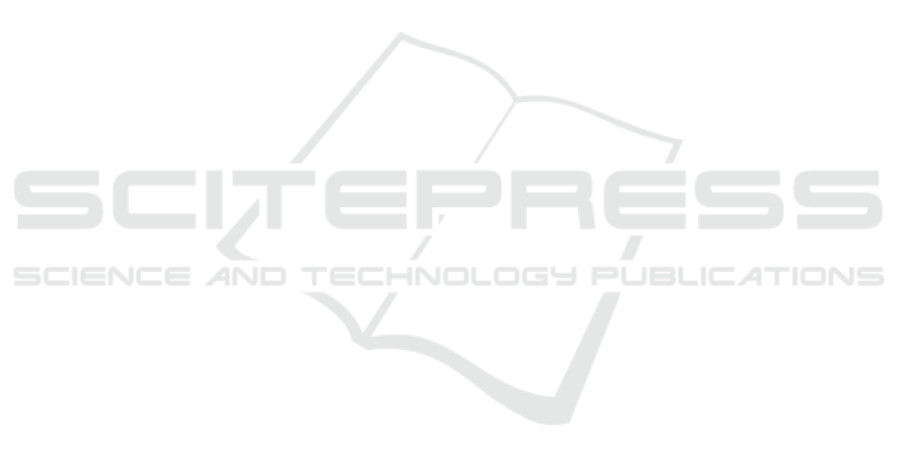
Text-Guided Salient Object Detection
Zixian Xu
1
, Luanqi Liu
1,*
, Yingxun Wang
1
, Xue Wang
1
and Pu Li
2
1
Qilu Institute of Technology, Shandong, China
2
Guangzhou College of Technology and Business, Guangzhou, China
Keywords: Salient Object Detection, Natural Language.
Abstract: Salient object detection (SOD), a core task in the field of computer vision, is dedicated to accurately
identifying the salient objects in images. Unlike previous research methods, this study recognizes the key role
of textual information in salient object detection and thus proposes a unique text-based range control method
for salient object detection. In this method, we introduce the semantic labels from the CoSOD3K dataset into
a pre-trained text-driven semantic segmentation model to align the textual and image feature information.
Subsequently, the image features are analyzed for saliency through a salient object detection network.
Through the SFE (Salient Feature Extractor) module, we fuse the extracted salient features with the
semantically aligned features to derive the saliency detection results. Experimental results show that the
robustness and efficiency of our framework surpass existing salient object detection methods. Users can guide
the detection process through natural language interaction, expanding applications such as image editing and
data annotation, and to some extent solving challenges like complex backgrounds, multi-scale issues, and
blurry boundaries. This offers the potential for new breakthroughs in the field of salient object detection.
1 INTRODUCTION
The goal of computer vision is to enable machines to
"see" and "understand" their environment, with
salient object detection being one of its important
tasks. The aim of this task is to identify salient, eye-
catching objects within images. These objects attract
the observer's attention due to their distinctiveness or
differences in context.
Traditional salient object detection methods
mainly rely on low-level visual cues or deep learning
techniques to extract and analyse image features.
However, these methods often face difficulties when
dealing with complex backgrounds, multi-scale
issues, and blurred boundaries. Moreover, they
frequently overlook the value of text information in
enhancing detection performance.
To address this issue, we propose a new solution
a text-based salient object detection range control
method. In this approach, we incorporate the semantic
labels from the CoSOD3k dataset (Fan D P, 2021)
into a pre-trained text-driven semantic segmentation
model to align text information with image feature
information. Then, we utilise a salient object
detection network to conduct saliency analysis on the
image features.
Through the SFE module, we fuse the extracted
saliency features with the semantically aligned
features to derive the saliency detection results.
Experimental results show that our framework
outperforms existing salient object detection methods
in terms of robustness and efficiency. Additionally,
the detection process can be guided by natural
language interaction, opening up new possibilities for
applications such as image editing, data annotation,
and more.
With this study, we aim to provide an effective
solution to the challenges of salient object detection
in complex backgrounds, multi-scale issues, and
blurred boundaries, paving the way for new
breakthroughs and opportunities in the field of salient
object detection.
Our contributions can be summarized as follows:
1. We propose a novel text-guided salient object
detection framework that integrates natural language
information to guide the detection process, expanding
possible applications such as image editing and data
annotation.
2. This research introduces the SFE module,
which combines salient features with semantically
aligned features and uses upsampling techniques to
derive saliency detection results. This innovative
Xu, Z., Liu, L., Wang, Y., Wang, X. and Li, P.
Text-Guided Salient Object Detection.
DOI: 10.5220/0012284400003807
Paper published under CC license (CC BY-NC-ND 4.0)
In Proceedings of the 2nd International Seminar on Artificial Intelligence, Networking and Information Technology (ANIT 2023), pages 381-385
ISBN: 978-989-758-677-4
Proceedings Copyright © 2024 by SCITEPRESS – Science and Technology Publications, Lda.
381