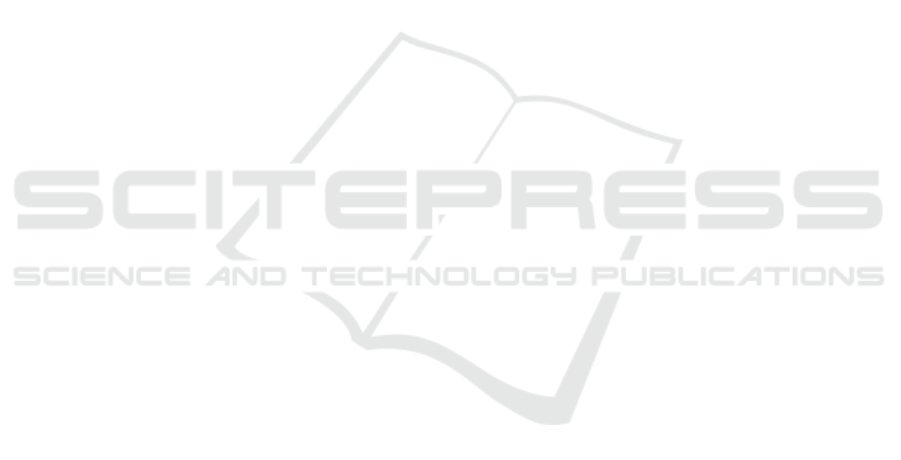
4 CONCLUSION
The preprocessing process for forest fire prediction
data research has been obtained from the forest fire
prediction research using data selection, data clean-
ing, and feature selection. Conventional machine
learning data mining approaches can process data
well with the multilayer perceptron method with the
parameters train 0.7 and test 0.3. The multilayer per-
ceptron method yields an accuracy of 86.70% and an
F1 score of 87.93% with a hidden layer size of 32.32,
which is higher than the Random Forest, Decision
Tree, Logistic Regression, and Nave Bayes methods.
This value is quite dominant compared to other meth-
ods. This research can determine the proportion of
the possibility of forest fires occurring, and it is antic-
ipated that in future research, it can be developed by
deepening the size of the hidden layer for more accu-
rate reporting of forest fires.
REFERENCES
Agustyaningrum, C., Gata, W., Nurfalah, R., and Radiyah,
U. (2020). Komparasi algoritma naive bayes , random
forest dan svm untuk memprediksi niat. J. Inform,
20(2).
Agustyaningrum, C., Haris, M., Aryanti, R., and Misriati, T.
(2021). Online shopper intention analysis using con-
ventional machine learning and deep neural network
classification algorithm. J. Penelit. Pos dan Inform,
11(1):89–100,.
Ayuningtyas, F. and Prasetyo, S. (2020). Pemanfaatan
teknologi machine learning untuk klasifikasi wilayah
risiko kekeringan di daerah istimewa yogyakarta
menggunakan citra landsat 8 operational land imager
(oli. J. Transform, 18(1):13,.
Baranovskiy, N. and Zharikova, M. (2014). A web-oriented
geoinformation system application for forest fire dan-
ger prediction in typical forests of the ukraine. Lect.
Notes Geoinf. Cartogr, 0(199669):13–22,.
Cortez, P. and Morais, A. (2007). A data mining approach to
predict forest fires using meteorological data. In Proc.
13th Port. Conf. Artif. Intell, pages 512–523,. Online].
Available:.
Husen, D., Sandi, D., and Bumbungan, S. (2022). Analisis
prediksi kebakaran hutan dengan menggunakan algo-
ritma random forest classifier kebakaran hutan dan la-
han di indonesia telah menjadi perhatian dunia inter-
nasional khususnya sejak kebakaran hutan yang ter-
jadi pada tahun 80-an [ 2 ]. penyebab kebaka.
Irfan, M., Sumbodo, B., and Candradewi, I. (2017). Sistem
klasifikasi kendaraan berbasis pengolahan citra digital
dengan metode multilayer perceptron. IJEIS (Indone-
sian J. Electron. Instrum. Syst, 7(2):139,.
Kabir, M., Ashraf, F., and Ajwad, R. (2019). Analysis of
different predicting model for online shoppers’ pur-
chase intention from empirical data. Conf. Comput.
Inf. Technol. ICCIT.
Leonardo, R., Pratama, J., and Chrisnatalis, C. (2020).
Perbandingan metode random forest dan na
¨
ıve bayes
dalam prediksi keberhasilan klien telemarketing. J.
Teknol. Dan Ilmu Komput. Prima, 3(2):1–5,.
Manalu, D., Zarlis, M., Mawengkang, H., and Sitompul, O.
(2021). Forest fire prediction in northern sumatera us-
ing support vector machine based on the fire weather
index. In no, pages 187–196,.
Negoro, N., diana, M., Ula, M., and Insani, F. (2022).
Analisis kebakaran pada hutan dan lokasi lahan di
provinsi riau menggunakan metode c4.5. Maret,
7(1):107–114,.
Nurachim, R. (2019). Pemilihan model prediksi indeks
harga saham yang dikembangkan berdasarkan algo-
ritma support vector machine ( svm ) atau multilayer
perceptron ( mlp ) studi kasus : Saham pt telekomu-
nikasi indonesia tbk.
Pham, B. (2020). Performance evaluation of machine learn-
ing methods for forest fire modeling and prediction.
Symmetry (Basel, 12(6):1–21,.
Pratiwi, T., Irsyad, M., and Kurniawan, R. (2021). Klasi-
fikasi kebakaran hutan dan lahan menggunakan algo-
ritma na
¨
ıve bayes (studi kasus: Provinsi riau. J. Sist.
dan Teknol. Inf, 9(2):101,.
Ratnawati, L. and Sulistyaningrum, D. (2019). Penera-
pan random forest untuk mengukur tingkat keparahan
penyakit. J. Sains Dan Seni Its, 8(2):71– 77,.
Saputra, R., Puspitasari, D., and Baidawi, T. (2022). De-
teksi kematangan buah melon dengan algoritma sup-
port vector machine berbasis ekstraksi fitur glcm.
Sarofi, M., Irhamah, I., and Mukarromah, A. (2020). Identi-
fikasi genre musik dengan menggunakan metode ran-
dom forest. J. Sains dan Seni ITS, 9(1):79–86,.
Shah, K., Patel, H., Sanghvi, D., and Shah, M. (2020). A
comparative analysis of logistic regression, random
forest and knn models for the text classification. Aug-
ment. Hum. Res, 5(1).
Yandi, J., Kurniawan, T., Negara, E., and Akbar, M. (2021).
Prediksi lokasi titik panas kebaran hutan menggu-
nakan model regresion svm (support vector machine)
pada data kebakaran hutan daops manggala agni oki
provinsi sumatera selatan tahun 2019. InfoTekJar J.
Nas. Inform. dan Teknol. Jar, 6(1):10–15,.
Zulfikar, W. and Lukman, N. (2016). Perbandingan naive
bayes classifier dengan nearest neighbor untuk identi-
fikasi penyakit mata. J. Online Inform, 1(2):82–86,.
Forest Fire Data Analysis Using Conventional Machine Learning Algorithms
37