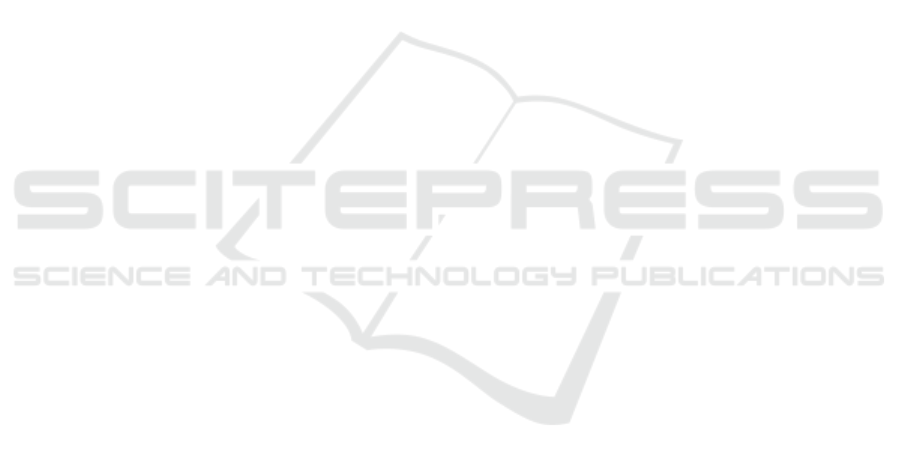
ics, Electronics & Vision (ICIEV) and 2020 4th In-
ternational Conference on Imaging, Vision & Pattern
Recognition (icIVPR), pages 1–6. IEEE.
Abri, S., Abri, R., and C¸ etin, S. (2021). An analytical com-
parison of approaches to real-time object detection to
handle concurrent surveillance video streams. In 2021
6th International Conference on Frontiers of Signal
Processing (ICFSP), pages 43–47. IEEE.
Abri, S., Abri, R., Yarıcı, A., and C¸ etin, S. (2020b). Multi-
thread frame tiling model in concurrent real-time ob-
ject detection for resources optimization in yolov3. In
Proceedings of the 2020 6th International Conference
on Computer and Technology Applications, pages 69–
73.
Bilakeri, S. and Kotegar, K. (2022). Strong base-
line with auto-encoder for scale-invariant person re-
identification.
Bochkovskiy, A., Wang, C.-Y., and Liao, H.-Y. M. (2020).
Yolov4: Optimal speed and accuracy of object detec-
tion. arXiv preprint arXiv:2004.10934.
Chen, T., DIng, S., Xie, J., Yuan, Y., Chen, W., Yang, Y.,
Ren, Z., and Wang, Z. (2019). Abd-net: Attentive
but diverse person re-identification. volume 2019-
October.
Dokmanic, I., Parhizkar, R., Ranieri, J., and Vetterli, M.
(2015). Euclidean distance matrices: essential theory,
algorithms, and applications. IEEE Signal Processing
Magazine, 32(6):12–30.
Hinton, G. E. and Salakhutdinov, R. R. (2006). Reducing
the dimensionality of data with neural networks. sci-
ence, 313(5786):504–507.
Ince, E., Kutuk, S., Abri, R., Abri, S., and Cetin, S.
(2022). A light weight approach for real-time back-
ground subtraction in camera surveillance systems. In
2022 IEEE 5th International Conference on Image
Processing Applications and Systems (IPAS), pages 1–
6. IEEE.
Kingma, D. P. and Ba, J. (2014). Adam: A
method for stochastic optimization. arXiv preprint
arXiv:1412.6980.
Kostinger, M., Hirzer, M., Wohlhart, P., Roth, P. M., and
Bischof, H. (2012). Large scale metric learning from
equivalence constraints.
Lee, K., Lee, K., Lee, H., and Shin, J. (2018). A simple uni-
fied framework for detecting out-of-distribution sam-
ples and adversarial attacks. Advances in neural infor-
mation processing systems, 31.
Li, W., Zhao, R., Xiao, T., and Wang, X. (2014). Deep-
reid: Deep filter pairing neural network for person re-
identification.
Liao, S., Hu, Y., Zhu, X., and Li, S. Z. (2015). Person re-
identification by local maximal occurrence represen-
tation and metric learning. volume 07-12-June-2015.
Maas, A. L., Hannun, A. Y., Ng, A. Y., et al. (2013). Rec-
tifier nonlinearities improve neural network acoustic
models. In Proc. icml, volume 30, page 3. Atlanta,
Georgia, USA.
Mansoub, S. K., Abri, R., and Yarıcı, A. (2019). Concur-
rent real-time object detection on multiple live streams
using optimization cpu and gpu resources in yolov3.
SIGNAL, pages 23–28.
Ming, Z., Zhu, M., Wang, X., Zhu, J., Cheng, J., Gao, C.,
Yang, Y., and Wei, X. (2022). Deep learning-based
person re-identification methods: A survey and out-
look of recent works. Image and Vision Computing,
119:104394.
Ren, J., Ma, X., Xu, C., Zhao, H., and Yi, S. (2021). Ha-
vana: Hierarchical and variation-normalized autoen-
coder for person re-identification.
Ristani, E., Solera, F., Zou, R., Cucchiara, R., and Tomasi,
C. (2016). Performance measures and a data set
for multi-target, multi-camera tracking. In Com-
puter Vision–ECCV 2016 Workshops: Amsterdam,
The Netherlands, October 8-10 and 15-16, 2016, Pro-
ceedings, Part II, pages 17–35. Springer.
Sezavar, A., Farsi, H., and Mohamadzadeh, S. (2023). A
new model for person reidentification using deep cnn
and autoencoders. Iranian (Iranica) Journal of Energy
& Environment, 14(4):314–320.
Tesfaye, Y. T., Zemene, E., Prati, A., Pelillo, M., and Shah,
M. (2017). Multi-target tracking in multiple non-
overlapping cameras using constrained dominant sets.
arXiv preprint arXiv:1706.06196.
Tian, M., Yi, S., Li, H., Li, S., Zhang, X., Shi, J., Yan, J.,
and Wang, X. (2018). Eliminating background-bias
for robust person re-identification. In Proceedings of
the IEEE conference on computer vision and pattern
recognition, pages 5794–5803.
Tibshirani, R. (1996). Regression shrinkage and selection
via the lasso. Journal of the Royal Statistical Society:
Series B (Methodological), 58(1):267–288.
Wu, D., Zheng, S.-J., Zhang, X.-P., Yuan, C.-A., Cheng,
F., Zhao, Y., Lin, Y.-J., Zhao, Z.-Q., Jiang, Y.-L., and
Huang, D.-S. (2019). Deep learning-based methods
for person re-identification: A comprehensive review.
Neurocomputing, 337:354–371.
Xia, B. N., Gong, Y., Zhang, Y., and Poellabauer, C. (2019).
Second-order non-local attention networks for person
re-identification. In Proceedings of the IEEE/CVF
international conference on computer vision, pages
3760–3769.
Zheng, L., Yang, Y., and Hauptmann, A. G. (2016). Per-
son re-identification: Past, present and future. arXiv
preprint arXiv:1610.02984.
Zheng, Z., Yang, X., Yu, Z., Zheng, L., Yang, Y., and Kautz,
J. (2019). Joint discriminative and generative learning
for person re-identification. volume 2019-June.
Similarity Learning for Person Re-Identification Using Deep Auto-Encoder
527