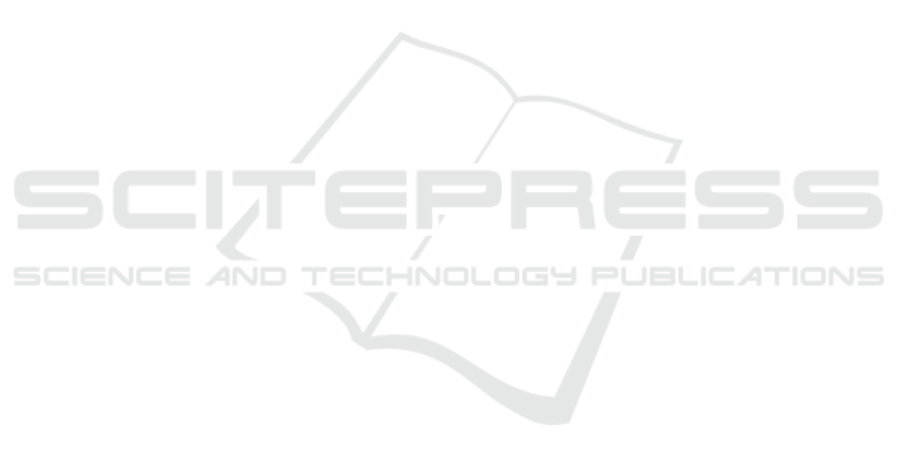
3.4.1 Comparison of Simulation Results of
Hebbian Algorithm Testing
After conducting a simulation test using the Hebbian
algorithm, the best accuracy value is Hebbian learn-
ing with binary input patterns, both with binary output
and with bipolar output, which produces an accuracy
value of 65
4 CONCLUSIONS
The conclusion of this study is that the Hebbian al-
gorithm can predict lung cancer in smokers with an
accuracy of 65% with binary input patterns and bi-
nary and bipolar output patterns. To produce better
test results for accuracy, it is necessary to study with
other algorithms.
REFERENCES
Bisri, H., Bustomi, M., and Purwanti, E. (2013). Image
classification of the lungs by histogram feature extrac-
tion and backpropagation neural networks. J. Sains
Dan Seni Pomits, 2(2):68–71,.
Gillett, M., Pereira, U., and Brunel, N. (2020). Characteris-
tics of sequential activity in networks with temporally
asymmetric hebbian learning. Proc. Natl. Acad. Sci.
U. S. A, 117(47):29948–29958,.
Golkar, S., Lipshutz, D., Bahroun, Y., Sengupta, A.,
and Chklovskii, D. (2020-12). A simple norma-
tive network approximates local non-hebbian learn-
ing in the cortex. Adv. Neural Inf. Process. Syst,
(NeurIPS):1–13,.
Illing, B., Ventura, J., Bellec, G., and Gerstner,
W. (2021). Local plasticity rules can learn
deep representations using self-supervised contrastive
predictions. Adv. Neural Inf. Process. Syst,
36(NeurIPS):30365–30379,.
Isomura, T. and Toyoizumi, T. (2019). Multi-context blind
source separation by error-gated hebbian rule. Sci.
Rep, 9(1):1–13,.
Journ
´
e, A., Rodriguez, H., Guo, Q., and Moraitis, T. (2022).
Hebbian deep learning without feedback. Available:.
Kwessi, E. (2022). Strong allee effect synaptic plasticity
rule in an unsupervised learning environment. Avail-
able:.
Liu, F., Sekh, A., Quek, C., Ng, G., and Prasad, D.
(2021). Rs-herr: a rough set-based hebbian rule re-
duction neuro-fuzzy system. Neural Comput. Appl,
33(4):1123–1137,.
Magotra, A. and Kim, J. (2020). Improvement of hetero-
geneous transfer learning efficiency by using hebbian
learning principle. Appl. Sci, 10(16).
Muscoloni, A. and Cannistraci, C. (2021). Short note
on comparing stacking modelling versus cannistraci-
hebb adaptive network automata for link prediction in
complex networks. Preprints, (May).
Najarro, E. and Risi, S. (2020-12). Meta-learning through
hebbian plasticity in random networks. Adv. Neural
Inf. Process. Syst.
Napitupulu, S. and Situmorang, Z. (2020). Optimization of
giving employee craft assessment using artificial neu-
ral network with hebb algorithm,”iop conf. Ser. Mater.
Sci. Eng, 725(1).
Napole, C., Barambones, O., Calvo, I., and Velasco, J.
(2020). Feedforward compensation analysis of piezo-
electric actuators using artificial neural networks with
conventional pid controller and single-neuron pid
based on hebb learning rules. Energies, 13(15):1–16,.
Nicola, W. and Clopath, C. (2019). A diversity of in-
terneurons and hebbian plasticity facilitate rapid com-
pressible learning in the hippocampus. Nat. Neurosci,
22(7):1168–1181,.
Osakabe, Y., Sato, S., Akima, H., Kinjo, M., and Sakuraba,
M. (2021). Learning rule for a quantum neural net-
work inspired by hebbian learning. IEICE Trans. Inf.
Syst, D(2):237–245,.
Pedersen, J. and Risi, S. (2021). Evolving and merging heb-
bian learning rules: Increasing generalization by de-
creasing the number of rules, volume 1. Association
for Computing Machinery.
Pogodin, R. and Latham, P. (2020-12). Kernelized informa-
tion bottleneck leads to biologically plausible 3-factor
hebbian learning in deep networks. Adv. Neural Inf.
Process. Syst.
Prasetio, R. and Susanti, S. (2019). Prediction of life ex-
pectancy in lung cancer patients after thoracic surgery
using boosted k-nearest neighbor. J. Responsif,
1(1):64–69,. Available:.
Qin, Y. and Duan, H. (2020). Single-neuron adaptive hys-
teresis compensation of piezoelectric actuator based
on hebb learning rules,”micromachines.
Setyadi, Y., Asror, I., and Wibowo, Y. (2020). Prediction of
life expectancy after thoracic surgery in patients with
lung cancer using genetic algorithm methods for fea-
ture selection and na
¨
ıve bayes classifier. e-Proceeding
Eng, 7(20):8349–8360,.
Solikhun, M., Safii, M., and Zarlis, M. (2020a). Back-
propagation network optimization using one step se-
cant (oss) algorithm. IOP Conf. Ser. Mater. Sci. Eng,
769(1).
Solikhun, M., Safii, M., and Zarlis, M. (2020b). Resilient
algorithm in predicting fertilizer imports by major
countries. IOP Conf. Ser. Mater. Sci. Eng, 769(1).
Tsai, M. (2021). Photoactive electro-controlled visual
perception memory for emulating synaptic metaplas-
ticity and hebbian learning. Adv. Funct. Mater,
31(40):13–14,.
ICAISD 2023 - International Conference on Advanced Information Scientific Development
280