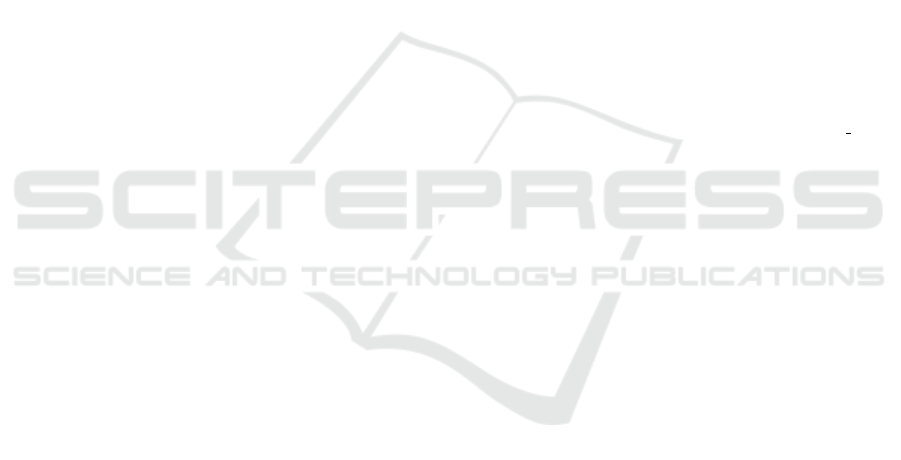
Group), UCA, ENSMR, and ENSIAS. We would like
to express our gratitude to the MANAGEM Group
and its subsidiary CMG for providing us with the
opportunity to conduct research, collect and validate
data on-site, and for being an industrial partner in this
project.
REFERENCES
Ai, M., Xie, Y., Xu, D., Gui, W., and Yang, C. (2018).
Data-driven flotation reagent changing evaluation via
union distribution analysis of bubble size and shape.
The Canadian Journal of Chemical Engineering,
96:2616–2626.
Aldrich, C., Avelar, E., and Liu, X. (2022). Recent advances
in flotation froth image analysis. Minerals Engineer-
ing, 188:107823.
Bendaouia., A., Abdelwahed., E., Qassimi., S., Boussetta.,
A., Benzakour., I., Amar., O., Bourzeix., F., Soulala.,
A., and Hasidi., O. (2023a). Conv-lstm for real
time monitoring of the mineral grades in the flotation
froth. In Proceedings of the 12th International Con-
ference on Data Science, Technology and Applications
- DATA, pages 89–96. INSTICC, SciTePress.
Bendaouia., A., Abdelwahed., E., Qassimi., S., Boussetta.,
A., Hasidi, I. B. O., Amar., O., and Chafiqui., Y.
(2023b). Fourier transform and machine learning for
real-time monitoring of froth flotation in mining in-
dustry. In Proceedings of the 6TH IEEE Conference
on Information and Multimedia Processing. IEEE,
Iieee xplore digital library.
Bendaouia, A., Abdelwahed, E. H., Qassimi, S., Boussetta,
A., Benhayoun, A., Benzakour, I., Amar, O., Zen-
nayi, Y., Bourzeix, F., Ba
¨
ına, K., Ba
¨
ına, S., Khalil, A.,
Cherkaoui, M., and Hasidi, O. (2022). Digital Trans-
formation of the Flotation Monitoring Towards an On-
line Analyzer. In Hamlich, M., Bellatreche, L., Sia-
dat, A., and Ventura, S., editors, Smart Applications
and Data Analysis, Communications in Computer and
Information Science, pages 325–338, Cham. Springer
International Publishing.
Cao, W., Wang, R., Fan, M., Fu, X., Wang, H., and
Wang, Y. (2022). A new froth image classification
method based on the MRMR-SSGMM hybrid model
for recognition of reagent dosage condition in the coal
flotation process. Appl. Intell.
Hasidi, O., Abdelwahed, E. H., Qazdar, A., Boulaamail,
A., Krafi, M., Benzakour, I., Bourzeix, F., Ba
¨
ına, S.,
Ba
¨
ına, K., Cherkaoui, M., and Bendaouia, A. (2022).
Digital Twins-Based Smart Monitoring and Optimi-
sation of Mineral Processing Industry. In Hamlich,
M., Bellatreche, L., Siadat, A., and Ventura, S., ed-
itors, Smart Applications and Data Analysis, pages
411–424, Cham. Springer International Publishing.
Horn, Z. C., Auret, L., McCoy, J. T., Aldrich, C., and
Herbst, B. M. (2017). Performance of Convolu-
tional Neural Networks for Feature Extraction in Froth
Flotation Sensing. IFAC-PapersOnLine, 50(2):13–18.
Jovanovi
´
c, I., Miljanovi
´
c, I., and Jovanovi
´
c, T. (2015). Soft
computing-based modeling of flotation processes – A
review. Minerals Engineering, 84:34–63.
Kaartinen, J., H
¨
at
¨
onen, J., Hy
¨
otyniemi, H., and Miettunen,
J. (2006). Machine-vision-based control of zinc flota-
tion—A case study. Control Engineering Practice, 14.
Liu, J., Gao, Q., Tang, Z., Xie, Y., Gui, W., Ma, T., and
Niyoyita, J. P. (2020). Online Monitoring of Flotation
Froth Bubble-Size Distributions via Multiscale De-
blurring and Multistage Jumping Feature-Fused Full
Convolutional Networks. IEEE Transactions on In-
strumentation and Measurement, 69:9618–9633.
Massinaei, M., Jahedsaravani, A., Taheri, E., and
Khalilpour, J. (2019). Machine vision based moni-
toring and analysis of a coal column flotation circuit.
Powder Technology, 343:330–341.
Rajapakse, N., Zargar, M., Sen, T., and Khiadani, M.
(2022). Effects of influent physicochemical character-
istics on air dissolution, bubble size and rise velocity
in dissolved air flotation: A review. Separation and
Purification Technology, 289.
Tang, M., Zhou, C., Zhang, N., Liu, C., Pan, J., and
Cao, S. (2021). Prediction of the Ash Content of
Flotation Concentrate Based on Froth Image Process-
ing and BP Neural Network Modeling. Interna-
tional Journal of Coal Preparation and Utilization,
41:191–202. Publisher: Taylor & Francis eprint:
https://doi.org/10.1080/19392699.2018.1458716.
Wen, Z., Zhou, C., Pan, J., Nie, T., Zhou, C., and Lu, Z.
(2021). Deep learning-based ash content prediction of
coal flotation concentrate using convolutional neural
network. Minerals Engineering, 174:107251.
Zarie, M., Jahedsaravani, A., and Massinaei, M. (2020).
Flotation froth image classification using convolu-
tional neural networks. Minerals Engineering.
Zhang, D. and Gao, X. (2021). Soft sensor of flotation froth
grade classification based on hybrid deep neural net-
work. International Journal of Production Research.
Zhang, L. and Xu, D. (2020). Flotation bubble size dis-
tribution detection based on semantic segmentation.
IFAC-PapersOnLine, 53.
KDIR 2023 - 15th International Conference on Knowledge Discovery and Information Retrieval
436