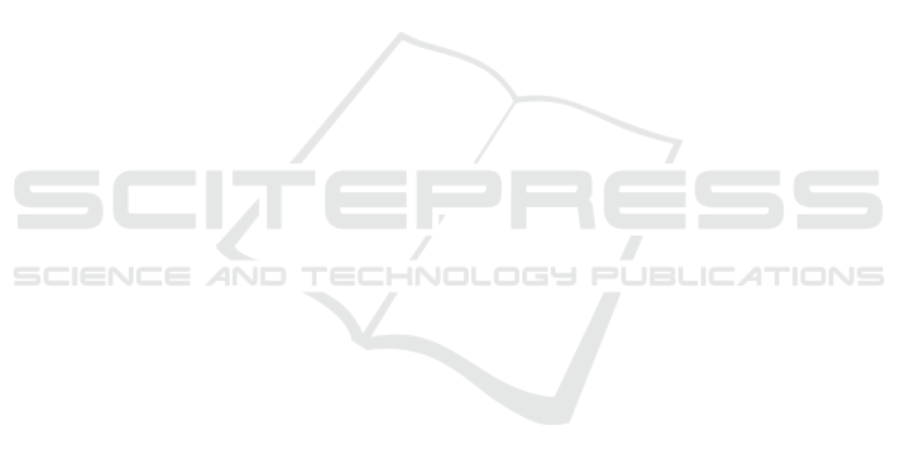
power electricity consumption using a high dimen-
sional dataset. Expert Systems with Applications,
187:115917.
Amber, K., Aslam, M., and Hussain, S. (2015). Electric-
ity consumption forecasting models for administration
buildings of the uk higher education sector. Energy
and Buildings, 90:127–136.
Amini, M. H., Kargarian, A., and Karabasoglu, O. (2016).
Arima-based decoupled time series forecasting of
electric vehicle charging demand for stochastic power
system operation. Electric Power Systems Research,
140:378–390.
Avami, A. and Boroushaki, M. (2011). Energy consumption
forecasting of iran using recurrent neural networks.
Energy Sources, Part B: Economics, Planning, and
Policy, 6(4):339–347.
Azeem, A., Ismail, I., Jameel, S. M., and Harindran, V. R.
(2021). Electrical load forecasting models for differ-
ent generation modalities: a review. IEEE Access,
9:142239–142263.
Chen, J., Ji, P., and Lu, F. (2010). Exponential smoothing
method and its application to load forecasting. Jour-
nal of China Three Gorges University (Natural Sci-
ence Edition), 32(03):37–4.
Deb, C., Zhang, F., Yang, J., Lee, S. E., and Shah, K. W.
(2017). A review on time series forecasting techniques
for building energy consumption. Renewable and Sus-
tainable Energy Reviews, 74:902–924.
Debusschere, V., Bacha, S., et al. (2012). One week
hourly electricity load forecasting using neuro-fuzzy
and seasonal arima models. IFAC Proceedings Vol-
umes, 45(21):97–102.
Fumo, N. and Biswas, M. R. (2015). Regression analysis
for prediction of residential energy consumption. Re-
newable and sustainable energy reviews, 47:332–343.
Ganesan, P., Rajakarunakaran, S., Thirugnanasambandam,
M., and Devaraj, D. (2015). Artificial neural network
model to predict the diesel electric generator perfor-
mance and exhaust emissions. Energy, 83:115–124.
Ghalehkhondabi, I., Ardjmand, E., Weckman, G. R., and
Young, W. A. (2017). An overview of energy demand
forecasting methods published in 2005–2015. Energy
Systems, 8:411–447.
Groß, A., Lenders, A., Schwenker, F., Braun, D. A., and
Fischer, D. (2021). Comparison of short-term elec-
trical load forecasting methods for different building
types. Energy Informatics, 4:1–16.
Hamzac¸ebi, C., Es, H. A., and C¸ akmak, R. (2019). Fore-
casting of turkey’s monthly electricity demand by sea-
sonal artificial neural network. Neural Computing and
Applications, 31:2217–2231.
Haq, M. R. and Ni, Z. (2019). A new hybrid model for
short-term electricity load forecasting. IEEE access,
7:125413–125423.
Hong, T., Pinson, P., Fan, S., Zareipour, H., Troccoli, A.,
and Hyndman, R. J. (2016). Probabilistic energy fore-
casting: Global energy forecasting competition 2014
and beyond.
Khan, Z. A., Ullah, A., Haq, I. U., Hamdy, M., Mauro,
G. M., Muhammad, K., Hijji, M., and Baik, S. W.
(2022). Efficient short-term electricity load forecast-
ing for effective energy management. Sustainable En-
ergy Technologies and Assessments, 53:102337.
Kuster, C., Rezgui, Y., and Mourshed, M. (2017). Electrical
load forecasting models: A critical systematic review.
Sustainable cities and society, 35:257–270.
Lam, J. C., Tang, H. L., and Li, D. H. (2008). Seasonal vari-
ations in residential and commercial sector electricity
consumption in hong kong. Energy, 33(3):513–523.
Lisi, F. and Edoli, E. (2018). Analyzing and forecasting
zonal imbalance signs in the italian electricity market.
The Energy Journal, 39(5).
Nepal, B., Yamaha, M., Yokoe, A., and Yamaji, T. (2020).
Electricity load forecasting using clustering and arima
model for energy management in buildings. Japan Ar-
chitectural Review, 3(1):62–76.
Nguyen, H. and Hansen, C. K. (2017). Short-term elec-
tricity load forecasting with time series analysis. In
2017 IEEE International Conference on Prognostics
and Health Management (ICPHM), pages 214–221.
IEEE.
Outlook, A. E. et al. (2010). Energy information adminis-
tration. Department of Energy, 92010(9):1–15.
Phan, Q. T., Wu, Y. K., and Phan, Q. D. (2021). A hybrid
wind power forecasting model with xgboost, data pre-
processing considering different nwps. Applied Sci-
ences, 11(3):1100.
Rossi, M. and Brunelli, D. (2013). Electricity demand fore-
casting of single residential units. In 2013 IEEE Work-
shop on Environmental Energy and Structural Moni-
toring Systems, pages 1–6. IEEE.
Singh, A. K., Khatoon, S., Muazzam, M., Chaturvedi,
D., et al. (2012). Load forecasting techniques and
methodologies: A review. In 2012 2nd International
Conference on Power, Control and Embedded Sys-
tems, pages 1–10. IEEE.
Suganthi, L. and Samuel, A. A. (2012). Energy models for
demand forecasting—a review. Renewable and sus-
tainable energy reviews, 16(2):1223–1240.
Tian, C. and Hao, Y. (2018). A novel nonlinear com-
bined forecasting system for short-term load forecast-
ing. Energies, 11(4):712.
Wang, Y., Sun, S., Chen, X., Zeng, X., Kong, Y., Chen,
J., Guo, Y., and Wang, T. (2021). Short-term load
forecasting of industrial customers based on svmd and
xgboost. International Journal of Electrical Power &
Energy Systems, 129:106830.
Wang, Y., Wang, J., Zhao, G., and Dong, Y. (2012). Appli-
cation of residual modification approach in seasonal
arima for electricity demand forecasting: A case study
of china. Energy Policy, 48:284–294.
Xiong, X., Hu, X., and Guo, H. (2021). A hybrid optimized
grey seasonal variation index model improved by
whale optimization algorithm for forecasting the resi-
dential electricity consumption. Energy, 234:121127.
Yukseltan, E., Yucekaya, A., and Bilge, A. H. (2017). Fore-
casting electricity demand for turkey: Modeling peri-
odic variations and demand segregation. Applied En-
ergy, 193:287–296.
NCTA 2023 - 15th International Conference on Neural Computation Theory and Applications
466