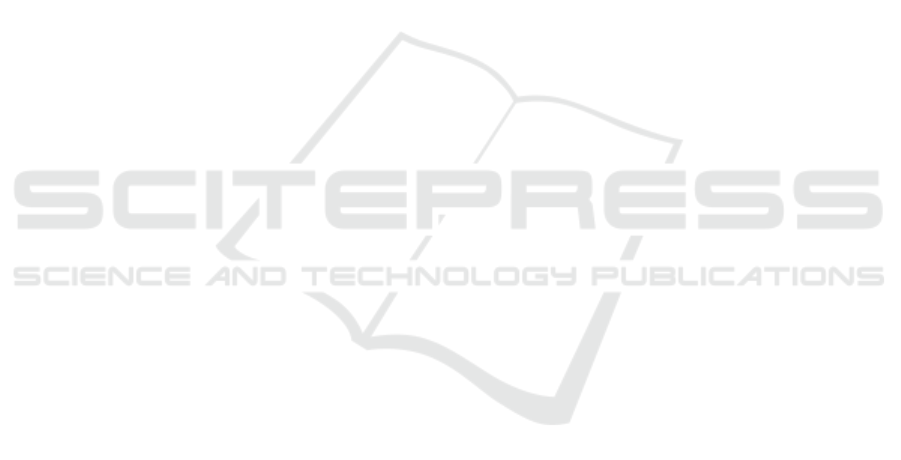
Saif, A. (2022). Financial fraud detection based on ma-
chine learning a systematic literature review. Applied
Sciences, 12(19):9637.
Aslam, F., Hunjra, A. I., Ftiti, Z., Louhichi, W., and Shams,
T. (2022). Insurance fraud detection: Evidence from
artificial intelligence and machine learning. Research
in International Business and Finance, 62:101744.
Badaro, G. and Papotti, P. (2022). Transformers for tab-
ular data representation: a tutorial on models and
applications. Proceedings of the VLDB Endowment,
15(12):3746–3749.
Borisov, V., Broelemann, K., Kasneci, E., and Kasneci, G.
(2023). Deeptlf: robust deep neural networks for het-
erogeneous tabular data. International Journal of Data
Science and Analytics, 16(1):85–100.
Borisov, V., Seßler, K., Leemann, T., Pawelczyk, M., and
Kasneci, G. (2022). Language models are realistic tab-
ular data generators. arXiv preprint arXiv:2210.06280.
Dal Pozzolo, A., Boracchi, G., Caelen, O., Alippi, C., and
Bontempi, G. (2015). Credit card fraud detection and
concept-drift adaptation with delayed supervised in-
formation. In 2015 International Joint Conference on
Neural Networks (IJCNN), pages 1–8.
Devlin, J., Chang, M.-W., Lee, K., and Toutanova, K. (2019).
BERT: Pre-training of deep bidirectional transformers
for language understanding. In Proceedings of the
2019 Conference of the North American Chapter of
the Association for Computational Linguistics: Human
Language Technologies, Volume 1 (Long and Short
Papers), pages 4171–4186, Minneapolis, Minnesota.
Association for Computational Linguistics.
Farquad, M. A. H., Ravi, V., and Raju, S. B. (2012). Analyt-
ical crm in banking and finance using svm: a modified
active learning-based rule extraction approach. Inter-
national Journal of Electronic Customer Relationship
Management.
Gama, J.,
ˇ
Zliobait
˙
e, I., Bifet, A., Pechenizkiy, M., and
Bouchachia, A. (2014). A survey on concept drift
adaptation. ACM computing surveys (CSUR), 46(4):1–
37.
Gomes, C., Jin, Z., and Yang, H. (2021). Insurance fraud
detection with unsupervised deep learning. Journal of
Risk and Insurance, 88(3):591–624.
Guo, H., Yuan, S., and Wu, X. (2021). Logbert: Log anomaly
detection via bert. In 2021 International Joint Confer-
ence on Neural Networks (IJCNN), pages 1–8. IEEE.
Gupta, R., Mudigonda, S., and Baruah, P. K. (2021). Tgans
with machine learning models in automobile insurance
fraud detection and comparative study with other data
imbalance techniques. International Journal of Recent
Technology and Engineering, 9:236–244.
Hassan, A. K. I. and Abraham, A. (2016). Modeling insur-
ance fraud detection using imbalanced data classifica-
tion. In Advances in nature and biologically inspired
computing, pages 117–127. Springer.
Herzig, J., Nowak, P. K., M
¨
uller, T., Piccinno, F., and Eisen-
schlos, J. (2020). TaPas: Weakly supervised table
parsing via pre-training. In Proceedings of the 58th
Annual Meeting of the Association for Computational
Linguistics, pages 4320–4333, Online. Association for
Computational Linguistics.
Itri, B., Mohamed, Y., Mohammed, Q., and Omar, B.
(2019a). Performance comparative study of machine
learning algorithms for automobile insurance fraud de-
tection. In 2019 Third International Conference on
Intelligent Computing in Data Sciences (ICDS), pages
1–4. IEEE.
Itri, B., Mohamed, Y., Mohammed, Q., and Omar, B.
(2019b). Performance comparative study of machine
learning algorithms for automobile insurance fraud de-
tection. In 2019 Third International Conference on
Intelligent Computing in Data Sciences (ICDS), page
1–4.
Jiao, Q. and Zhang, S. (2021). A brief survey of word
embedding and its recent development. In 2021 IEEE
5th Advanced Information Technology, Electronic and
Automation Control Conference (IAEAC), volume 5,
page 1697–1701.
Kate, P., Ravi, V., and Gangwar, A. (2023). Fingan:
Chaotic generative adversarial network for analyti-
cal customer relationship management in banking
and insurance. Neural Computing and Applications,
35(8):6015–6028.
Kirlidog, M. and Asuk, C. (2012). A fraud detection ap-
proach with data mining in health insurance. Procedia-
Social and Behavioral Sciences, 62:989–994.
Krawczyk, B. and Wo
´
zniak, M. (2015). One-class clas-
sifiers with incremental learning and forgetting for
data streams with concept drift. Soft Computing,
19(12):3387–3400.
Li, X., Sun, X., Meng, Y., Liang, J., Wu, F., and Li, J. (2019).
Dice loss for data-imbalanced nlp tasks. arXiv preprint
arXiv:1911.02855.
Majhi, S. K., Bhatachharya, S., Pradhan, R., and Biswal, S.
(2019). Fuzzy clustering using salp swarm algorithm
for automobile insurance fraud detection. Journal of
Intelligent & Fuzzy Systems, 36(3):2333–2344.
Nian, K., Zhang, H., Tayal, A., Coleman, T., and Li, Y.
(2016). Auto insurance fraud detection using unsuper-
vised spectral ranking for anomaly. The Journal of
Finance and Data Science, 2(1):58–75.
Peng, Y., Kou, G., Sabatka, A., Chen, Z., Khazanchi, D., and
Shi, Y. (2006). Application of clustering methods to
health insurance fraud detection. In 2006 International
Conference on Service Systems and Service Manage-
ment, volume 1, pages 116–120. IEEE.
Phua, C., Alahakoon, D., and Lee, V. (2004). Minority
report in fraud detection: Classification of skewed data.
SIGKDD Explor. Newsl., 6(1):50–59.
Ryman-Tubb, N. F., Krause, P., and Garn, W. (2018). How
artificial intelligence and machine learning research
impacts payment card fraud detection: A survey and
industry benchmark. Engineering Applications of Arti-
ficial Intelligence, 76:130–157.
Sithic, H. L. and Balasubramanian, T. (2013). Survey of
insurance fraud detection using data mining techniques.
arXiv preprint arXiv:1309.0806.
Soufiane, E., EL Baghdadi, S.-E., Berrahou, A., Mesbah, A.,
and Berbia, H. (2022). Automobile insurance claims
An Explorative Guide on How to Detect Forged Car Insurance Claims with Language Models
411