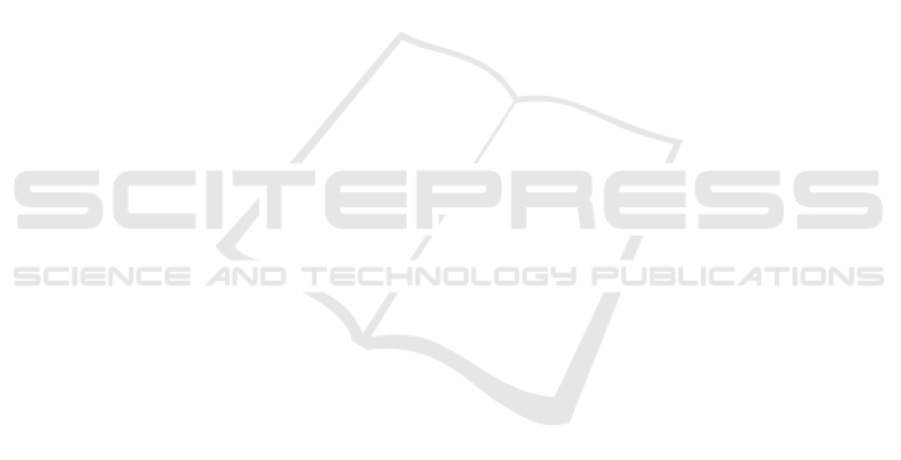
6 CONCLUSION
We presented in this paper an steganalysis attack based
on nine popular machine learning algorithms of the
newly proposed text embedding method of Aziz et al.
(Aziz et al., 2022). We found that the accuracy val-
ues of detecting embedded content using our machine
learning classifiers was surprisingly low (0.553, 0.584
and 0.555), generally resulting in a ROC curve close
to random guess. This initial study indicates that the
new method proposed in (Aziz et al., 2022) so far can
withstand standard machine learning-based attacks.
We plan to continue applying other attack meth-
ods, in particular, other statistical attack methods such
as the 𝜒
2
attack (Pearson, 1900; Plackett, 1983), to
determine if the method can still withstand security
attacks. We also plan to test the embedding capacity
of the (Aziz et al., 2022) to determine its efficiency
in embedding large sized hidden content, and whether
size is a factor in undermining the security of this em-
bedding method.
REFERENCES
Agarwal, M. (2013). Text steganographic approaches: A
comparison. International Journal of Network Secu-
rity and its Applications, 5:91–106.
Aziz, B., Bukhelli, A., Khusainov, R., and Mohasseb, A.
(2022). A novel method for embedding and extracting
secret messages in textual documents based on para-
graph resizing. In 19th International Conference on
Security and Cryptography. SciTePress.
Bayes, T. (1763). Lii. an essay towards solving a problem in
the doctrine of chances. by the late rev. mr. bayes, frs
communicated by mr. price, in a letter to john canton,
amfr s. Philosophical transactions of the Royal Society
of London, 53:370–418.
Cao, H., Naito, T., and Ninomiya, Y. (2008). Approxi-
mate rbf kernel svm and its applications in pedestrian
classification. In The 1st International Workshop on
Machine Learning for Vision-based Motion Analysis-
MLVMA’08.
Chapman, M. and Davida, G. (1997). Hiding the hidden: A
software system for concealing ciphertext as innocu-
ous text. In International Conference on Informa-
tion and Communications Security, pages 335–345.
Springer.
Chinchor, N. (1992). Muc-4 evaluation metrics. In Proceed-
ings of the 4th Conference on Message Understanding,
MUC4 ’92, pages 22–29, Stroudsburg, PA, USA. As-
sociation for Computational Linguistics.
Cortes, C. and Vapnik, V. (1995). Support-vector networks.
Machine learning, 20:273–297.
Hart, M. (1971). Free ebooks - Project Gutenberg.
Kumar, R. and Singh, H. (2020). Recent trends in text
steganography with experimental study. In Handbook
of Computer Networks and Cyber Security, pages 849–
872. Springer.
LeCun, Y., Bottou, L., Bengio, Y., and Haffner, P. (1998).
Gradient-based learning applied to document recogni-
tion. Proceedings of the IEEE, 86(11):2278–2324.
Lockwood, R. and Curran, K. (2017). Text based steganog-
raphy. International Journal of Information Privacy,
Security and Integrity, 3(2):134–153.
Maher, K. (1995). Texto. URL:
ftp://ftp.funet.fi/pub/crypt/steganography/texto.
tar. gz.
Majeed, M. A., Sulaiman, R., Shukur, Z., and Hasan, M. K.
(2021). A review on text steganography techniques.
Mathematics, 9(21).
Mucherino, A., Papajorgji, P. J., and Pardalos, P. M. (2009).
K-nearest neighbor classification. In Data mining in
agriculture, pages 83–106. Springer.
Parmar, A., Katariya, R., and Patel, V. (2019). A Review on
Random Forest: An Ensemble Classifier. In Hemanth,
J., Fernando, X., Lafata, P., and Baig, Z., editors, Inter-
national Conference on Intelligent Data Communica-
tion Technologies and Internet of Things (ICICI) 2018,
pages 758–763, Cham. Springer International Publish-
ing.
Pearson, K. (1900). X. On the criterion that a given sys-
tem of deviations from the probable in the case of a
correlated system of variables is such that it can be
reasonably supposed to have arisen from random sam-
pling. The London, Edinburgh, and Dublin Philosoph-
ical Magazine and Journal of Science, 50(302):157–
175.
Plackett, R. L. (1983). Karl Pearson and the Chi-Squared
Test. International Statistical Review / Revue Interna-
tionale de Statistique, 51(1):59–72.
Ridley, D. R., Dominguez, P. S., and Walker, C. B. (1999).
English letter frequencies in transcribed speech versus
written samples. Perceptual and Motor Skills, 88(3
part 2):1181–1188.
Rosenblatt, F. (1958). The perceptron: a probabilistic model
for information storage and organization in the brain.
Psychological review, 65(6):386.
Rumelhart, D. E., Hinton, G. E., and Williams, R. J. (1986).
Learning representations by back-propagating errors.
nature, 323(6088):533–536.
Schapire, R. E. (2013). Explaining adaboost. In Empirical
inference, pages 37–52. Springer.
Simmons, G. J. (1984). The prisoners’ problem and the sub-
liminal channel. In Advances in Cryptology, pages 51–
67. Springer.
Swain, P. H. and Hauska, H. (1977). The decision tree classi-
fier: Design and potential. IEEE Transactions on Geo-
science Electronics, 15(3):142–147.
Taleby Ahvanooey, M., Li, Q., Hou, J., Rajput, A. R., and
Chen, Y. (2019). Modern text hiding, text steganalysis,
and applications: A comparative analysis. Entropy,
21(4).
Taskiran, C., Topkara, U., Topkara, M., and Delp, E. (2006).
Attacks on lexical natural language steganography sys-
tems. Proceedings of SPIE - The International Society
for Optical Engineering.
DMMLACS 2023 - 3rd International Special Session on Data Mining and Machine Learning Applications for Cyber Security
564