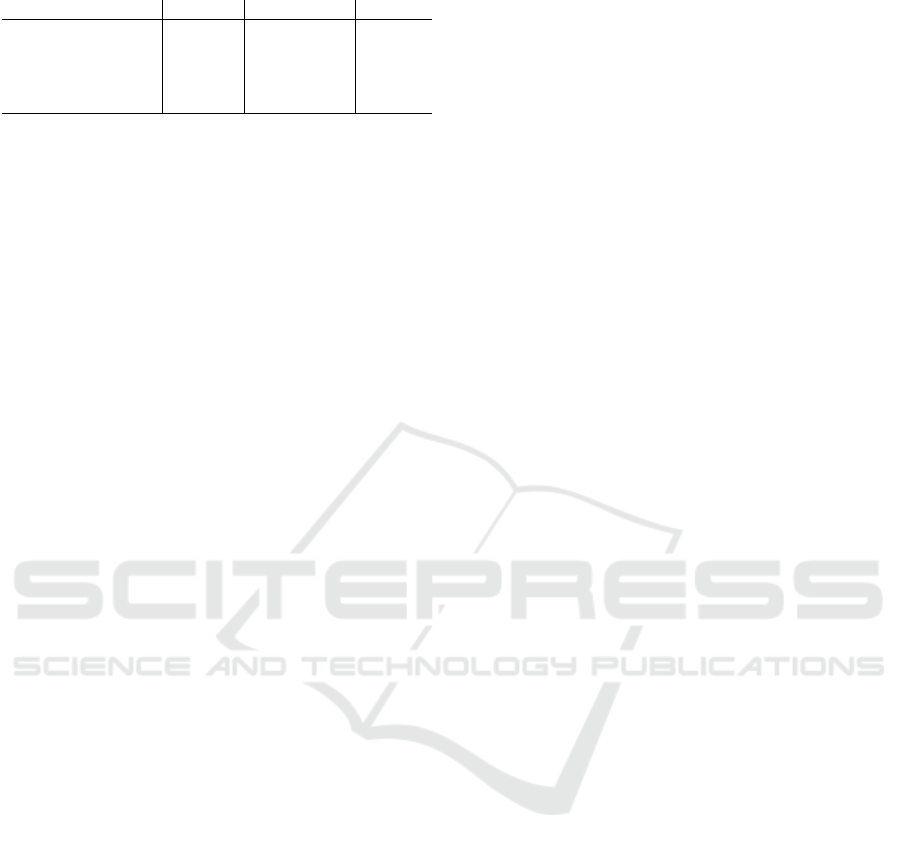
Table 2: Quantity of articles per category.
Category Irrelevant Less Relevant Relevant
Innovation 6317 473 253
Efficiency 3606 353 238
Sustainability 1181 97 59
Industry 4.0 1232 98 53
Artificial Intelligence 184 10 7
showcasing the names of the top 5 most relevant arti-
cles within each category:
8 CONCLUSION
In conclusion, this paper aimed to compare systematic
literature review methods to identify the most efficient
approach for conducting quick and effective literature
reviews. We categorized productivity in the industry
into six principal sectors, namely Innovation, Sustain-
ability, Industry 4.0, Industry 5.0, Efficiency, and Ar-
tificial Intelligence. Subsequently, we conducted sys-
tematic literature reviews for each of these sectors.
To achieve efficient categorization, we employed
four Machine Learning models: Multinomial Naive
Bayes Classification (MNBC), Stochastic Gradient
Descent (SGD), Support Vector Machines (SVM),
and Decision Tree Classifier (DTC). Among these
models, the Decision Tree Classifier (DTC) demon-
strated superior performance with a classification ac-
curacy exceeding 75%.
To identify the most relevant contributions of sci-
entific articles in each research domain or category,
we utilized a relevance classifier with two essential
metrics: the standard deviation of the mean and the Z
score.
As a forward-looking suggestion for further re-
search, we highlight the significance of incorporating
additional data sources and considering the h-index
as an alternative approach for ranking the relevance
of articles. By expanding the scope of data and incor-
porating diverse evaluation metrics, future studies can
enhance the accuracy and depth of systematic litera-
ture reviews, ultimately contributing to more compre-
hensive insights and informed decision-making in the
domain of industrial productivity.
ACKNOWLEDGMENTS
Thanks to the University of Brasilia (UnB) for sup-
porting this research and to the National Industrial
Learning Service (SENAI) of the National Confed-
eration of Industry (CNI) for partially supporting this
research.
REFERENCES
Adesope, O. O., Lavin, T., Thompson, T., and Ungerleider,
C. (2010). A systematic review and meta-analysis of
the cognitive correlates of bilingualism. Review of ed-
ucational research, 80(2):207–245.
Borracini, G. F. (2021). O impacto dos subs
´
ıdios na
evoluc¸
˜
ao da produtividade do setor agr
´
ıcola: e
obtenc¸
˜
ao do t
´
ıtulo de mestre no mestrado profissional
em economia. IDP/FAB.
Chebil, W., Wedyan, M., Alazab, M., Alturki, R., and
Elshaweesh, O. (2023). Improving semantic informa-
tion retrieval using multinomial naive bayes classifier
and bayesian networks. Information, 14(5):272.
Cohen, A. M., Hersh, W. R., Peterson, K., and Yen, P.-
Y. (2006). Reducing workload in systematic review
preparation using automated citation classification.
Journal of the American Medical Informatics Associ-
ation, 13(2):206–219.
de Melo, M. K., Faria, A. V. A., Weigang, L., Nery, A. G.,
de Oliveira, F. A. R., Barreiro, I. T., and Celestino, V.
R. R. (2022). Few-shot approach for systematic lit-
erature review classifications. Proceedings of the 18th
International Conference on Web Information Systems
and Technologies WEBIST.
Galv
˜
ao, M. C. B., Pluye, P., and Ricarte, I. L. M.
(2017). M
´
etodos de pesquisa mistos e revis
˜
oes de
literatura mistas: conceitos, construc¸
˜
ao e crit
´
erios de
avaliac¸
˜
ao. InCID: Revista de Ci
ˆ
encia da Informac¸
˜
ao
e Documentac¸
˜
ao, 8(2):4–24.
Howick, J. (2011). The oxford 2011 levels of evidence.
http://www. cebm. net/index. aspx? o= 5653.
Leong, W. C., Bahadori, A., Zhang, J., and Ahmad, Z.
(2021). Prediction of water quality index (wqi) us-
ing support vector machine (svm) and least square-
support vector machine (ls-svm). International Jour-
nal of River Basin Management, 19(2):149–156.
Mundial, B. (2018). Emprego e crescimento: a agenda da
produtividade. Bras
´
ılia: Grupo Banco Mundial.
Nakkiran, P., Kaplun, G., Kalimeris, D., Yang, T., Edelman,
B. L., Zhang, F., and Barak, B. (2019). Sgd on neu-
ral networks learns functions of increasing complex-
ity. arXiv preprint arXiv:1905.11604.
Santos, M. R. d., Pandullo, R. P., and Souza, E. C. d. (2019).
Evoluc¸
˜
ao da produtividade total dos fatores e da pro-
dutividade da m
˜
ao de obra da ind
´
ustria brasileira: uma
an
´
alise setorial e comparativa. Universidade Cat
´
olica
de Bras
´
ılia.
Torezani, T. A. (2018). Evoluc¸
˜
ao da produtividade
brasileira: mudanc¸a estrutural e din
ˆ
amica tecnol
´
ogica
em uma abordagem multissetorial. Universidade Fed-
eral do Rio Grande do Sul.
Wang, F., Wang, Q., Nie, F., Li, Z., Yu, W., and Ren, F.
(2020). A linear multivariate binary decision tree clas-
sifier based on k-means splitting. Pattern Recognition,
107:107521.
Weigang, L., Enamoto, L. M., Li, D. L., and Rocha Filho,
G. P. (2022). New directions for artificial intelli-
gence: human, machine, biological, and quantum in-
telligence. Frontiers of Information Technology &
Electronic Engineering, 23(6):984–990.
WEBIST 2023 - 19th International Conference on Web Information Systems and Technologies
478