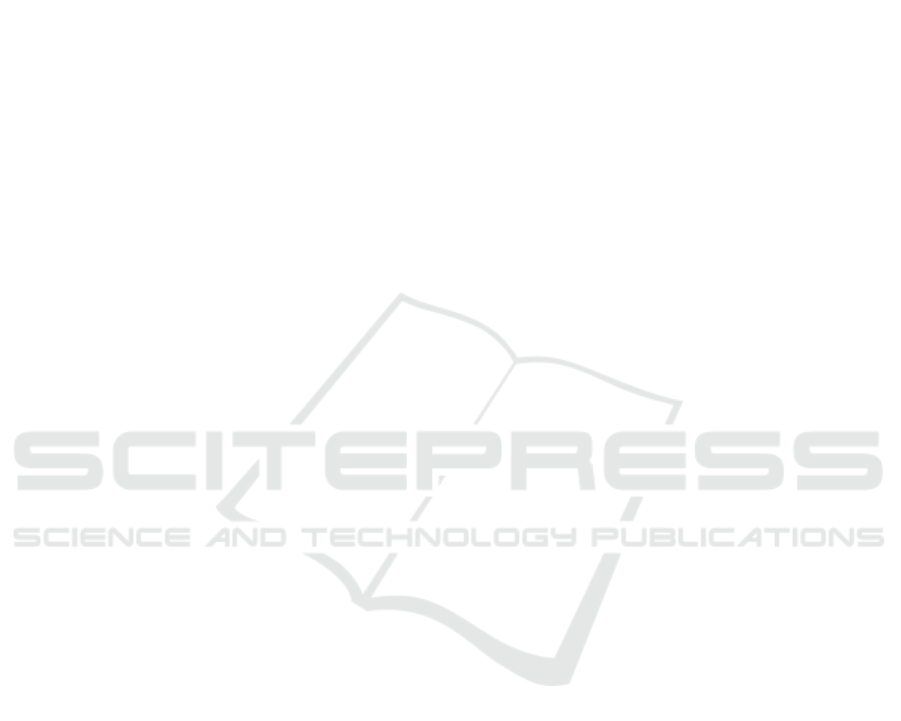
Graph Convolutional Networks with Knowledge Graph for Myers-Briggs
Type Indicator
Heru Mardiansyah, Saib Suwilo, Erna Budhiarti Nababan and Syahril Efendi
Universitas Sumatera Utara, Medan, Indonesia
Keywords:
Knowledge Graph, Graph Convolutional Networks, Myers-Briggs Type Indicator.
Abstract:
The order of vertices in a graph is very important because the graph is oriented. Or the vertices are not im-
portant because they are not oriented. The graph of data is a heterogeneous polydigraph, called a directed
vertex set, with many edges between any two vertices. Information is created by establishing real-world rela-
tionships between graphics and objects. This study was conducted to improve machine learning performance
by proposing a theoretical model for the data used and creating a graph convolutional network (GCN) model
for training the data. Data are created from a low-dimensional (latent) space, but can only be observed in
a high-dimensional (observation) space. The results of these studies may not always yield the same results
because they were not measured on the same person, were unreliable, or the results obtained did not provide
consistent results. MBTI tests may change at any time. It is obtained according to the result of a person’s
mood. This MBTI method is often considered weak and unscientific, so it must be tested with 200 iterations
on the GCN. The resulting GCN scores are 89.8% accuracy and 2.78 Test Loss.
1 INTRODUCTION
MBTI (Myers-Briggs Type Indicator) gives a simple
description in a manner psychometric about type per-
sonality. Although characterization is short This is
Possibly useful in some context apply (in predicting
style behavior characteristics individual, intellectual,
and interpersonal), exists limitations to the psycho-
metric clear instrument (Albrecht et al., ). The MBTI
is a system-type personality that divides people into
16 types of different personalities of 4 parts: Introver-
sion (I) - Extroversion (E), Intuition (N) - Feeling (S),
Thinking (T) - Feeling (F), Judging (J) - Perceiving
(P), (You can read more carry on about What mean-
ing here).
For example, someone with more introversion, in-
tuition, thinking, and perception is called an INTP
inside the MBTI system, and there are Lots of
component-based modeling personality or describe
preference or the person’s behavior based on appoint-
ment (Altuner and Kilimci, ).
This is one tests the most popular personality in
the world. This is used For business, online, fun, re-
search, and more. A simple Google search will dis-
close all method different that has been used to test
from time to time. Can be said that testing This Still
very up to date its use worldwide. From the corner
view scientific or psychological based on Carl Jung’s
work on function cognitive, that is Jungian typology.
This is a model of eight functions, thought processes,
or methods to think differently that has been sug-
gested for is inside the mind. Work This has changed
become some system with different personalities for
facilitating it, the most popular Of course just is the
MBTI (Hogan et al., ). recently, its usage/validity
questioned because, among other things, it doesn’t
can dependable in experiments around. However, it
has still become a very tool useful in many fields, and
the goals of this data set are to determine if there is a
possible pattern recognized in type and style writing
certain and, more general, for test validity test mo-
ment analysis, predict, or classify behavior
2 METHODOLOGY
The vertices in a graph is very important so that the
graph is directed, or the vertices of the graph are not
important, so they are not directed. The knowledge
graph is a heterogeneous multidigraph which is called
a sequence of directed vertices and has many edges
between the two nodes. A knowledge is created by
making a real relationship between graphics and ob-
jects. The knowledge graph (KG), also known as
Mardiansyah, H., Suwilo, S., Nababan, E. and Efendi, S.
Graph Convolutional Networks with Knowledge Graph for Myers-Briggs Type Indicator.
DOI: 10.5220/0012448500003848
Paper published under CC license (CC BY-NC-ND 4.0)
In Proceedings of the 3rd International Conference on Advanced Information Scientific Development (ICAISD 2023), pages 285-288
ISBN: 978-989-758-678-1
Proceedings Copyright © 2024 by SCITEPRESS – Science and Technology Publications, Lda.
285