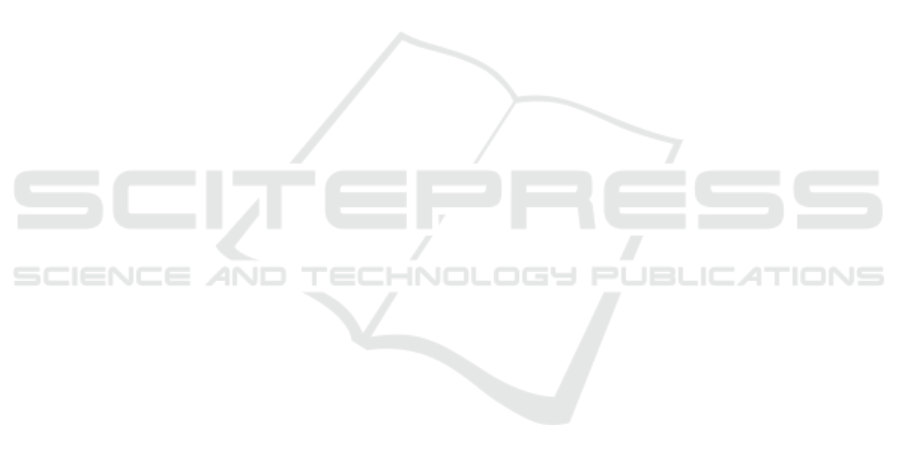
Technology Transfer: Theories, Concepts, and Prac-
tices in an Age of Complexity, pages 207–230.
Bliznets, I. A., Kartskhiya, A. A., and Smirnov, M. G.
(2018). Technology transfer in digital era: legal en-
vironment. Journal of History Culture and Art Re-
search, 7(1):354–363.
Cagnani, G. R., da Costa Oliveira, T., Mattioli, I. A.,
Sedenho, G. C., Castro, K. P., and Crespilho, F. N.
(2022). From research to market: correlation between
publications, patent filings, and investments in devel-
opment and production of technological innovations
in biosensors. Analytical and Bioanalytical Chem-
istry, pages 1–9.
Calvi, R., Pihlajamaa, M., and Servajean-Hilst, R. (2020).
Innovation scouting: a new challenge for the purchas-
ing function. The nature of purchasing: Insights from
research and practice, pages 295–313.
Chen, H., Zhang, G., Zhu, D., and Lu, J. (2015). A patent
time series processing component for technology in-
telligence by trend identification functionality. Neural
Computing and applications, 26:345–353.
Chen, X. and Han, T. (2019). Disruptive technology fore-
casting based on gartner hype cycle. In 2019 IEEE
technology & engineering management conference
(TEMSCON), pages 1–6. IEEE.
Chung, D. (2023). Machine learning for predictive model in
entrepreneurship research: predicting entrepreneurial
action. Small Enterprise Research, pages 1–18.
Conti, A., Thursby, J., and Thursby, M. (2013). Patents as
signals for startup financing. The Journal of Industrial
Economics, 61(3):592–622.
Dedehayir, O. and Steinert, M. (2016). The hype cycle
model: A review and future directions. Technologi-
cal Forecasting and Social Change, 108:28–41.
Desruelle, P. and Nepelski, D. (2017). The’innovation
radar’: A new policy tool to support innovation man-
agement. Available at SSRN 2944104.
Farre-Mensa, J., Hegde, D., and Ljungqvist, A. (2016). The
bright side of patents. Technical report, National Bu-
reau of Economic Research.
Ferri, S., Fiorentino, R., Parmentola, A., and Sapio, A.
(2019). Patenting or not? the dilemma of academic
spin-off founders. Business Process Management
Journal, 25(1):84–103.
Golovatchev, J. and Budde, O. (2010). Technology and in-
novation radar - effective instruments for the devel-
opment of a sustainable innovation strategy. In 2010
IEEE International Conference on Management of In-
novation and Technology, pages 760–764.
Gonz
´
alez-Pern
´
ıa, J. L., Kuechle, G., and Pe
˜
na-Legazkue,
I. (2013). An assessment of the determinants of uni-
versity technology transfer. Economic Development
Quarterly, 27(1):6–17.
Helmers, C. and Rogers, M. (2011). Does patenting help
high-tech start-ups? Research Policy, 40(7):1016–
1027.
Kalinowski, B. (2016). Increasing the potential for commer-
cialisation of innovation and research results within
polish universities. Modern Management Review,
21(23):2.
Kegel, P. (2016). A comparison of startup entrepreneurial
activity between the united states and japan. Journal
of Management Policy & Practice, 17(1).
Lee, M. and Lee, S. (2017). Identifying new business oppor-
tunities from competitor intelligence: An integrated
use of patent and trademark databases. Technological
Forecasting and Social Change, 119:170–183.
Montebruno, P., Bennett, R. J., Smith, H., and Van Lieshout,
C. (2020). Machine learning classification of en-
trepreneurs in british historical census data. Informa-
tion Processing & Management, 57(3):102210.
Rippa, P. and Secundo, G. (2019). Digital academic en-
trepreneurship: The potential of digital technologies
on academic entrepreneurship. Technological Fore-
casting and Social Change, 146:900–911.
Rivera-Kempis, C., Valera, L., and Sastre-Castillo, M. A.
(2021). Entrepreneurial competence: Using machine
learning to classify entrepreneurs. Sustainability,
13(15):8252.
Rohrbeck, R., Heuer, J., and Arnold, H. (2006). The tech-
nology radar - an instrument of technology intelli-
gence and innovation strategy. In 2006 IEEE Interna-
tional Conference on Management of Innovation and
Technology, volume 2, pages 978–983.
Sabahi, S. and Parast, M. M. (2020). The impact of en-
trepreneurship orientation on project performance: A
machine learning approach. International Journal of
Production Economics, 226:107621.
Schultz, C. (2021). Does technology scouting impact spin-
out generation? an action research study in the context
of an entrepreneurial university. New Perspectives in
Technology Transfer: Theories, Concepts, and Prac-
tices in an Age of Complexity, pages 107–128.
Siegel, D. S. and Wright, M. (2015). Academic en-
trepreneurship: time for a rethink? British journal
of management, 26(4):582–595.
Van Lente, H., Spitters, C., and Peine, A. (2013). Com-
paring technological hype cycles: Towards a the-
ory. Technological Forecasting and Social Change,
80(8):1615–1628.
Wang, C.-H. and Quan, X. I. (2021). The role of ex-
ternal technology scouting in inbound open innova-
tion generation: Evidence from high-technology in-
dustries. IEEE Transactions on Engineering Manage-
ment, 68(6):1558–1569.
Winnink, J., Tijssen, R. J., and Van Raan, A. (2019).
Searching for new breakthroughs in science: How ef-
fective are computerised detection algorithms? Tech-
nological Forecasting and Social Change, 146:673–
686.
KDIR 2023 - 15th International Conference on Knowledge Discovery and Information Retrieval
486