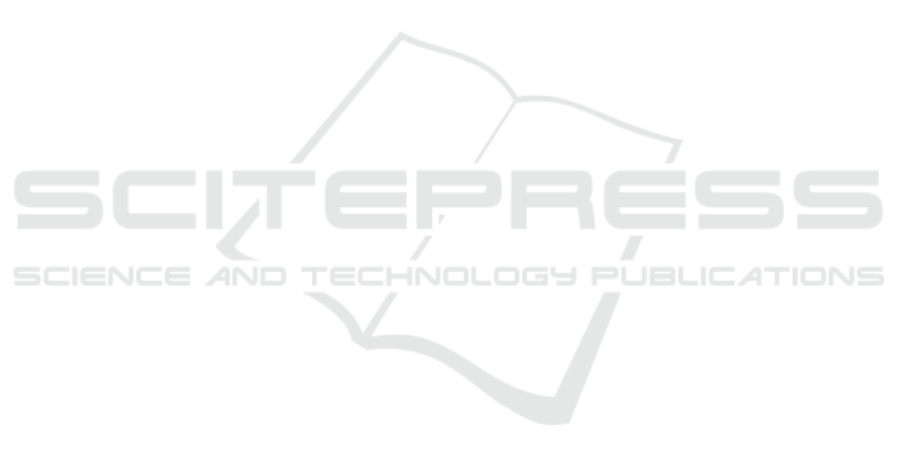
catalogue. In 2021 IEEE 29th international require-
ments engineering conference (RE), pages 197–208.
IEEE.
Doshi-Velez, F. and Kim, B. (2017). Towards a rigorous sci-
ence of interpretable machine learning. arXiv preprint
arXiv:1702.08608.
Duell, J., Fan, X., Burnett, B., Aarts, G., and Zhou, S.-M.
(2021). A comparison of explanations given by ex-
plainable artificial intelligence methods on analysing
electronic health records. In 2021 IEEE EMBS Inter-
national Conference on Biomedical and Health Infor-
matics (BHI), pages 1–4. IEEE.
et. al., O. G. (2023). Six human-centered artificial intel-
ligence grand challenges. International journal of
human-computer interaction, 39(3):391–437.
Goodman, B. and Flaxman, S. (2017). European union reg-
ulations on algorithmic decision-making and a “right
to explanation”. AI magazine, 38(3):50–57.
Gunning, D., Stefik, M., Choi, J., Miller, T., Stumpf, S.,
and Yang, G.-Z. (2019). Xai—explainable artificial
intelligence. Science robotics, 4(37):eaay7120.
Gupta, A., Anpalagan, A., Guan, L., and Khwaja, A. S.
(2021). Deep learning for object detection and scene
perception in self-driving cars: Survey, challenges,
and open issues. Array, 10:100057.
K
¨
ohl, M. A., Baum, K., Langer, M., Oster, D., Speith, T.,
and Bohlender, D. (2019). Explainability as a non-
functional requirement. In 2019 IEEE 27th Inter-
national Requirements Engineering Conference (RE),
pages 363–368. IEEE.
Langer, M., Baum, K., Hartmann, K., Hessel, S., Speith,
T., and Wahl, J. (2021a). Explainability audit-
ing for intelligent systems: a rationale for multi-
disciplinary perspectives. In 2021 IEEE 29th inter-
national requirements engineering conference work-
shops (REW), pages 164–168. IEEE.
Langer, M., Oster, D., Speith, T., Hermanns, H., K
¨
astner,
L., Schmidt, E., Sesing, A., and Baum, K. (2021b).
What do we want from explainable artificial intel-
ligence (xai)?–a stakeholder perspective on xai and
a conceptual model guiding interdisciplinary xai re-
search. Artificial Intelligence, 296:103473.
Longo, L., Goebel, R., Lecue, F., Kieseberg, P., and
Holzinger, A. (2020). Explainable artificial intelli-
gence: Concepts, applications, research challenges
and visions. In International cross-domain conference
for machine learning and knowledge extraction, pages
1–16. Springer.
Miller, T. (2019). Explanation in artificial intelligence: In-
sights from the social sciences. Artificial intelligence,
267:1–38.
Mittelstadt, B., Russell, C., and Wachter, S. (2019). Ex-
plaining explanations in ai. In Proceedings of the con-
ference on fairness, accountability, and transparency,
pages 279–288.
Mondal, M. R. H., Bharati, S., and Podder, P. (2021). Co-
irv2: Optimized inceptionresnetv2 for covid-19 detec-
tion from chest ct images. PloS one, 16(10):e0259179.
Moustakidis, S., Plakias, S., Kokkotis, C., Tsatalas, T.,
and Tsaopoulos, D. (2023). Predicting football team
performance with explainable ai: Leveraging shap to
identify key team-level performance metrics. Future
Internet, 5(5):174.
Nadeem, A., Verwer, S., Moskal, S., and Yang, S. J.
(2021). Alert-driven attack graph generation using s-
pdfa. IEEE Transactions on Dependable and Secure
Computing, 19(2):731–746.
Nadeem, A., Vos, D., Cao, C., Pajola, L., Dieck, S., Baum-
gartner, R., and Verwer, S. (2022). Sok: Explainable
machine learning for computer security applications.
arXiv preprint arXiv:2208.10605.
Nazar, M., Alam, M. M., Yafi, E., and Su’ud, M. M. (2021).
A systematic review of human–computer interaction
and explainable artificial intelligence in healthcare
with artificial intelligence techniques. IEEE Access,
9:153316–153348.
Padilla, L. M., Creem-Regehr, S. H., Hegarty, M., and Ste-
fanucci, J. K. (2018). Decision making with visualiza-
tions: a cognitive framework across disciplines. Cog-
nitive research: principles and implications, 3(1):1–
25.
P
´
aez, A. (2019). The pragmatic turn in explainable artificial
intelligence (xai). Minds and Machines, 29(3):441–
459.
Panigutti, C., Beretta, A., Giannotti, F., and Pedreschi, D.
(2022). Understanding the impact of explanations on
advice-taking: a user study for ai-based clinical deci-
sion support systems. In Proceedings of the 2022 CHI
Conference on Human Factors in Computing Systems,
pages 1–9.
Rathi, K., Somani, P., Koul, A. V., and Manu, K. (2020).
Applications of artificial intelligence in the game of
football: The global perspective. Researchers World,
11(2):18–29.
Russell, S. J. (2010). Artificial intelligence a modern ap-
proach. Pearson Education, Inc.
Ryan Beal, T. J. N. and Ramchurn, S. D. (2019). Artificial
intelligence for team sports: a survey. The Knowledge
Engineering Review, 34:1–40.
Sopan, A., Berninger, M., Mulakaluri, M., and Katakam,
R. (2018). Building a machine learning model for the
soc, by the input from the soc, and analyzing it for the
soc. In 2018 IEEE Symposium on Visualization for
Cyber Security (VizSec), pages 1–8. IEEE.
Van Lent, M., Fisher, W., and Mancuso, M. (2004). An ex-
plainable artificial intelligence system for small-unit
tactical behavior. In Proceedings of the national con-
ference on artificial intelligence, pages 900–907. Cite-
seer.
Vishwarupe, V., Joshi, P. M., Mathias, N., Maheshwari, S.,
Mhaisalkar, S., and Pawar, V. (2022). Explainable ai
and interpretable machine learning: A case study in
perspective. Procedia Computer Science, 204:869–
876.
Viton, F., Elbattah, M., Gu
´
erin, J.-L., and Dequen, G.
(2020). Heatmaps for visual explainability of cnn-
based predictions for multivariate time series with ap-
plication to healthcare. In 2020 IEEE International
Conference on Healthcare Informatics (ICHI), pages
1–8. IEEE.
icSPORTS 2023 - 11th International Conference on Sport Sciences Research and Technology Support
220