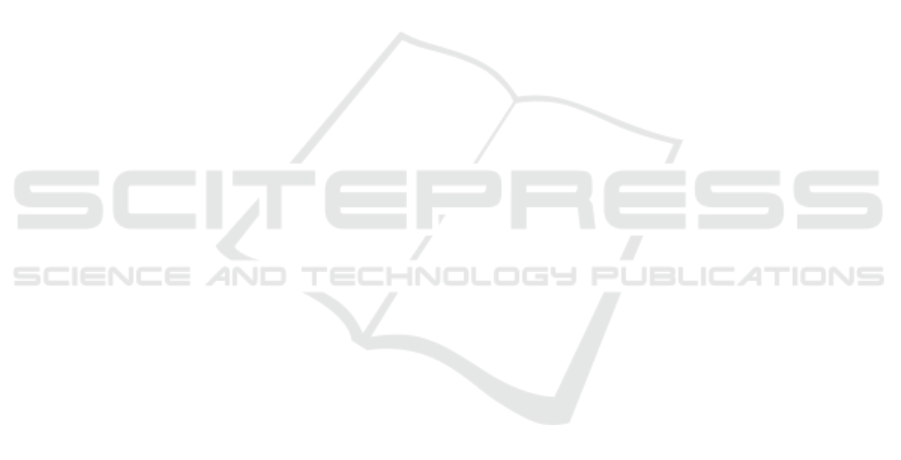
Geurts, P., Ernst, D., and Wehenkel, L. (2006). Extremely
randomized trees. Machine Learning, 63:3–42.
Ho, T. K. (1995). Random decision forests. In Proceedings
of 3rd International Conference on Document Analy-
sis and Recognition, volume 1, pages 278–282 vol.1.
Holland, P. W. and Welsch, R. E. (2007). Robust regression
using iteratively reweighted least-squares. Taylor &
Francis.
Huber, P. and Ronchetti, E. (2011). Robust Statistics. Wiley
Series in Probability and Statistics. Wiley.
Janusz, A., Jamiołkowski, A., and Okulewicz, M. (2022).
Predicting the costs of forwarding contracts: Analysis
of data mining competition results. In 2022 17th Con-
ference on Computer Science and Intelligence Systems
(FedCSIS), pages 399–402.
Lewis, C. (1982). Industrial and business forecasting meth-
ods. London: Butterworths.
Li, D., Ge, Q., Zhang, P., Xing, Y., Yang, Z., and Nai, W.
(2020). Ridge regression with high order truncated
gradient descent method. In 12th International Con-
ference on Intelligent Human-Machine Systems and
Cybernetics, volume 1, pages 252–255.
Pioro
´
nski, S. and G
´
orecki, T. (2022). Using gradient boost-
ing trees to predict the costs of forwarding contracts.
In 2022 17th Conference on Computer Science and In-
telligence Systems (FedCSIS), pages 421–424. IEEE.
Schulz, E., Speekenbrink, M., and Krause, A. (2018). A
tutorial on Gaussian process regression: Modelling,
exploring, and exploiting functions. Journal of Math-
ematical Psychology, 85:1–16.
Seborg, D. E., Mellichamp, D. A., Edgar, T. F., and Doyle,
F. J. (2010). Process dynamics and control. Wiley.
Shinskey, F. G. (2002). Process control: As taught vs as
practiced. Industrial and Engineering Chemistry Re-
search, 41:3745–3750.
Stasi
´
nski, K. (2020). A literature review on dynamic pric-
ing - state of current research and new directions. In
Hernes, M., Wojtkiewicz, K., and Szczerbicki, E., ed-
itors, Advances in Computational Collective Intelli-
gence, pages 465–477, Cham. Springer International
Publishing.
Sutton, C. D. (2005). 11 - classification and regression trees,
bagging, and boosting. In Rao, C., Wegman, E., and
Solka, J., editors, Data Mining and Data Visualiza-
tion, volume 24 of Handbook of Statistics, pages 303–
329. Elsevier.
Tibshirani, R. (1996). Regression shrinkage and selection
via the lasso. Journal of the Royal Statistical Society.
Series B (Methodological), 58(1):267–288.
Tiwari, H. and Kumar, S. (2021). Link prediction in so-
cial networks using histogram based gradient boost-
ing regression tree. In 2021 International Conference
on Smart Generation Computing, Communication and
Networking (SMART GENCON), pages 1–5.
Tropp, J. (2004). Greed is good: algorithmic results for
sparse approximation. IEEE Transactions on Infor-
mation Theory, 50(10):2231–2242.
Tsolaki, K., Vafeiadis, T., Nizamis, A., Ioannidis, D., and
Tzovaras, D. (2022). Utilizing machine learning on
freight transportation and logistics applications: A re-
view. ICT Express.
Vu, Q. H., Cen, L., Ruta, D., and Liu, M. (2022). Key fac-
tors to consider when predicting the costs of forward-
ing contracts. In 2022 17th Conference on Computer
Science and Intelligence Systems (FedCSIS), pages
447–450. IEEE.
Wang, H. and Hu, D. (2005). Comparison of SVM and LS-
SVM for regression. In 2005 International conference
on neural networks and brain, volume 1, pages 279–
283. IEEE.
Wang, X., Dang, X., Peng, H., and Zhang, H. (2009). The
Theil-Sen estimators in a multiple l;inear regression
model. accessed on 18 August 2023.
Yamashita, T., Yamashita, K., and Kamimura, R. (2006). A
Stepwise AIC Method for Variable Selection in Linear
Regression. Taylor & Francis.
Yao, Z. and Ruzzo, W. (2006). A regression-based k nearest
neighbor algorithm for gene function prediction from
heterogeneous data. BMC bioinformatics, 7 Suppl
1:S11.
Zou, H. and Hastie, T. (2005). Regularization and vari-
able selection via the elastic net. Journal of the Royal
Statistical Society. Series B (Statistical Methodology),
67(2):301–320.
Study on Cost Estimation of the External Fleet Full Truckload Contracts
323