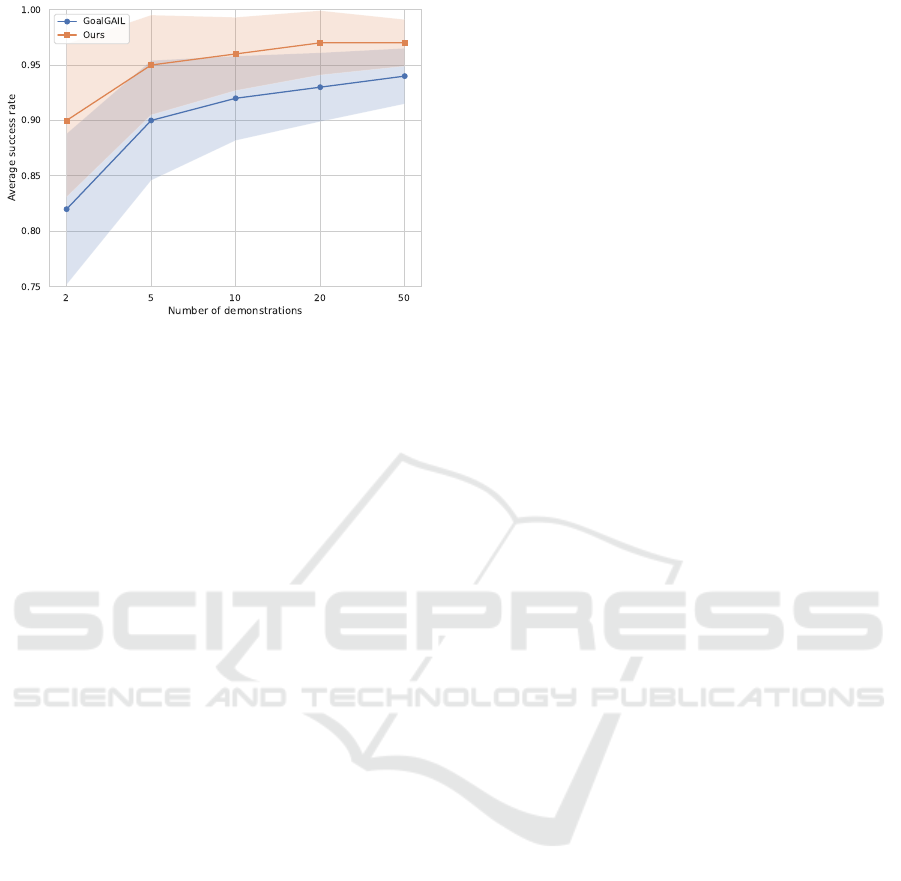
Figure 7: Average success rate for different numbers of
demonstrations: Our method is more resilient to the de-
crease of the number of demonstrations.
and make a step toward achieving more reliable imi-
tation learning using causality.
ACKNOWLEDGEMENTS
This work was funded in part by the region of Brittany
under the ROGAN project. We are grateful for their
support, which made this research possible.
REFERENCES
Andrychowicz, M., Wolski, F., Ray, A., Schneider, J., Fong,
R., Welinder, P., McGrew, B., Tobin, J., Pieter Abbeel,
O., and Zaremba, W. (2017). Hindsight experience re-
play. Advances in neural information processing sys-
tems, 30.
Arjovsky, M. and Bottou, L. (2017). Towards principled
methods for training generative adversarial networks.
arXiv preprint arXiv:1701.04862.
Bain, M. and Sammut, C. (1999). A framework for be-
havioural cloning. In Machine Intelligence 15, Intel-
ligent Agents [St. Catherine’s College, Oxford, July
1995], page 103–129, GBR. Oxford University.
Ding, Y., Florensa, C., Abbeel, P., and Phielipp, M. (2019).
Goal-conditioned imitation learning. Advances in
neural information processing systems, 32.
Hare, J. (2019). Dealing with sparse rewards in reinforce-
ment learning. arXiv preprint arXiv:1910.09281.
Ho, J. and Ermon, S. (2016). Generative adversarial imi-
tation learning. Advances in neural information pro-
cessing systems, 29.
Kaddour, J., Lynch, A., Liu, Q., Kusner, M. J., and Silva, R.
(2022). Causal machine learning: A survey and open
problems. arXiv preprint arXiv:2206.15475.
Kingma, D. P. and Welling, M. (2013). Auto-encoding vari-
ational bayes. arXiv preprint arXiv:1312.6114.
Lillicrap, T. P., Hunt, J. J., Pritzel, A., Heess, N., Erez, T.,
Tassa, Y., Silver, D., and Wierstra, D. (2015). Contin-
uous control with deep reinforcement learning. arXiv
preprint arXiv:1509.02971.
Liu, M., Zhu, M., and Zhang, W. (2022). Goal-
conditioned reinforcement learning: Problems and so-
lutions. arXiv preprint arXiv:2201.08299.
Mnih, V., Kavukcuoglu, K., Silver, D., Graves, A.,
Antonoglou, I., Wierstra, D., and Riedmiller, M.
(2013). Playing atari with deep reinforcement learn-
ing. arXiv preprint arXiv:1312.5602.
Pearl, J. (2013). Structural counterfactuals: A brief intro-
duction. Cognitive science, 37(6):977–985.
Pearl, J. et al. (2000). Models, reasoning and inference.
Cambridge, UK: CambridgeUniversityPress, 19(2):3.
Plappert, M., Andrychowicz, M., Ray, A., McGrew, B.,
Baker, B., Powell, G., Schneider, J., Tobin, J.,
Chociej, M., Welinder, P., Kumar, V., and Zaremba,
W. (2018). Multi-goal reinforcement learning: Chal-
lenging robotics environments and request for re-
search. CoRR, abs/1802.09464.
Robinson, J. D., Chuang, C.-Y., Sra, S., and Jegelka, S.
(2021). Contrastive learning with hard negative sam-
ples. In International Conference on Learning Repre-
sentations.
Russell, S. (1998). Learning agents for uncertain environ-
ments. In Proceedings of the eleventh annual confer-
ence on Computational learning theory, pages 101–
103.
Sch
¨
olkopf, B. (2019). Causality for machine learning.
CoRR, abs/1911.10500.
Shah, H., Tamuly, K., Raghunathan, A., Jain, P., and Netra-
palli, P. (2020). The pitfalls of simplicity bias in neural
networks. Advances in Neural Information Processing
Systems, 33:9573–9585.
Silver, D., Huang, A., Maddison, C. J., Guez, A., Sifre, L.,
Van Den Driessche, G., Schrittwieser, J., Antonoglou,
I., Panneershelvam, V., Lanctot, M., et al. (2016).
Mastering the game of go with deep neural networks
and tree search. nature, 529(7587):484–489.
Sutton, R. S. and Barto, A. G. (2018). Reinforcement learn-
ing: An introduction. MIT press.
Sutton, R. S., McAllester, D., Singh, S., and Mansour, Y.
(1999). Policy gradient methods for reinforcement
learning with function approximation. Advances in
neural information processing systems, 12.
Improving Reward Estimation in Goal-Conditioned Imitation Learning with Counterfactual Data and Structural Causal Models
337