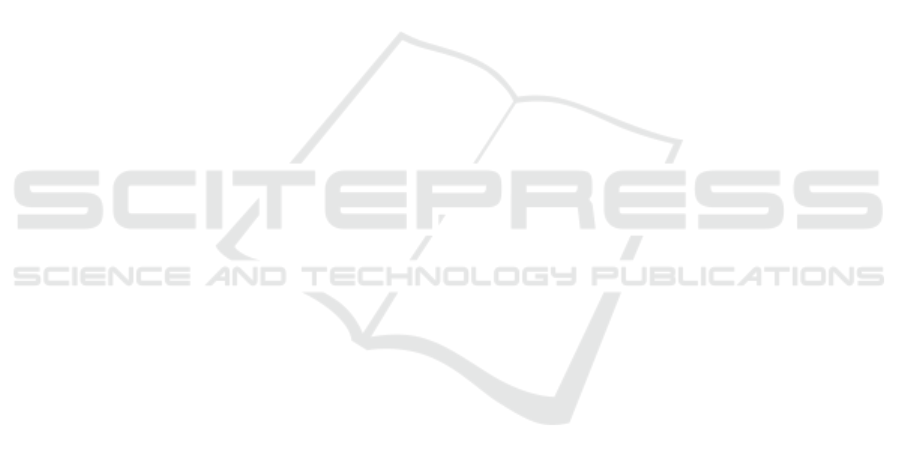
(Nikiforova, 2020). This approach contains three
components, namely data objects, data quality specifi-
cations, and data quality measurement processes. The
proposed model is applied to open data set of register
companies in several countries such as Latvia, Nor-
way, England, and Estonia. The authors claim that the
proposed method is able to overcome the weaknesses
left by the previous approach. Despite the proposed
method is quite comprehensive, it only handles data
that meets certain criteria such as: complete, free of
ambiguous values, and correct. This such constraints
present that the data quality issues continuously pro-
vide new challenges must be addressed.
Another aspect that must be considered to ensure
data quality is the proper handling of master data
(Prokhorov and Kolesnik, 2018). The proposed mas-
ter data model management system consists of three
activities: consolidation, harmonization, and man-
agement. In the consolidation stage, improvements
are applied to the data structure and data collection.
In the harmonization stage, alignment, normalization
and classification are carried out, whereas in the man-
agement action stage that must be carried out is cen-
tralization and management.
Data quality assurance is more challenging when
data collection processes are almost real time, such
as highfrequency water quality monitoring systems
as investigated by Zhang et al (Zhang and Thorburn,
2022). Many factors influence the decreasing of the
real time data quality such as network problems, de-
vice malfunction, device replacement etc. To over-
come the missing values of real time data set that
will affect the quality of the information provided,
the authors developed a cloud-based system that com-
bines several techniques and advance algorithms to
perform the missing values imputation. Several im-
putation techniques used in the system such as Mean
Imputation, LOCF, Linear Imputation, EM, MICE,
Dual-SSIM and M-RNN. Among these techniques,
by overall Dual-SSIM provides the best performance
when it applied to nitrate and water temperature data.
Despite the methods is powerful in handling the real
time data, however it does not handle integrity be-
tween data or integrity between attributes / attributes
relations.
Real world data is mostly dirty due to errors found
in data sets. In many cases, the actual data set is
inconsistent, contains missing values, lacks of in-
tegrity, ambiguous, and contains outliers. Therefore
data cleaning is not only the main task, but also the
most important part of data management since data
quality determines the quality of the information pro-
duced (Ridzuan and Zainon, 2019). The handling
of data cleansing cases requires a different approach.
Ouyang et al, as presented in (Ouyang et al., 2021),
used a ML-based ensemble approach to detect outliers
in a concrete measurement regression data set. The
technique used in this case is the ANN-based model
compared to KNN, LOF, COF, OCSVM, IFOREST,
ABOD, and SOS. The approach used in the study is
the best algorithm selection approach with forward
and backward techniques. Based on the experimental
results, ANN gives the best results in detecting out-
liers in the regression data used.
Noisy data that contributes to unreasonable
decision-making also occurs in the energy manufac-
turing industry such as the offshore wind turbine
structures health data collected through the SCADA
monitoring system. To overcome the problem,
(Martinez-Luengo et al., 2019) proposes a method
based on ANN techniques to improve data quality
through automation of data cleaning processes. The
proposed framework consists of two steps: data noise
checking and removal, and missing data imputation.
This research was conducted to improve the quality
of fatigue assessment on turbines which are thought
to be heavily influenced by the quality of monitor-
ing data generated by SCADA sensors. Therefore, in
his research the authors compared the quality of data
without cleaning with the quality of data with clean-
ing using the proposed method. From the experimen-
tal results, the authors concluded that the quality of
the data after cleaning proved to be better.
In their review of big data cleansing, (Ridzuan and
Zainon, 2019) summarize some methods can be ap-
plied to the purposes. Those methods are developed
based on various techniques such as rule-based, ML-
based, and knowledge-based. However, those existing
methods contain some limitations when it deals with
dirty data.
The complete data collection of Cooperations and
SMEs conducted by the Ministry of SMEs is a unique
data collection model. The uniqueness, complexity,
and problems are found in all components, including
area coverage, individual data targets, data collection
model which is performed manually, various skill and
knowledge of data collection officers, the complex-
ity of data entry forms, short time allocated, and the
project management as well. In terms of area cover-
age, the project covers more 240 district, 34 provinces
crossover Indonesia Country with various topogra-
phies and land contours. The data collection is car-
ried out manually by more than 1.000 enumerators.
As other models of real data collection, data qual-
ity is also a major issue that must be resolved before
further use of the data. Due to this uniqueness and
exclusiveness, to the author’s knowledge, there is no
model/approach that can deal with this data quality
Comprehensive Approach to Assure the Quality of MSME Data in Indonesia: A Framework Proposal
155