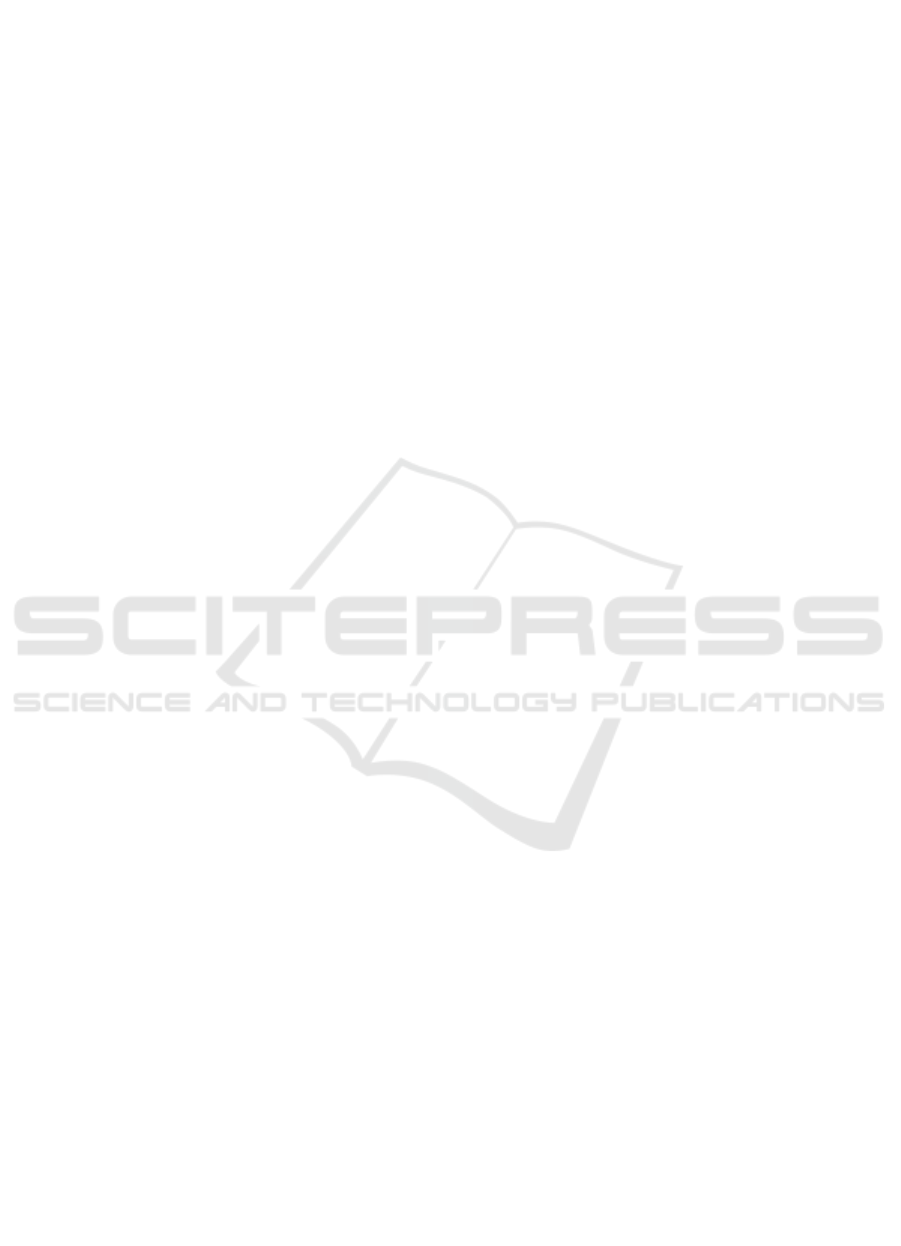
They developed RA flare estimators in ML. Machine
learning tools are built from modern clinical data.
Risk estimation of the model using 10 clinical
variables DAS28-ESR; the duration of the disease;
the mode of administration of the biological
DMARD; against the CCP; gender; HAQ (Health
Assessment Questionnaire); To count. The
prevalence of seizures in patients at high risk of
seizures is 23%. To be binary, <23% must be marked
"no glow" and >=23 must be marked "glare". The
sensitivity of the first RA disease predictor was 72%,
specificity 76%, positive predictive value 37%,
negative predictive value 93%, and AUROC 80%.
The overall accuracy of the hazard assessment
tool is 75%. Small sample sizes, lack of comparative
studies, exclusion of patients, bias, reliability issues,
and uncertainty are limitations of previous studies.
Disagreement between doctors and forecasting tools
can also lead to more emotion for patients. This will
be beneficial for patient care.
2.2 Explainable AI for Decision
Support System
This article discusses the use of AI in CDSS (Clinical
Decision Support Systems). Explain the advantages
and disadvantages of using AI in CDSS. Their
analysis focuses on three levels: emotional
intelligence, human factors, and the role of role in
decision-making. They present, with further
interpretation, two cases of social-technical relations.
In the case of the definition described above, they use
the heart attack predictor. The tool is a black box
(ANN-artificial network node) Research studies
show better understanding and shorter research times.
In terms of decision-making, it should be ensured that
the system performance is not limited to certain
situations, but can be generalized to many situations,
in order to achieve the best performance and achieve
the best performance. In general, technical
excellence, robust validation, and generalizability are
required when translation is neglected in medical
systems. In the next layer of human factors, users
must believe that there is also a process in the audit
verification process of the system.
The recommended way to solve this is to involve
the client in the design and analysis. The design phase
of research should allow for user feedback and
criticism. which should be done. This increases their
confidence in the system. Level 3 Responsibilities
established in decision making, critical conditions for
emergency dispatch, miscalculation can be fatal.
Therefore, the lack of explanation significantly
changes the performance of the system, because the
decision-making process must support the algorithm-
driven system.
In summary, CDSS depends on many factors.
These factors include efficiency, the effectiveness of
descriptive algorithms, specific content features,
assigned roles, and user groups for decision-making.
According to the authors, a clear answer to the role of
translation cannot be determined at the theoretical
level. Context specific consideration of interpretation
is required in CDSS and supports collaboration to
address the ethical and practical implications of AI in
healthcare (Amann, 2022).
2.3 AI in Depression Treatment on
Doctor-Patient Communication
CDSS in the context of depression treatment. CDSS
is designed to support the hospital by taking into
account many patient changes. It emphasizes the trial-
and-error process, which is often used in treatment
selection, and aims to reduce the number of failed
treatments. The tool is designed for use in patient-
centered discussions and facilitates collaborative
decision-making between doctors and patients. Their
aim is to connect patients with their healthcare
providers as they explore decision-making, patient
preferences, and best practices for shared decision-
making. use it 75% of the participants reported using
the hospital app for 5 minutes or less during the
consultation. 40% of the participants stated that the
application saves them time, and 30% stated that it is
possible to do it in a real clinical setting, considering
that the application does not save them time or waste
their time. In most cases, participants can check the
application at the designated time, indicating that the
application is complete. These results provide
preliminary evidence of participant satisfaction,
perceived efficacy, and potential clinical
implications. It should be noted that these are
preliminary results and full research results will be
published separately (Benrimoh, 2021).
Aifred is an AI-powered clinical decision support
system for the treatment of major depression. So, find
the presence of Aifred. In particular, it affects the
doctor-patient interaction. 20 psychiatrists and family
physicians attended, and residents completed 2.5
hours of training in a standard clinical trial. All
psychiatric disorders have options to use CDSS and
the patient sample can be defined as mild, moderate,
and severe depression. Data were collected through
patient questionnaires, case studies, and interviews.
Therefore, the results for all 20 participants. Where it
is acceptable and possible to use the device during
treatment. Physicians are willing to rely on artificial
AI4IoT 2023 - First International Conference on Artificial Intelligence for Internet of things (AI4IOT): Accelerating Innovation in Industry
and Consumer Electronics
462