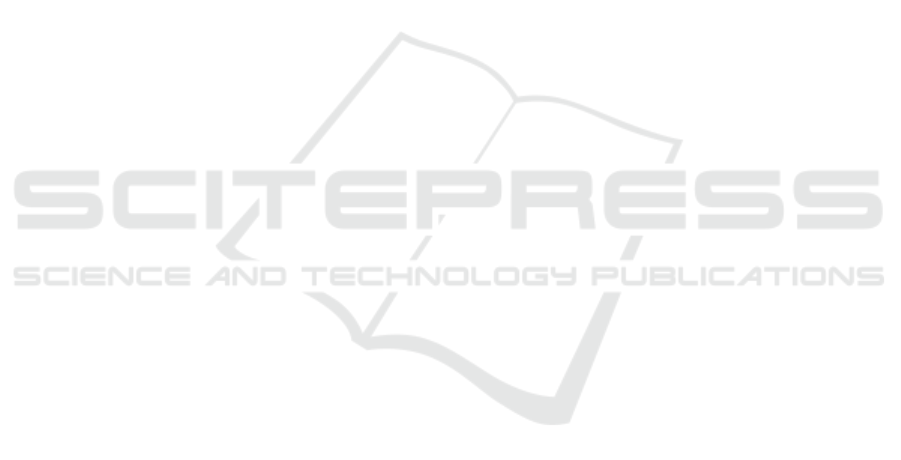
the classification of chilli leaf disease, it is hoped
that in the next study, it can classify the diseases that
attack chilli leaves. Need to do a comparison with
other CNN architectures like DenseNet, Resnet and
Alexnet to get better accuracy.
ACKNOWLEDGEMENTS
The author would like to thank all parties who have
supported the completion of this research process, as
well as those who have contributed both in the form
of time and thoughts.
REFERENCES
Anton, A., Nissa, N., Janiati, A., Cahya, N., and Astuti, P.
(2021). Application of deep learning using convolu-
tional neural network (cnn) method for women’s skin
classification. Sci. J. Informatics, 8(1):144–153,.
Das, S., Silva, C., Malemath, V., and Sundaram, K.
(2019). Disease identification in chilli leaves using
machine learning techniques. Int. J. Eng. Adv. Tech-
nol, 9(1S3):325–329,.
Gorunescu, F. (2011). Data Mining, 12.
Gulcu, A. and Kus, Z. (2020). Hyper-parameter se-
lection in convolutional neural networks using mi-
crocanonical optimization algorithm. IEEE Access,
8:52528–52540,.
Islam, A., Schreinemachers, P., and Kumar, S. (2020).
Farmers’ knowledge, perceptions and management of
chili pepper anthracnose disease in bangladesh. Crop
Prot, 133(February):105139,.
L, K., VK, K., NG, T., and D, N. (2018). Effects of cli-
mate change on growth and development of chilli.
Agrotechnology, 07(02):2–5,.
Meilin, A. and Tanaman Cabai Serta Pengendaliannya, H.
(2014). volume 16. Balai Pengkajian Teknologi Per-
tanian Jambi, Jambi.
Muslim, A. and Arnie, R. (2015). Sistem pakar diag-
nosa hama dan penyakit cabai berbasis teorema bayes.
Jutisi, 04(3):797–876,.
Nuanmeesri, S. and Sriurai, W. (2021). Multi-layer per-
ceptron neural network model development for chili
pepper disease diagnosis using filter and wrapper fea-
ture selection methods. Eng. Technol. Appl. Sci. Res,
11(5):7714–7719,.
Raziani, S. and Azimbagirad, M. (2022). Deep cnn hyper-
parameter optimization algorithms for sensor-based
human activity recognition. Neurosci. Informatics,
2(3):100078,.
Rosalina, R. and Wijaya, A. (2020). Pendeteksian penyakit
pada daun cabai dengan menggunakan metode deep
learning. J. Tek. Inform. dan Sist.Inf, 6(3):452–461,.
Rozlan, S. and Hanafi, M. (2022). Efficacy of chili plant
diseases classification using deep learning: A prelim-
inary study. Indones. J. Electr. Eng. Comput. Sci,
25(3):1442–1449,.
Saputra, R., Puspitasari, D., and Baidawi, T. (2022). De-
teksi kematangan buah melon dengan algoritma sup-
port vector machine berbasis ekstraksi fitur glcm. J.
Infortech, 2(2):2,. doi:.
Sari, Y., Baskara, A., and Wahyuni, R. (2021). Classifi-
cation of chili leaf disease using the gray level co-
occurrence matrix (glcm) and the support vector ma-
chine (svm) methods. In 2021 6th Int. Conf. Informat-
ics Comput. ICIC 2021.
Sekaran, K., Chandana, P., Krishna, N., and Kadry, S.
(2020). Deep learning convolutional neural net-
work (cnn) with gaussian mixture model for pre-
dicting pancreatic cancer. Multimed. Tools Appl,
79(15):10233–10247,.
Simalango, Y., Pane, D., and Calam, A. (2020). Sistem
pakar untuk mengidentifikasi hama dan penyakit pada
tanaman apel menggunakan metode dempster shafer.
J. CyberTech, 3(3):426,.
Tsany, A. and Dzaky, R. (2021). Deteksi penyakit tanaman
cabai menggunakan metode convolutional neural net-
work. e-Proceeding Eng, 8(2):3039–3055,.
Wahab, A. A., Zahari, R., and Lim, T. (2019). Detecting dis-
eases in chilli plants using k-means segmented support
vector machine,”2019 3rd int. Conf. Imaging, Signal
Process. Commun. ICISPC, pages 57–61,.
Zhu, Y. (2018). Gp-cnas: Convolutional neural network
architecture search with genetic programming. arXiv,.
Zikra, F., Usman, K., Patmasari, R., and Semin (2021).
Deteksi penyakit cabai berdasarkan citra daun meng-
gunakan metode gray level co-occurence matrix dan
support vector machine. Has. Penelit. dan Pengabdi.
Masy, l(N: 2598, no. E-ISSN):2598–0238, 105,.
ICAISD 2023 - International Conference on Advanced Information Scientific Development
142