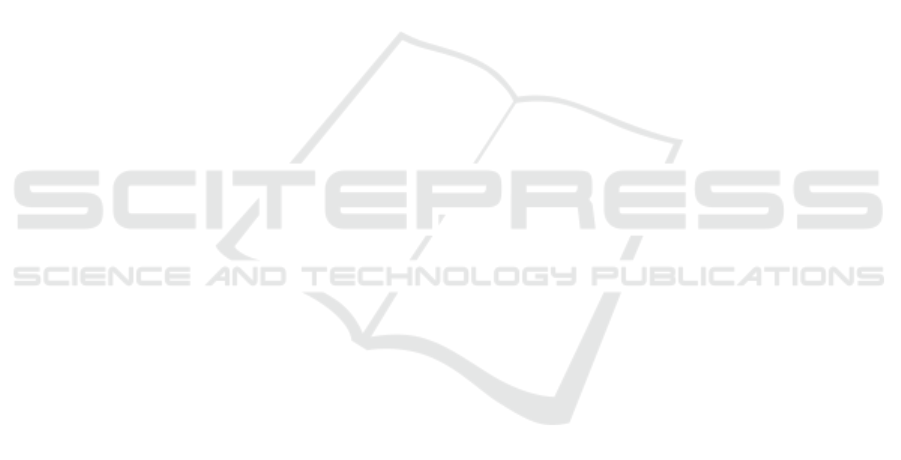
each order consists of multiple items to be fulfilled)
for an urban warehouse.
Multi-product inventory systems with substitution
can be traced back to the 1970s. Pioneering works in-
clude Mcgillivray and Silver (1978) and Parlar and
Goyal (1984). They analyzed the structure of the
problem and developed several heuristics. Later in
Netessine and Rudi (2003), it was shown that the ex-
pected profit for a simple two-product problem may
not be quasiconcave. Our work belongs to the stream
of research on customer-based substitution with rank-
based.
A choice model, where each customer tries to find
the available products following their own ranks. This
ranking can also be determined by a utility maxi-
mization criterion. Related works include but are not
limited to Smith and Agrawal (2000), Mahajan and
van Ryzin (2001), Honhon et al.(2010), Honhon et
al. (2012), Honhon and Seshadri (2013), Goyal et al.
(2016). We refer the reader to K”ok et al. (2015)
and Chen Gong (2018) for a more comprehensive lit-
erature review. It can be noticed that the existing lit-
erature mainly focuses on analyzing structural prop-
erties, and proposing efficient solution methods for
optimization problems. To the best of our knowl-
edge, Chen and Chao (2019) is the only paper that
studies joint learning and inventory control optimiza-
tion problems with stock-out substitution. In Chen
and Chao (2019), they consider one-time or Markov
chain-based stock-out substitution, where the sub-
stitution behavior is determined by the substitution
probability between each pair of product and demand.
Furthermore, their algorithm has separate predefined
learning and earning cycles, and in the learning cy-
cle the algorithm allocates a specific period to learn
the substitution probabilities. This model is applica-
ble with rank-based choice models without specify-
ing substitution probabilities. Algorithms that do not
have separate predefined learning and earning cycles,
and can achieve much more efficient numerical per-
formance (e.g., achieving an average of 15% regret in
about 50 periods).
The multi-product inventory assortment problem
with multi-product orders faced by urban warehouses
is a more recent topic. We call it the inventory se-
lection problem for short runs. The model is moti-
vated by the inventory challenges faced by emerging
growth and fierce competition ecommerce companies.
To enable very fast delivery, companies use expen-
sive urban warehouses in high-traffic cities. Accord-
ing to eMarketer (Hartomo and Winarko, 2015), in
the United States, the total order value of same-day
delivery merchandise has reached 4.03 billion dol-
lars in 2018, while this number was 0.04 billion in
2013. Meanwhile, according to DeValve et al. (2018),
one of the largest e-retailers in China also adopted a
two-tier distribution system consisting of regional dis-
tribution centers and urban warehouses. Due to the
importance of this topic, researchers began to study
the challenges faced by urban warehouses. Jin et
al. (2018) considered the product selection problem
faced by urban warehouses. DeValve et al. (2018)
studied the value of flexibility for the fulfillment of
problems that occur in the daily operations of urban
warehouses.
Assortment optimization was brought to the atten-
tion of the revenue management community by van
Ryzin and Mahajan (2009). Since then assortment
optimization techniques and models have been widely
researched, with much of the previous work well sum-
marized. The multinomial logit (MNL) model, our fo-
cus in this paper, is among the most commonly stud-
ied customer choice models for assortment optimiza-
tion (Talluri and van Ryzin, 2004, Du et al., (2016),
and Rusmevichientong et al., (2010) are a few exam-
ples).
Train [20091] provides a good practical and the-
oretical summary of logit models in the context of
choice modeling. A common assumption made in the
literature on MNL models is that the utility of each
product is linear in the product attributes. See Vol-
cano et al. (2018) and Rusmevichientong et al. (2010)
for a discussion of this assumption. However, com-
bining different customer attributes is a more recent
trend that we discuss later.
In the assortment optimization literature, two
trends are particularly relevant to our work: model
estimation and personalization. Recently, researchers
have investigated the problem of estimating the model
of choice for multivariate optimization.
2.3 Perishable Product
Perishable products are products that can no longer be
used after a certain period of time. The period of use
of perishable products is only as long as the quality of
the product is still good and depends on the survival
time of the product. If the survival time has been ex-
ceeded, the product can no longer be used and must be
disposed of, examples of products that are often en-
countered are food, blood, and medicine (Prastacos,
1981), thus the product will experience a decrease in
quality along with a reduction in its life time which
results in a decrease in the value of the product (Hi-
dayah, 2015a).
Generally, perishable products that are sold have
a lifespan of no more than 14 days, this is usu-
ally caused by microbial damage and the occurrence
Enhanced of Analysed Optimization Learning Model for Multi Product Retail and Distribution System
309