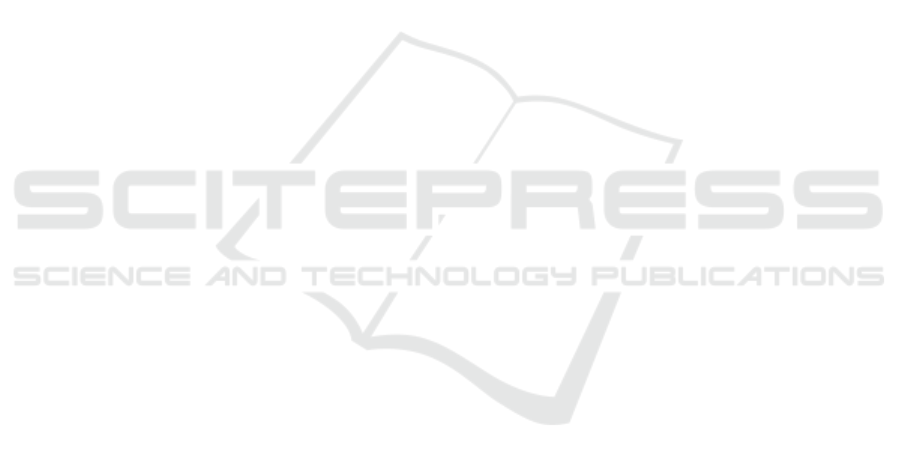
Conference on Information Retrieval, pages 552–566.
Springer.
Boratto, L. and Marras, M. (2021). Advances in bias-aware
recommendation on the web. In Proceedings of the
14th ACM International Conference on Web Search
and Data Mining, pages 1147–1149.
Bronstein, M. M., Bruna, J., LeCun, Y., Szlam, A., and Van-
dergheynst, P. (2017). Geometric deep learning: going
beyond euclidean data. IEEE Signal Processing Mag-
azine, 34(4):18–42.
Bruce, P., Bruce, A., and Gedeck, P. (2020). Practical
statistics for Data Scientists, 2nd edition. O’Reilly
Media, Inc.
Caton, S. and Haas, C. (2020). Fairness in machine learn-
ing: A survey. arXiv preprint arXiv:2010.04053.
Celma, O. (2010). Music Recommendation and Discovery
in the Long Tail. Springer.
Chen, J., Dong, H., Wang, X., Feng, F., Wang, M., and
He, X. (2020). Bias and debias in recommender sys-
tem: A survey and future directions. arXiv preprint
arXiv:2010.03240.
Chen, Z., Xiao, T., and Kuang, K. (2022). Ba-gnn: On
learning bias-aware graph neural network. In 2022
IEEE 38th International Conference on Data Engi-
neering (ICDE), pages 3012–3024. IEEE.
Chia, P. J., Tagliabue, J., Bianchi, F., He, C., and Ko, B.
(2022). Beyond ndcg: behavioral testing of recom-
mender systems with reclist. In Companion Proceed-
ings of the Web Conference 2022, pages 99–104.
Chizari, N., Shoeibi, N., and Moreno-Garc
´
ıa, M. N. (2022).
A comparative analysis of bias amplification in graph
neural network approaches for recommender systems.
Electronics, 11(20):3301.
Chizari, N., Tajfar, K., and Moreno-Garc
´
ıa, M. N. (2023).
Bias assessment approaches for addressing user-
centered fairness in gnn-based recommender systems.
Information, 14(2):131.
Dai, E. and Wang, S. (2021). Say no to the discrimina-
tion: Learning fair graph neural networks with limited
sensitive attribute information. In Proceedings of the
14th ACM International Conference on Web Search
and Data Mining, pages 680–688.
Di Noia, T., Tintarev, N., Fatourou, P., and Schedl, M.
(2022). Recommender systems under european ai reg-
ulations. Communications of the ACM, 65(4):69–73.
Dong, Y., Liu, N., Jalaian, B., and Li, J. (2022a). Edits:
Modeling and mitigating data bias for graph neural
networks. In Proceedings of the ACM Web Confer-
ence 2022, pages 1259–1269.
Dong, Y., Wang, S., Wang, Y., Derr, T., and Li, J. (2022b).
On structural explanation of bias in graph neural net-
works. In Proceedings of the 28th ACM SIGKDD
Conference on Knowledge Discovery and Data Min-
ing, pages 316–326.
Fabbri, F., Croci, M. L., Bonchi, F., and Castillo, C. (2022).
Exposure inequality in people recommender systems:
The long-term effects. In Proceedings of the Inter-
national AAAI Conference on Web and Social Media,
volume 16, pages 194–204.
Fahse, T., Huber, V., and Giffen, B. v. (2021). Managing
bias in machine learning projects. In International
Conference on Wirtschaftsinformatik, pages 94–109.
Springer.
Farnadi, G., Kouki, P., Thompson, S. K., Srinivasan, S., and
Getoor, L. (2018). A fairness-aware hybrid recom-
mender system. arXiv preprint arXiv:1809.09030.
Feldman, M., Friedler, S. A., Moeller, J., Scheidegger, C.,
and Venkatasubramanian, S. (2015). Certifying and
removing disparate impact. In proceedings of the 21th
ACM SIGKDD international conference on knowl-
edge discovery and data mining, pages 259–268.
Floridi, L., Holweg, M., Taddeo, M., Amaya Silva, J.,
M
¨
okander, J., and Wen, Y. (2022). capai-a procedure
for conducting conformity assessment of ai systems in
line with the eu artificial intelligence act. Available at
SSRN 4064091.
Foulds, J. R., Islam, R., Keya, K. N., and Pan, S. (2019).
Differential fairness. UMBC Faculty Collection.
Gao, C., Lei, W., Chen, J., Wang, S., He, X., Li, S., Li, B.,
Zhang, Y., and Jiang, P. (2022a). Cirs: Bursting fil-
ter bubbles by counterfactual interactive recommender
system. arXiv preprint arXiv:2204.01266.
Gao, C., Wang, X., He, X., and Li, Y. (2022b). Graph neural
networks for recommender system. In Proceedings of
the Fifteenth ACM International Conference on Web
Search and Data Mining, pages 1623–1625.
Hardt, M., Price, E., and Srebro, N. (2016). Equality of op-
portunity in supervised learning. Advances in neural
information processing systems, 29.
Islam, R., Keya, K. N., Zeng, Z., Pan, S., and Foulds, J.
(2021). Debiasing career recommendations with neu-
ral fair collaborative filtering. In Proceedings of the
Web Conference 2021, pages 3779–3790.
Khan, Z. Y., Niu, Z., Sandiwarno, S., and Prince, R. (2021).
Deep learning techniques for rating prediction: a sur-
vey of the state-of-the-art. Artificial Intelligence Re-
view, 54(1):95–135.
Kordzadeh, N. and Ghasemaghaei, M. (2022). Algorithmic
bias: review, synthesis, and future research directions.
European Journal of Information Systems, 31(3):388–
409.
Lazovich, T., Belli, L., Gonzales, A., Bower, A., Tantipong-
pipat, U., Lum, K., Huszar, F., and Chowdhury, R.
(2022). Measuring disparate outcomes of content rec-
ommendation algorithms with distributional inequal-
ity metrics. arXiv preprint arXiv:2202.01615.
Li, P., Wang, Y., Zhao, H., Hong, P., and Liu, H. (2021).
On dyadic fairness: Exploring and mitigating bias in
graph connections. In International Conference on
Learning Representations.
Li, X. (2023). Graph Learning in Recommender Systems:
Toward Structures and Causality. PhD thesis, Univer-
sity of Illinois at Chicago.
Lin, S., Wang, J., Zhu, Z., and Caverlee, J. (2022).
Quantifying and mitigating popularity bias in con-
versational recommender systems. arXiv preprint
arXiv:2208.03298.
Loveland, D., Pan, J., Bhathena, A. F., and Lu, Y. (2022).
Fairedit: Preserving fairness in graph neural net-
WEBIST 2023 - 19th International Conference on Web Information Systems and Technologies
186