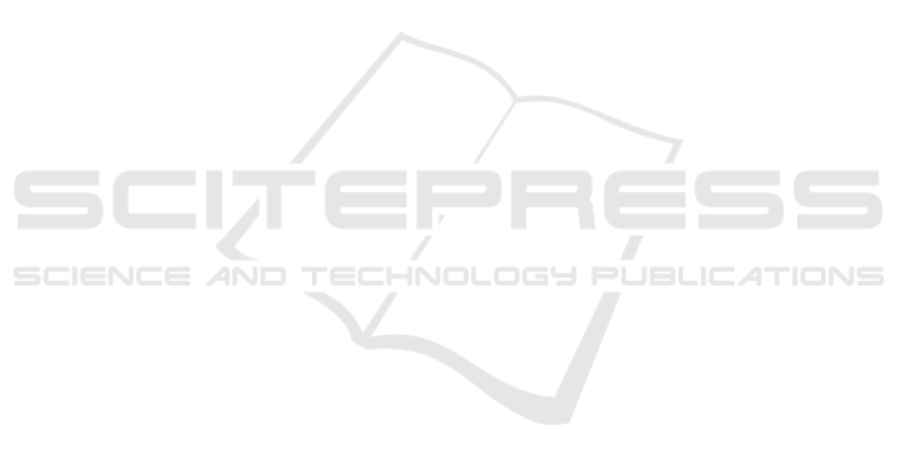
Based on test data on 20 people, it was success-
fully classified into 3 clusters, namely students in
class 1 with a trend of X values being in the do not
know the value, Y with a very ignorant value while
the Y value is in a value between not knowing and par-
tially understanding with the total 9 people. Students
in class 2 with the trend that the value of X is in the
very ignorant value, Y with a partial understanding
value while the Y value is in the partial understanding
value with a total of 5 people. Students are in class
3 with a trend with a value of X being between not
knowing and partially understanding, Y with a partial
understanding value while the Y value is at a very ig-
norant value with a total of 6 people with an accuracy
level of the f1 test score of 100%.
REFERENCES
Alani, M. and Tawfik, H. (2022). Phishnot: A cloud-based
machine-learning approach to phishing url detection.
Comput. Networks, 218:109407,. doi:.
Asish, S., Kulshreshth, A., and Borst, C. (2022). Detect-
ing distracted students in educational vr environments
using machine learning on eye gaze data. Comput.
Graph, 109:75–87,. doi:.
Baceviciute, S., Mottelson, A., Terkildsen, T., and Makran-
sky, G. (2020). Investigating representation of text and
audio in educational vr using learning outcomes and
eeg. In Proceedings of the 2020 CHI Conference on
Human Factors in Computing Systems, pages 1–13,.
Butcher, K. (2017). The multimedia principle. In The
Cambridge handbook of multimedia learning, page
174–205. Cambridge University Press, New York,
NY, US, 2nd edition.
Fu, T. (2011). Engineering applications of artificial intelli-
gence a review on time series data mining. Eng. Appl.
Artif. Intell, 24(1):164–181,.
Geyer, S., Papaioannou, I., and Straub, D. (2019). Cross
entropy-based importance sampling using gaussian
densities revisited. Struct. Saf, 76:15–27,. doi:.
Han, Y., Miao, Y., Lu, J., Guo, M., and Xiao, Y. (2022). Ex-
ploring intervention strategies for distracted students
in vr classrooms.
Jiang, G., Wang, W., and Zhang, W. (2019). A novel dis-
tance measure for time series: Maximum shifting cor-
relation distance. Pattern Recognit. Lett, 117:58–65,.
doi:.
Jung, I. (2011). The dimensions of e-learning quality: From
the learner’s perspective. Educ. Technol. Res. Dev,
59(4):445–464,.
Keogh, E. and Kasetty, S. On the need for time series data
mining benchmarks : A survey and empirical demon-
stration.
Kuklin, V., Alexandrov, I., Polezhaev, D., and Tatarkanov,
A. (2023). Prospects for developing digital
telecommunication complexes for storing and ana-
lyzing media data. Bull. Electr. Eng. Informatics,
12(3):1536–1549,.
Kumar, Y., Kaul, S., and Hu, Y.-C. (2022). Machine learn-
ing for energy-resource allocation, workflow schedul-
ing and live migration in cloud computing: State-
of-the-art survey. Sustain. Comput. Informatics Syst,
36:100780,. doi:.
Lauwers, O. and Moor, B. (2017). A time series distance
measure for efficient clustering of input / output sig-
nals by their underlying dynamics.
Liu, D., Fox, K., Weber, G., and Miller, T. (2022). Confed-
erated learning in healthcare: Training machine learn-
ing models using disconnected data separated by in-
dividual, data type and identity for large-scale health
system intelligence. J. Biomed. Inform, 134:104151,.
doi:.
Marelli, S. and Sudret, B. (2018). An active-learning algo-
rithm that combines sparse polynomial chaos expan-
sions and bootstrap for structural reliability analysis.
Struct. Saf, 75:67–74,. doi:.
Moore, D. (2016). E-learning and the science of instruc-
tion: Proven guidelines for consumers and design-
ers of multimedia learning. Educ. Technol. Res. Dev,
54(2).
Nordin, N., Zainol, Z., Noor, M., and Chan, L. (2022).
Suicidal behaviour prediction models using machine
learning techniques: A systematic review. Artif. In-
tell. Med, 132:102395,. doi:.
Rasheed, F. and Wahid, A. (2021). Learning style detection
in e-learning systems using machine learning tech-
niques. Expert Syst. Appl, 174(February).
Ree, J., Muralidharan, K., Pradhan, M., and Rogers, H.
(2018). Double for nothing? experimental evidence
on an unconditional teacher salary increase in indone-
sia. Q. J. Econ, 133(2).
Serra, J. and Arcos, J. An empirical evaluation of similarity
measures for time series classification.
Sukanya, J., Gandhi, K., and Palanisamy, V. (2022). An
assessment of machine learning algorithms for health-
care analysis based on improved mapreduce. Adv.
Eng. Softw, 173:103285,. doi:.
Umatani, R., Imai, T., Kawamoto, K., and Kunimasa, S.
(2023). Time series clustering with an em algorithm
for mixtures of linear gaussian state space models.
Pattern Recognit, pages 109375,. doi:.
Victor, A. and Ghalib, M. (2017). Automatic detection and
classification of skin cancer. Int. J. Intell. Eng. Syst,
10(3):444–451,.
Wickramasinghe, I. (2022). Applications of machine learn-
ing in cricket: A systematic review. Mach. Learn. with
Appl, 10:100435,. doi:.
Clustering Diagnostic Assessment of Students with the K-Means Algorithm Based on Talents and Interests
301