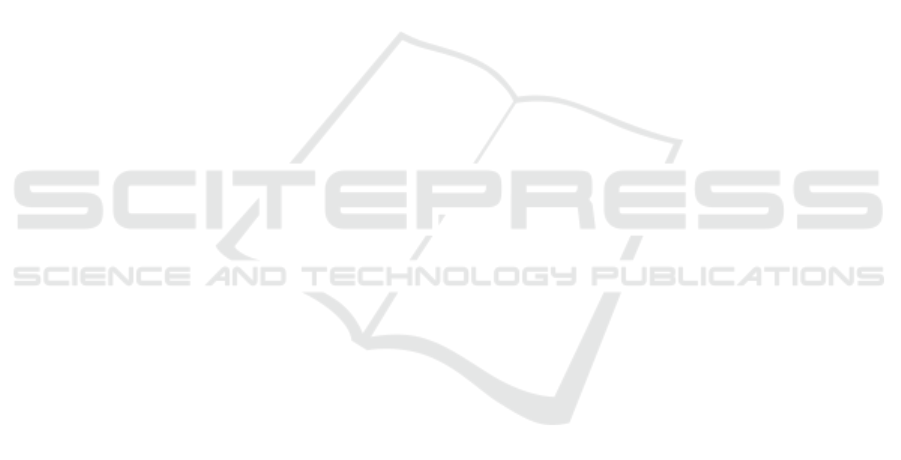
5 CONCLUSIONS
This paper applies evolutionary game theory to study
the route choice behavior of tourist groups with
different perceptions of crowding within a scenic
area. The influence of guidance information on route
choice is analyzed, considering various strategies of
information supply. A game model is constructed to
examine the evolutionary stable states of tourist route
choices under the impact of guidance information.
Dynamic equations are used to analyze the long-term
stability evolution trend of the scenic tours system.
Numerical experiments are conducted to simulate the
effects of different guidance information strategies on
the system's evolution. The main conclusions are as
follows: (1) Without guidance information, all
tourists choose the original route (unique ESS). (2)
With guidance information, the uniqueness of ESS
depends on the size of payoffs loss caused by
congestion revealed in the information: (a) When the
payoffs loss from congestion is small, all tourists still
choose the original route (little effect from guidance
information); (b) When the payoffs loss from
congestion falls between the detouring payoffs loss of
two tourist groups, tourists with low crowding
perception choose the original route and those with
high crowding perception choose alternative routes
(successful guidance information to ease congestion);
(c) When the payoffs loss from congestion exceeds
the detouring payoffs loss of both groups, the ESS for
route choice becomes non-unique, with each group
choosing different routes to avoid congestion.
ACKNOWLEDGMENTS
This work was supported by Humanities and social
sciences fund of the Ministry of Education
(No.19YJC630119).
REFERENCES
R. Chen, C.Y. Liang, W.C. Hong, D.X. Gu, Forecasting
holiday daily tourist flow based on seasonal support
vector regression with adaptive genetic algorithm(J).
Appl. Soft Comput., 2015, 26: 435-443.
https://doi.org/10.1016/j.asoc.2014.10.022
A. G. Assaf, G. Li, H. Song, M. G. Tsionas, Modeling and
forecasting regional tourism demand using the Bayesian
global vector autoregressive (BGVAR) model (J). J.
Travel Res, 2019, 58(3): 383-397,
https://doi.org/10.1177/0047287518759226
X. Yang, B. Pan, J. A. Evans, B. Lv. Forecasting Chinese
tourist volume with search engine data (J). Tourism
Manage., 2015, 46, 386-397.
https://doi.org/10.1016/j.tourman.2014.07.019
S. Li, T. Chen, L. Wang, C. Ming, Effective tourist volume
forecasting supported by PCA and improved BPNN
using Baidu index (J). Tourism Manage., 2018, 68: 116-
126. https://doi.org/10.1016/j.tourman.2018.03.006
S. Sun, Y. Wei, K. L. Tsui, S. Wang, Forecasting tourist
arrivals with machine learning and internet search index
(J). Tourism Manage., 2019, 70:1-10.
https://doi.org/10.1016/j.tourman.2018.07.010
W. Zheng, P. Ren, P. Ge, Q. An, M. Jin, Tourist
management based on behaviour characteristics during
peak travel period - a case study of Jiuzhaigou (J). Int.
J. Environ. Pollut., 2013, 51(3-4):222-237.
https://doi.org/10.1504/IJEP.2013.054031
J. Kamruzzaman, G.Karmakar, A dynamic content
distribution scheme for decentralized sharing in tourist
hotspots (J). J.Netw. Comput. Appl., 2019, 129, 9-24.
https://doi.org/10.1016/j.jnca.2018.12.011
F. M. Hsu, Y. T. Lin, T. K. Ho, Design and implementation
of an intelligent recommendation system for tourist
attractions: The integration of EBM model, Bayesian
network and Google Maps (J). Expert Syst. Appl., 2012,
39(3): 3257-3264.
https://doi.org/10.1016/j.eswa.2011.09.013
J. Ruiz-Meza, J. R. Montoya-Torres, Tourist trip design
with heterogeneous preferences, transport mode
selection and environmental considerations. Ann. Oper.
Res., 2021, 305(1-2): 227-249.
https://doi.org/10.1007/s10479-021-04209-7
I. Y.Choi, Y. U.Ryu, J. K. Kim, A recommender system
based on personal constraints for smart tourism city(J).
Asia Pac. J. Tourism Res., 2021, 26(4): 440-453.
https://doi.org/10.1080/10941665.2019.1592765
Z. Liao, W. Zheng, Using a heuristic algorithm to design a
personalized day tour route in a time-dependent
stochastic environment (J). Tourism Manage., 2018,
68:284-300.
https://doi.org/10.1016/j.tourman.2018.03.012
W. Zheng, H. Ji, C. Lin, W. Wang, B. Yu, Using a heuristic
approach to design personalized urban tourism
itineraries with hotel selection (J). Tourism Manage.,
2020, 76:103956.
https://doi.org/10.1016/j.tourman.2019.103956
Analysis of Tourists’ Route Selection in Scenic Areas Based on Game Theory Model
349