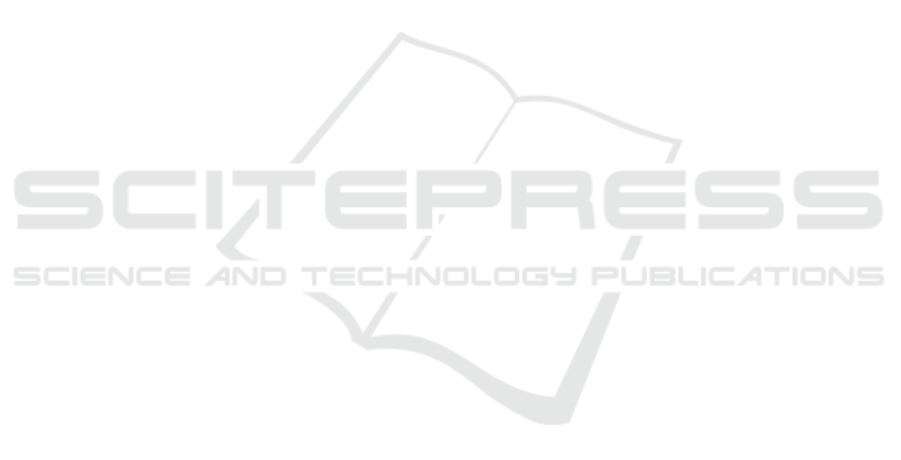
Ben Abdallah, E., Grati, R., and Boukadi, K. (2022). A
machine learning-based approach for smart agricul-
ture via stacking-based ensemble learning and feature
selection methods. In 2022 18th International Con-
ference on Intelligent Environments (IE), pages 1–8.
ISSN: 2472-7571.
Ben Abdallah, E., Grati, R., and Boukadi, K. (2023). To-
wards an explainable irrigation scheduling approach
by predicting soil moisture and evapotranspiration via
multi-target regression. Journal of Ambient Intelli-
gence and Smart Environments, 15:89–110.
Chakraborty, D., Bas¸a
˘
gao
˘
glu, H., and Winterle, J. (2021).
Interpretable vs. noninterpretable machine learning
models for data-driven hydro-climatological pro-
cess modeling. Expert Systems with Applications,
170:114498.
Di Bucchianico, A. (2008). Coefficient of Determination
(R2). John Wiley and Sons, Ltd.
Fereres, E. and Garc
´
ıa-Vila, M. (2018). Irrigation Man-
agement for Efficient Crop Production. In Meyers,
R. A., editor, Encyclopedia of Sustainability Science
and Technology, pages 1–17. Springer, New York, NY.
Ghosal, S., Blystone, D., Singh, A. K., Ganapathysubrama-
nian, B., Singh, A., and Sarkar, S. (2018). An ex-
plainable deep machine vision framework for plant
stress phenotyping. Proceedings of the National
Academy of Sciences of the United States of America,
115(18):4613–4618. Place: Washington Publisher:
Natl Acad Sciences WOS:000431119600044.
Goap, A., Sharma, D., Shukla, A. K., and Rama Krishna,
C. (2018). An IoT based smart irrigation management
system using Machine learning and open source tech-
nologies. Computers and Electronics in Agriculture,
155:41–49.
Goldstein, A., Fink, L., Meitin, A., Bohadana, S., Luten-
berg, O., and Ravid, G. (2018). Applying machine
learning on sensor data for irrigation recommenda-
tions: revealing the agronomist’s tacit knowledge.
Precision Agriculture, 19(3):421–444.
Hochreiter, S. and Schmidhuber, J. (1997). Long Short-
Term Memory. Neural Computation, 9(8):1735–1780.
Jimenez, A.-F., Ortiz, B. V., Bondesan, L., Morata, G., and
Damianidis, D. (2021). Long Short-Term Memory
Neural Network for irrigation management: a case
study from Southern Alabama, USA. Precision Agri-
culture, 22(2):475–492.
Kon
´
e, B. A. T., Grati, R., Bouaziz, B., and Boukadi, K.
(2023). A new long short-term memory based ap-
proach for soil moisture prediction. Journal of Am-
bient Intelligence and Smart Environments, 15:255–
268.
Liakos, K. G., Busato, P., Moshou, D., Pearson, S., and
Bochtis, D. (2018). Machine Learning in Agriculture:
A Review. Sensors, 18(8):2674. Place: Basel Pub-
lisher: Mdpi WOS:000445712400274.
Navarro-Hell
´
ın, H., Mart
´
ınez-del Rincon, J., Domingo-
Miguel, R., Soto-Valles, F., and Torres-S
´
anchez, R.
(2016). A decision support system for managing ir-
rigation in agriculture. Computers and Electronics in
Agriculture, 124:121–131.
Ras, G., Xie, N., Gerven, M. v., and Doran, D. (2022).
Explainable Deep Learning: A Field Guide for the
Uninitiated. Journal of Artificial Intelligence Re-
search, 73:329–396.
Ribeiro, M., Singh, S., and Guestrin, C. (2016). “Why
Should I Trust You?”: Explaining the Predictions of
Any Classifier. In Proceedings of the 2016 Confer-
ence of the North American Chapter of the Associa-
tion for Computational Linguistics: Demonstrations,
pages 97–101, San Diego, California. Association for
Computational Linguistics.
Rima, G., Myriam, A., and Kouloud, B. (2023). Towards a
novel approach for smart agriculture predictability. In
Proceedings of the 18th International Conference on
Software Technologies , ICSOFT’23, pages 96–105,
Italy, Rome. SCITEPRESS - Science and Technology
Publications.
Sammut, C. and Webb, G. I., editors (2010). Encyclopedia
of Machine Learning. Springer US, Boston, MA.
Schmidhuber, J. (2015). Deep learning in neural networks:
An overview. Neural Networks, 61:85–117.
Stanley, S., Antoniou, V., Askquith-Ellis, A., Ball, L., Ben-
nett, E., Blake, J., Boorman, D., Brooks, M., Clarke,
M., Cooper, H., Cowan, N., Cumming, A., Evans, J.,
Farrand, P., Fry, M., Hitt, O., Lord, W., Morrison, R.,
Nash, G., Rylett, D., Scarlett, P., Swain, O., Szczykul-
ska, M., Thornton, J., Trill, E., Warwick, A., and Win-
terbourn, B. (2021). Daily and sub-daily hydrometeo-
rological and soil data (2013-2019) [COSMOS-UK].
Sutskever, I., Vinyals, O., and Le, Q. V. (2014). Sequence to
sequence learning with neural networks. In Proceed-
ings of the 27th International Conference on Neural
Information Processing Systems - Volume 2, NIPS’14,
pages 3104–3112, Montreal, Canada.
Valiant, L. (2014). Probably Approximately Correct. Basic
Books, New York, 1st edition edition.
Xing, L., Cui, N., Guo, L., Du, T., Gong, D., Zhan, C.,
Zhao, L., and Wu, Z. (2022). Estimating daily ref-
erence evapotranspiration using a novel hybrid deep
learning model. Journal of Hydrology, 614:128567.
Yuan, Q., Shen, H., Li, T., Li, Z., Li, S., Jiang, Y., Xu, H.,
Tan, W., Yang, Q., Wang, J., Gao, J., and Zhang, L.
(2020). Deep learning in environmental remote sens-
ing: Achievements and challenges. Remote Sensing of
Environment, 241:111716.
Zhuang, S., Wang, P., Jiang, B., and Li, M. (2020). Learned
features of leaf phenotype to monitor maize water sta-
tus in the fields. Computers and Electronics in Agri-
culture, 172:105347.
ICINCO 2023 - 20th International Conference on Informatics in Control, Automation and Robotics
104