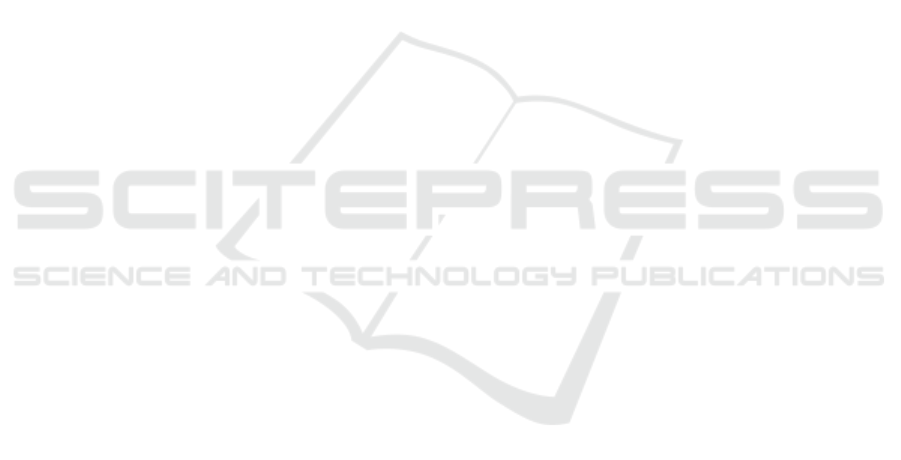
5 CONCLUSIONS
DL has revolutionized computer vision and robotics
by enabling remarkable advancements in perception
tasks. However, as discussed in this paper, a signif-
icant limitation persists in many existing DL-based
systems: the static inference paradigm. Most DL
models operate on fixed, static inputs, neglecting the
potential benefits of active perception – a process that
mimics how humans and certain animals interact with
their environment to better understand it. Active per-
ception offers advantages in terms of accuracy and ef-
ficiency, making it a crucial area of exploration for
enhancing robotic perception. While the incorpora-
tion of deep learning and active perception in robotics
presents numerous opportunities, it also poses sev-
eral challenges. Training often necessitates interac-
tive simulation environments and more advanced ap-
proaches like deep reinforcement learning. Moreover,
deployment pipelines need to be adapted to enable
control within perception algorithms. These chal-
lenges highlight the importance of ongoing research
and development in this field.
ACKNOWLEDGMENTS
This work was supported by the European Union’s
Horizon 2020 Research and Innovation Program
(OpenDR) under Grant 871449. This publication re-
flects the authors’ views only. The European Com-
mission is not responsible for any use that may be
made of the information it contains.
REFERENCES
Aloimonos, Y. (2013). Active perception. Psychology Press.
Ammirato, P., Poirson, P., Park, E., Ko
ˇ
seck
´
a, J., and Berg,
A. C. (2017). A dataset for developing and bench-
marking active vision. In Proceedings of the IEEE In-
ternational Conference on Robotics and Automation,
pages 1378–1385.
Badrinarayanan, V., Kendall, A., and Cipolla, R. (2017).
Segnet: A deep convolutional encoder-decoder ar-
chitecture for image segmentation. IEEE Transac-
tions on Pattern Analysis and Machine Intelligence,
39(12):2481–2495.
Bajcsy, R., Aloimonos, Y., and Tsotsos, J. K. (2018).
Revisiting active perception. Autonomous Robots,
42(2):177–196.
Bojarski, M., Del Testa, D., Dworakowski, D., Firner,
B., Flepp, B., Goyal, P., Jackel, L. D., Monfort,
M., Muller, U., Zhang, J., et al. (2016). End to
end learning for self-driving cars. arXiv preprint
arXiv:1604.07316.
Bozinis, T., Passalis, N., and Tefas, A. (2021). Improv-
ing visual question answering using active perception
on static images. In Proceedings of the International
Conference on Pattern Recognition, pages 879–884.
Buckman, J., Hafner, D., Tucker, G., Brevdo, E., and Lee,
H. (2018). Sample-efficient reinforcement learning
with stochastic ensemble value expansion. Proceed-
ings of the Advances in Neural Information Process-
ing Systems, 31.
Dimaridou, V., Passalis, N., and Tefas, A. (2023). Deep ac-
tive robotic perception for improving face recognition
under occlusions. In Proceedings of the IEEE Sympo-
sium Series on Computational Intelligence (accepted),
page 1.
Georgiadis, C., Passalis, N., and Nikolaidis, N. (2023). Ac-
tiveface: A synthetic active perception dataset for face
recognition. In Proceedings of the International Work-
shop on Multimedia Signal Processing (accepted),
page 1.
Ginargyros, S., Passalis, N., and Tefas, A. (2023). Deep ac-
tive perception for object detection using navigation
proposals. In Proceedings of the IEEE Symposium Se-
ries on Computational Intelligence (accepted), page 1.
Haas, J. K. (2014). A history of the unity game engine.
Han, X., Liu, H., Sun, F., and Zhang, X. (2019). Active
object detection with multistep action prediction us-
ing deep q-network. IEEE Transactions on Industrial
Informatics, 15(6):3723–3731.
Heffner, R. S. and Heffner, H. E. (1992). Evolution of sound
localization in mammals. In The evolutionary biology
of hearing, pages 691–715.
Hua, J., Zeng, L., Li, G., and Ju, Z. (2021). Learning for
a robot: Deep reinforcement learning, imitation learn-
ing, transfer learning. Sensors, 21(4):1278.
Kakaletsis, E. and Nikolaidis, N. (2023). Using synthesized
facial views for active face recognition. Machine Vi-
sion and Applications, 34(4):62.
LeCun, Y., Bengio, Y., and Hinton, G. (2015). Deep learn-
ing. Nature, 521(7553):436–444.
Li, J.-h. (2018). Cyber security meets artificial intelligence:
a survey. Frontiers of Information Technology & Elec-
tronic Engineering, 19(12):1462–1474.
Liu, Q., Liu, Z., Xu, W., Tang, Q., Zhou, Z., and Pham, D. T.
(2019). Human-robot collaboration in disassembly for
sustainable manufacturing. International Journal of
Production Research, 57(12):4027–4044.
Loukas, G., Vuong, T., Heartfield, R., Sakellari, G., Yoon,
Y., and Gan, D. (2017). Cloud-based cyber-physical
intrusion detection for vehicles using deep learning.
IEEE Access, 6:3491–3508.
Manousis, T., Passalis, N., and Tefas, A. (2023). Enabling
high-resolution pose estimation in real time using ac-
tive perception. In Proceedings of the IEEE Interna-
tional Conference on Image Processing, pages 2425–
2429.
Michel, O. (2004). Cyberbotics ltd. webots™: professional
mobile robot simulation. International Journal of Ad-
vanced Robotic Systems, 1(1):5.
Mnih, V., Kavukcuoglu, K., Silver, D., Graves, A.,
Antonoglou, I., Wierstra, D., and Riedmiller, M.
IJCCI 2023 - 15th International Joint Conference on Computational Intelligence
20