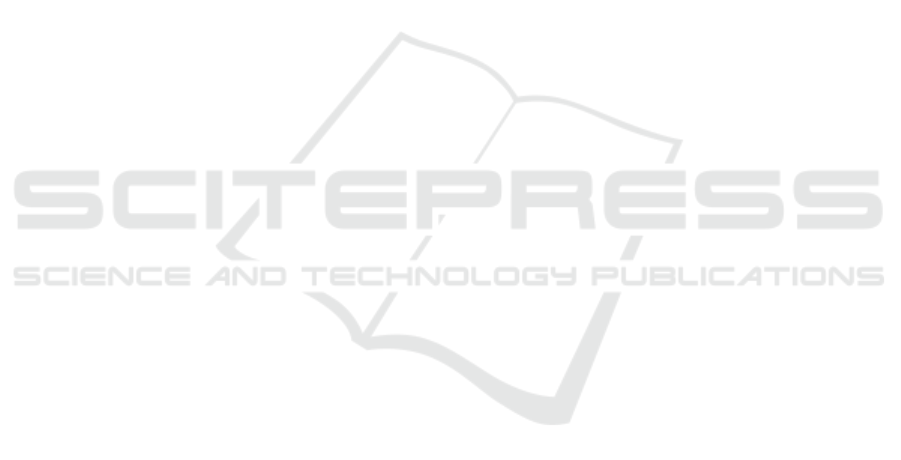
ACKNOWLEDGEMENTS
This work is co-funded by the Research Council of
Norway under the project entitled “Next Generation
3D Machine Vision with Embedded Visual Comput-
ing” with grant number 325748.
REFERENCES
Aalerud, A., Dybedal, J., and Subedi, D. (2020). Reshaping
field of view and resolution with segmented reflectors:
Bridging the gap between rotating and solid-state li-
dars. Sensors, 20(12).
Belgiu, M. and Dr
˘
agut¸, L. (2016). Random forest in remote
sensing: A review of applications and future direc-
tions. ISPRS journal of photogrammetry and remote
sensing, 114:24–31.
Cao, C., Preda, M., and Zaharia, T. (2019). 3d point cloud
compression: A survey. In The 24th International
Conference on 3D Web Technology, pages 1–9.
Cutler, D. R., Edwards Jr., T. C., Beard, K. H., Cutler, A.,
Hess, K. T., Gibson, J., and Lawler, J. J. (2007). Ran-
dom forests for classification in ecology. Ecology,
88(11):2783–2792.
Dai, D., Chen, Z., Bao, P., and Wang, J. (2021). A review of
3d object detection for autonomous driving of electric
vehicles. World Electric Vehicle Journal, 12(3).
Dalal, N., Triggs, B., and Schmid, C. (2006). Human de-
tection using oriented histograms of flow and appear-
ance. volume 3952, pages 428–441.
Goodfellow, I., Bengio, Y., and Courville, A. (2016). Deep
learning. Nature, 521(7553):436–444.
Huang, X., Mei, G., Zhang, J., and Abbas, R. (2021).
A comprehensive survey on point cloud registration.
arXiv preprint arXiv:2103.02690.
Javaheri, A., Brites, C., Pereira, F., and Ascenso, J. (2017).
Subjective and objective quality evaluation of 3d point
cloud denoising algorithms. In 2017 IEEE Interna-
tional Conference on Multimedia & Expo Workshops
(ICMEW), pages 1–6. IEEE.
Li, Y., Bu, R., Sun, M., Wu, W., Di, X., and Chen, B.
(2018a). Pointcnn: Convolution on x-transformed
points. In Bengio, S., Wallach, H., Larochelle, H.,
Grauman, K., Cesa-Bianchi, N., and Garnett, R., edi-
tors, Advances in Neural Information Processing Sys-
tems, volume 31. Curran Associates, Inc.
Li, Y., Bu, R., Sun, M., Wu, W., Di, X., and Chen, B.
(2018b). Pointcnn: Convolution on x-transformed
points. Advances in neural information processing
systems, 31.
Lin, C.-H., Kong, C., and Lucey, S. (2018). Learning ef-
ficient point cloud generation for dense 3d object re-
construction. In proceedings of the AAAI Conference
on Artificial Intelligence, volume 32.
Pedersoli, M., Gonzalez, J., Chakraborty, B., and Vil-
lanueva, J. J. (2008). Enhancing real-time human
detection based on histograms of oriented gradients.
In Computer Recognition Systems 2, pages 739–746.
Springer.
Qi, C. R., Liu, W., Wu, C., Su, H., and Guibas, L. J. (2017a).
Frustum pointnets for 3d object detection from RGB-
D data. CoRR, abs/1711.08488.
Qi, C. R., Su, H., Mo, K., and Guibas, L. J. (2017b). Point-
net: Deep learning on point sets for 3d classification
and segmentation.
Qi, C. R., Su, H., Mo, K., and Guibas, L. J. (2017c). Point-
net: Deep learning on point sets for 3d classification
and segmentation. In Proceedings of the IEEE con-
ference on computer vision and pattern recognition,
pages 652–660.
Qi, C. R., Yi, L., Su, H., and Guibas, L. J. (2017d). Point-
net++: Deep hierarchical feature learning on point sets
in a metric space. In Guyon, I., Luxburg, U. V., Ben-
gio, S., Wallach, H., Fergus, R., Vishwanathan, S., and
Garnett, R., editors, Advances in Neural Information
Processing Systems, volume 30. Curran Associates,
Inc.
Qi, C. R., Yi, L., Su, H., and Guibas, L. J. (2017e). Point-
net++: Deep hierarchical feature learning on point sets
in a metric space. Advances in neural information pro-
cessing systems, 30.
Shi, S., Wang, X., and Li, H. (2019). Pointrcnn: 3d object
proposal generation and detection from point cloud.
In IEEE Conference on Computer Vision and Pattern
Recognition, CVPR 2019, Long Beach, CA, USA, June
16-20, 2019, pages 770–779. Computer Vision Foun-
dation / IEEE.
Simon, M., Milz, S., Amende, K., and Gross, H. (2018).
Complex-yolo: Real-time 3d object detection on point
clouds. CoRR, abs/1803.06199.
Uy, M. A., Pham, Q.-H., Hua, B.-S., Nguyen, T., and
Yeung, S.-K. (2019). Revisiting point cloud clas-
sification: A new benchmark dataset and classifica-
tion model on real-world data. In Proceedings of the
IEEE/CVF international conference on computer vi-
sion, pages 1588–1597.
Yang, G., Huang, X., Hao, Z., Liu, M.-Y., Belongie, S., and
Hariharan, B. (2019a). Pointflow: 3d point cloud gen-
eration with continuous normalizing flows. In Pro-
ceedings of the IEEE/CVF international conference
on computer vision, pages 4541–4550.
Yang, Z., Sun, Y., Liu, S., Shen, X., and Jia, J. (2019b).
STD: sparse-to-dense 3d object detector for point
cloud. CoRR, abs/1907.10471.
ICINCO 2023 - 20th International Conference on Informatics in Control, Automation and Robotics
526