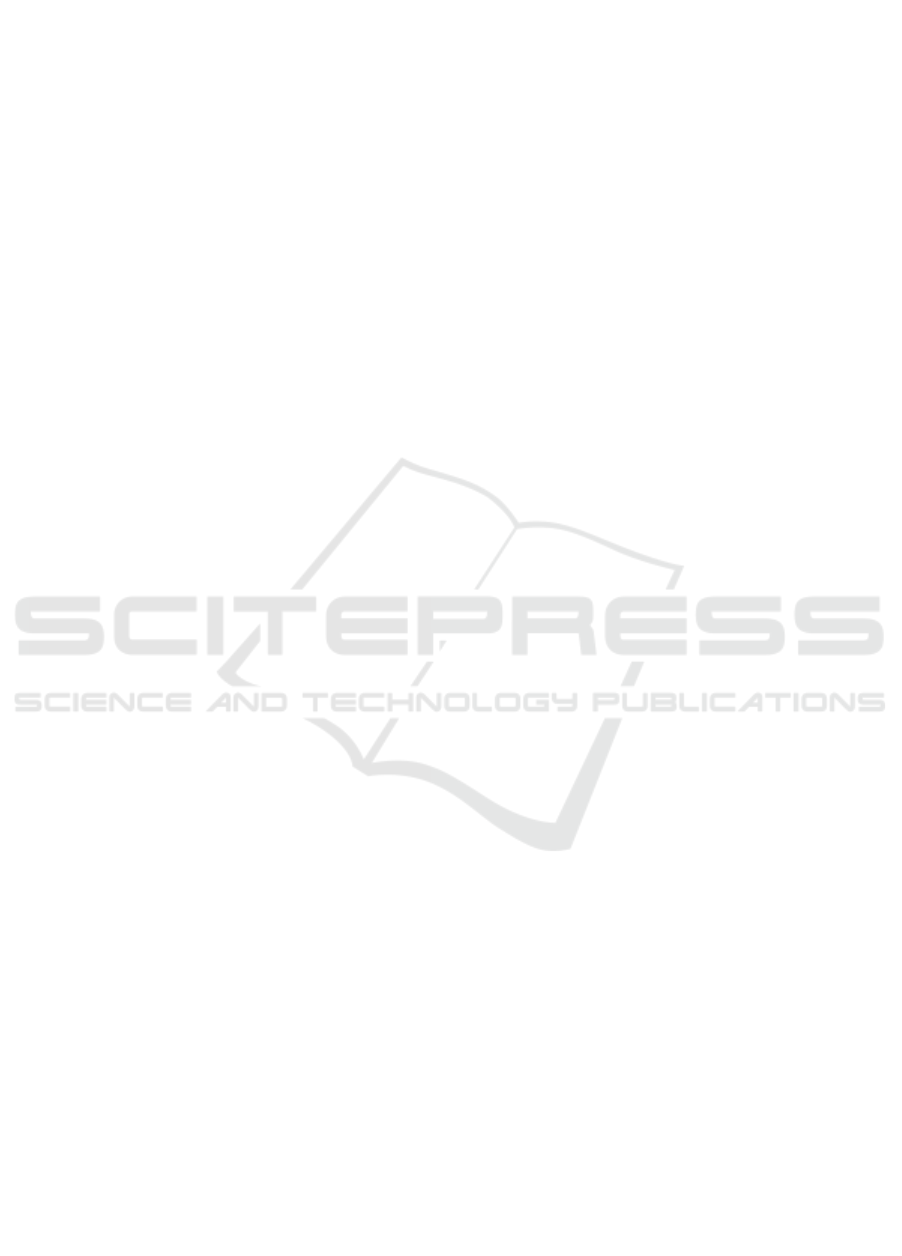
6 CONCLUSION
In the ongoing quest for developing optimal machine
learning algorithms for predicting used car prices, the
Novel XGBoost Regressor has emerged as a notably
effective solution. When compared against the
AdaBoost Regressor, the Novel XGBoost Regressor
outperforms with a commendable accuracy rate of
87.74%. In contrast, the AdaBoost Regressor lags
slightly behind with an accuracy of 84.31%.
Several aspects from the study underline this
superiority:
• Statistical Significance: A rigorous T-test
analysis for independent samples was
conducted, leading to a p-value of 0.000
(p<0.05). This result alone provides a
compelling argument for the statistical
superiority of the Novel XGBoost Regressor
over the AdaBoost Regressor. The low p-
value highlights that the difference in their
performances is significant and not just a
random occurrence.
• Supporting Literature: Multiple research
endeavors in the domain corroborate the
findings. For instance, a study by K.
Samruddhi and R. A. Kumar in 2020 reached
a model accuracy close to 85%. Another
investigation using the Random Forest
algorithm, as suggested by Pal et al. (2019),
secured an accuracy of 83.63%. These
findings align with the current study's
observations that advanced machine learning
techniques, like the Novel XGBoost
Regressor, tend to outdo traditional methods
in predicting used car prices.
• Mean Accuracy and Standard Deviation: The
analysis further delineated the Standard
Deviation and Mean Accuracy of both models.
While the AdaBoost Regressor recorded a
mean accuracy of 84.31% with a standard
deviation of 1.64992, the Novel XGBoost
Regressor surpassed it with a mean accuracy
of 87.74% and a slightly lower standard
deviation of 1.33167. This not only highlights
the higher accuracy of the Novel XGBoost but
also suggests its consistent performance
across different datasets.
• Limitations & Future Scope: Despite its
impressive accuracy, the study did confront
challenges linked to recent economic
phenomena, such as the semiconductor
shortage triggered by the pandemic, which
affected used car prices. The dataset's
potential inability to represent real-time
market prices underscores the necessity for
continuous algorithm updating and training.
In conclusion, while both models hold merit in
their respective capacities, the Novel XGBoost
Regressor exhibits a clear edge in the context of
predicting used car prices. Its amalgamation of speed,
efficiency, and accuracy makes it a potent tool for
researchers and industry professionals, offering a
blueprint for future advancements in the realm of
machine learning-powered price predictions.
REFERENCES
Santosh Kumar Satapathy, Rutvikraj Vala, and Shiv
System Using Effective Machine Learning
https://doi.org/10.1109/CISES54857.2022.9844350.
Asghar, Muhammad, Khalid Mehmood, Samina Yasin, and
Prediction Using Machine Learning with Optimal
Pakistan Journal of Engineering and
Technology 4 (2): 11319.
Bharambe, Prof Pallavi, Bhargav Bagul, Shreyas Dandekar,
International Journal for Research in Applied Science
and Engineering Technology 10 (4): 77378.
Cui, Baoyang, Zhonglin Ye, Haixing Zhao, Zhuome
Price Prediction Based on the Iterative Framework of
Electronics 11 (18): 2932.
Gültek
Secondhand Cars Sold on the Internet with Artificial
Journal of Internet
Applications and Management 11 (1): 4961.
Janke, Varshitha, Jahnavi K, and Lakshmi C. 2022.
2022
International Conference on Computer Communication
and Informatics (ICCCI). IEEE.
https://doi.org/10.1109/iccci54379.2022.9740817.
Liu, Enci, Jie Li, Anni Zheng, Haoran Liu, and Tao Jiang.
Car Price in View of the PSO-GRA-BP Neural
Sustainability: Science Practice and Policy
14 (15): 8993.
Kishore Kumar, M. Aeri, A. Grover, J. Agarwal, P. Kumar,
10.1109/icesc51422.2021.9532845.
Palanivelu, J., Thanigaivel, S., Vickram, S., Dey, N.,
Mihaylova, D., & Desseva, I. (2022). Probiotics in
functional foods: survival assessment and approaches
for improved viability. Applied Sciences, 12(1), 455.
Pal, Nabarun, Priya Arora, Puneet Kohli, Dhanasekar
Sundararaman, and Sai Sumanth Palakurthy. 2019.
A Comparative Analysis of XGBoost Model and AdaBoost Regressor for Prediction of Used Car Price
445