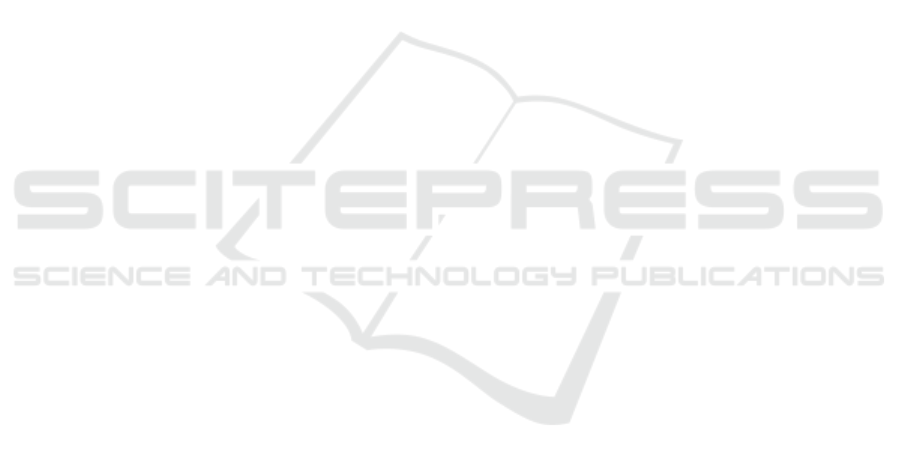
2 METHOD
Priority estimation from the pairwise comparison ma-
trix is a major part of AHP. Through the pairwise
comparison matrix, the priority level of each element
will be determined. By developing priority vectors
for all matrices in the hierarchies formed for a par-
ticular decision problem, it is possible to perform
aggregation and obtain the final priority vector (to-
tal priority). There are different techniques for de-
termining priority vectors from comparison matrices
and much research effort has been directed towards
finding the best estimation method. The eigenvector
(EV) method, which was proposed for the first time
by (Saaty, 1977), proved that the eigenvector principle
of the comparison matrix can be used as the required
priority vector, for consistent or non-consistent judg-
ments from decision makers. The standard procedure
for determining priority vectors with the EV method
is based on a square comparison matrix and normaliz-
ing the number of rows. Saaty also proposed several
simple approximation methods to obtain the required
vectors.
The simplest method is the addition normalization
method or the additive normalization (AN) method.
This method generates priority by taking the sum of
the columns in the comparison matrix and by averag-
ing the values obtained in the rows. Although AN
is not widely accepted in the scientific community
which prefers more sophisticated methods, it is still
widely used because of its simplicity. The results of
the analysis show that this method is competitive with
other methods.
An interesting modification of the EV method pro-
posed by (Cogger and Yu, 1985), is based on the
premise that the overall preference intensity informa-
tion is contained in the upper triangular matrix of
the comparison matrix. The calculation procedure is
recursive and simple, but the study by (Golany and
Kress, 1993), shows that this method is not effective
and can be excluded.
Used the EV method to investigate prioritization
with the addition of alternatives to the Analytic Hi-
erarchy Process. With the addition of alternatives
to the Analytic Hierarchy Process for certain cases,
the priority order of the previous alternatives can be
changed. But by modifying the way to normalize
the Eigen Vector (EV) it produces a procedure that is
able to maintain the order of priority (Schmidt et al.,
2015).
Most of the other methods of obtaining priority
from a comparison matrix are considered extreme be-
cause they are based on an optimization approach.
The prioritization problem is expressed as minimiz-
ing a certain objective function that measures the de-
viation between the ideal solution and the actual solu-
tion, subject to some additional constraints. As stated
by (Mikhailov and Singh, 1999), priority assessment
can be formulated as a non-linear optimization prob-
lem with constraints and solved by the direct least-
squares (DLS) method (Chu et al., 1979), stated that
although this method minimizes the Euclidean dis-
tance between the ideal solution and the actual solu-
tion, this method generally results in multiple solu-
tions which can be considered as a drawback from a
practical point of view. To eliminate it, several opti-
mization methods such as the weighted least-squares
method or the weighted least-squares (WLS) method
are proposed, using a modified Euclidean norm as the
objective function.
(Crawford and Williams, 1985) suggested the log-
arithmic least squares (LLS) method provides an ex-
plicit solution through an optimization procedure that
minimizes the logarithm of the objective function
by fulfilling the multiplication constraints. (Cook
and Kress, 1988), put forward the logarithmic least
absolute values (LLAV) method, a median-relation
method that is not biased toward determining extreme
values. (Wang et al., 2007), put forward an estimation
of priority in AHP through a correlation coefficient,
known as the Correlation Coefficient Maximization
Approach (CCMA). CCMA is able to maximize the
correlation coefficient between its own priorities and
each column of the pairwise comparison matrix. Of
the various methods in determining priorities, the au-
thors put forward a model that will be discussed in
setting priorities, namely through the correlation co-
efficient or the Correlation Coefficient Maximization
Approach (CCMA).
3 RESULTS AND DISCUSSION
Correlation Coefficient Maximization Approach
(CCMA) as an approach to maximizing the correla-
tion coefficient used in determining the priority of the
Pairwise Comparison Judgment Matrices (PCJM).
According to (Wang et al., 2007), prioritization by
maximizing the correlation coefficient can maximize
the correlation coefficient between priorities and
each column of the pairwise comparison matrix.
Suppose A = (a
i j
)
nxn
is a pairwise comparison matrix
a
i j
= 1/a
i j
,a
ii
= 1 and a
i j
> 0 for i, j = 1,..,n
and W = (w
1
,...,w
n
)
T
as a priority vector with
∑
n
i=1
w
i
= 1 and w
i
≥ 0, for i = 1, ..., n . According
to (Saaty, 1988), if a
i j
= a
ik
a
k j
for k = 1, ..., n then
A = (a
i j
)
nxn
it is called a perfectly consistent pairwise
comparison matrix. For a perfectly consistent com-
A Model to Determine Priority in AHP Using Coefficient Correlation
241