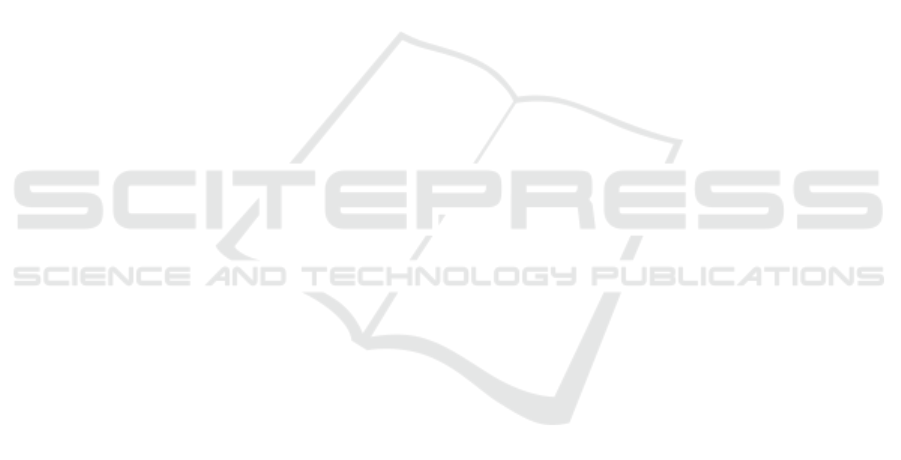
develop efficient data storage and processing
technologies, improve the security and privacy of the
system, and provide better support for scientific
research communication.
4 CONCLUSION
In summary, the analysis of the communication mode
and system design of scientific research in vocational
colleges and universities based on new media big data
is a work of great significance. Through the research
of this paper, we find that the impact of new media
big data on the communication mode of scientific
research work is mainly reflected in the
communication speed, content richness and audience
targeting. In view of these problems, we design a
scientific research work dissemination system based
on new media big data, and verify its effectiveness
and feasibility through practice. In the future, we will
continue to study the application of the system in
other fields and make more contributions to
promoting digital development.
REFERENCES
Rivas, J. G., Carrion, D. M., Tortolero, L., Veneziano, D.,
Esperto, F. Scientific social media, A new way to
expand knowledge. What do urologists need to know?
Actas Urologicas Espanolas(J), 2019, 43(5), 269-276.
https://doi.org/10.1016/j.acuro.2018.12.003
O'Keefe, C. M., & Head, R. J. Application of logic models
in a large scientific research program. Evaluation and
Program Planning (J), 2011, 34(3), 174-184.
https://doi.org/10.1016/j.evalprogplan. 2011.02.008
Julpisit, A., & Esichaikul, V. A collaborative system to
improve knowledge sharing in scientific research
projects. Information Development (J), 2019, 35(4),
624-638. https://doi.org/10.1177/026 66669 18779240
Zhao, W. B., Yin, Z. X., Fan, T. R., & Luo, J. S. Research
on influence spread of scientific research team based on
scientific factor quantification of big data. International
Journal of Distributed Sensor Networks (J), 2019,
15(4). https://doi.org/10.1177/1550147719842158
Xin, C. C., & He, C. H. Research on university scientific
research patent management information system based
on BS mode. Journal of Intelligent & Fuzzy Systems
(J), 2020, 38(2), 1371-1379.
https://doi.org/10.3233/jifs-179500
Weller, K. Accepting the challenges of social media
research. Online Information Review (J), 2015, 39(3),
281-289. https://doi.org/10.1108/oir-03-2015-0069
Liu, L., & Luan, J. Survey analysis and discussion on
cultivating scientific research quality among
undergraduates in medical colleges. Pharmacology
Research & Perspectives (J), 2023, 11(3).
https://doi.org/10.1002/prp2.1095
Chen, C., Zhe, C., Zheng, Y. Y., Xiong, X., Xiao, T., & Lu,
X. F. Evaluation of Scientific Research in Universities
Based on the Theories for Sustainable Competitive
Advantage. Sage Open (J), 2023, 13(2).
https://doi.org/10.1177/21582440231177048
De Winter, J., & Kosolosky, L. The Epistemic Integrity of
Scientific Research. Science and Engineering Ethics
(J), 2013, 19(3), 757-774.
https://doi.org/10.1007/s11948-012-9394-3
Levine, F. J., & Iutcovich, J. M. Challenges in studying the
effects of scientific societies on research integrity.
Science and Engineering Ethics (J), 2003, 9(2), 257-
268. https://doi.org/10.1007/s11948-003-0012-2
Post, R. Constitutional Restraints on the Regulations of
Scientific Speech and Scientific Research. Science and
Engineering Ethics (J), 2009, 15(3), 431-438.
https://doi.org/10.1007/s11948-009-9133-6
Saeed-Ul, H., Imran, M., Gillani, U., Aljohani, N. R.,
Bowman, T. D., & Didegah, F. Measuring social media
activity of scientific literature: an exhaustive
comparison of scopus and novel altmetrics big data.
Scientometrics(J), 2017, 113(2), 1037-1057.
https://doi.org/10.1007/s11192-017-2512-x
Romano, R., & Davino, C. Assessing scientific research
activity evaluation models using multivariate analysis.
Statistics and Its Interface (J), 2016, 9(3), 303-313.
https://doi.org/10.4310 /SII .201 6.v9.n 3.a5
Shi, Y. K., Wang, D. C., & Zhang, Z. M. Categorical
Evaluation of Scientific Research Efficiency in Chinese
Universities: Basic and Applied Research.
Sustainability (J), 2022, 14(8).
https://doi.org/10.3390/su14084402
ANIT 2023 - The International Seminar on Artificial Intelligence, Networking and Information Technology
430