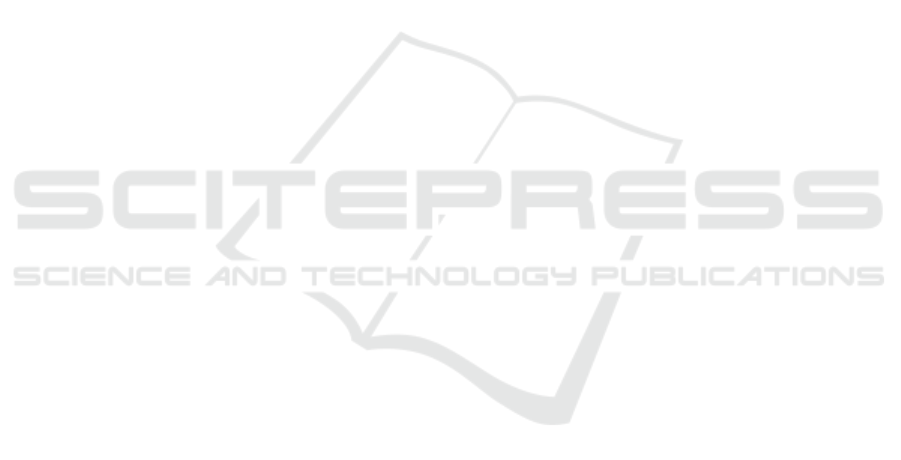
ther to exemplify such discrimination in society, as
any type of disregard to self-determination factors
such as race, color, gender and etc., defined in the
International Covenant on Civil and Political Rights
(United Nations (General Assembly), 1966), there is
the purpose of this study, wage discrimination. Wage
discrimination can be defined, based on the Interna-
tional Covenant, as a structural reduction on some-
one’s salary “just because” of factors they do not have
control over, e.g., race and gender, or they have the
right of choice and not being discriminated because of
it, e.g., religious or political opinion. This type of dis-
crimination can be observed in many different studies
of many different purposes, like bias against handi-
capped workers, presented in (Johnson and Lambri-
nos, 1985), discriminatory behavior by employers us-
ing Oaxaca-Blinder estimator in (Neumark, 1988),
bias analysis comparing gender and color factors us-
ing a linear regression function in (Blinder, 1973) and
comparing salary differential based on gender in pub-
lic and private sectors in Brazil, presented in (Passos
and Machado, 2022). But, as well as social factors
being analyzed in wage structures, there is also purely
(or mostly) objective factors being used in salary pre-
diction automated decision-making systems, such as
(Viroonluecha and Kaewkiriya, 2018), (Lothe et al.,
2021) and (Kuo et al., 2021). Even then, these sim-
ilar studies either do not analyse the full scope of
wage discrimination (evaluating different AI models
for predictions) or do not approach a detailed analysis
of both objective and social feature’s impact in wages.
With this in mind, it is important to also notice this
discrimination and bias will likely be, at some point,
stored in databases. Given the importance of data to
create machine learning models, and the possibility of
this biased data being used as a source of learning by
the model, a problem starts: the use of Big Data for
AI models. Since the model learns searching patterns
in data, social features in Big Data being used, spe-
cially for financial problems, if not well handled, may
perpetuate inequality in a workplace environment, as
explained in (Kim, 2016) and (Favaretto et al., 2019).
Data-driven solution to problems of financial nature,
depending on how it is approached, may have implicit
discrimination if the data available often rely on fea-
ture correlation and not cause-effect, as explained in
(Gillis and Spiess, 2019).
These AI models will use this biased data to get
statistical patterns of, for example, correlation be-
tween gender and salary, will find it, and will repli-
cate it. To analyze the model and prove that it is
not biased, it is necessary to a) show that the meth-
ods for the model assumptions and statistical analy-
sis are not biased and b) show that the data used for
the model training is not biased, according to (Fer-
rer et al., 2021). And based on this, it is possible to
affirm the same, but with the opposite objective: if
a) the model training method is biased or b) the data
used for training is biased, then the model is also bi-
ased. The search for bias in data can be made with a
combination of descriptive statistics, based in (Fisher
and Marshall, 2009), and inferential statistics, based
in (Marshall and Jonker, 2011).
Analyzing the model’s results is important not
only as a part of model tuning, but also to define
its impacts when used: in this case, discrimination.
This step of AI modeling is better described in (Cabr-
era et al., 2023), but, in a more objective descrip-
tion, this is making sense of model results, that is,
understanding what kind of patterns the model repli-
cate, through grouping data and analyzing the most
repeated patterns in the results, for example, this can
be made by getting the model predictions and ana-
lyzing them with multiple descriptive and inferential
statistical approaches, similar to (Blinder, 1973), al-
though this study simply made a descriptive analysis
of the results based on the model’s way of learning.
When analyzing the model’s results, if it has got-
ten to the conclusion of bias being present in the
model and, possibly, in data, it is needed to mitigate
it. To reduce the bias, ultimately, it is necessary to
handle the data used, especially regarding factors in-
cluding sensitive data and Big Data previously dis-
cussed. There is also the possibility of the bias to
be present only in the AI model, but not in data it-
self, being that, in this case, the change of algorithm
would likely be needed. For data bias to be reduced,
it is not as simple as removing social features from
the data in hopes of the bias to disappear, since social
discrimination might still be strongly linked to objec-
tive factors (Kamiran and Calders, 2009) (Pelillo and
Scantamburlo, 2021). In other words, implicit bias,
or “involuntary discrimination”, can, and likely will,
be present. Implicit bias is when, without noticing,
someone ends up discriminating against a given so-
cial group (Brownstein and Zalta, 2019). This bias
can happen based on personal experiences of an indi-
vidual (Tversky and Kahneman, 1974) or a systemic,
and historical, discrimination that makes an individ-
ual be biased without knowing (Payne and Hannay,
2021).
4 METHODOLOGY
A simple description of the methodology used is, as
described in Figure 1: (1) descriptive analysis of the
available data to display distribution patterns; (2) in-
Impacts of Social Factors in Wage Definitions
143