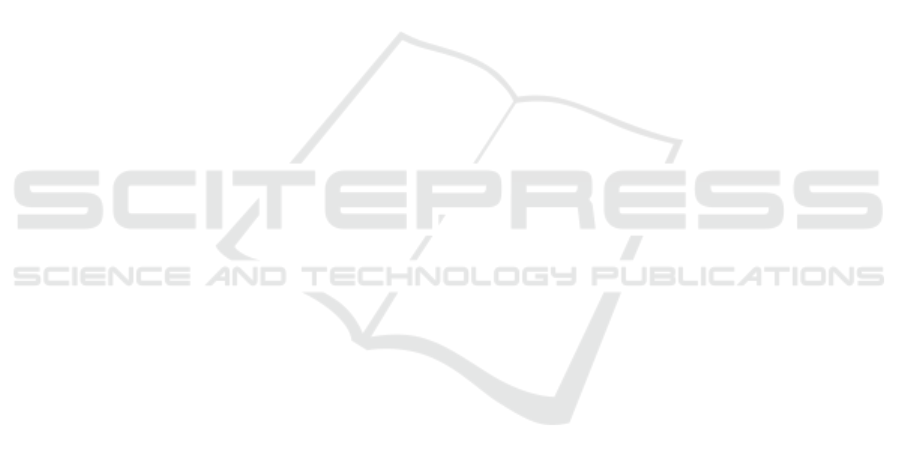
This study's limitations include potential issues
arising when the number of predictions falls short of
the number of observations. If there's a presence of
two or more highly collinear variables, one is chosen
arbitrarily. The method is susceptible to over-
amplification, and insufficient data might not deliver
the anticipated results. Furthermore, not all the
relevant training factors are considered. Looking
forward, the planned work's subsequent phase will
centre on forecasting Black Friday sales using
regression techniques and further refining the
accuracy of the Enhanced XGBoost.
6 CONCLUSION
In the constantly evolving realm of predictive
analytics, especially in the retail sector, accurate
forecasting remains a cornerstone for effective
decision-making. Black Friday, a crucial sales event
for retailers, necessitates precision in predictions to
ensure they remain competitive and meet consumer
demand. In light of our recent research, we assessed
two prominent predictive algorithms: the Enhanced
XGBoost Regression and the Light Gradient
Boosting Machine Regressor, to determine their
efficacy in forecasting Black Friday sales.
Here are six key points stemming from our
analysis:
● Performance Consistency: The Enhanced
XGBoost Regression not only achieved a higher
accuracy but also showed consistent results across
various test scenarios.
● Standard Deviation: The consistency of the
Enhanced XGBoost Regression is further
exemplified by its smaller standard deviation,
indicating lesser variability in its predictions.
● Adaptability to Data: The Enhanced XGBoost
Regression exhibited superior adaptability to
different datasets, making it a more versatile tool
for diverse prediction scenarios.
● Computational Efficiency: Although not
explicitly mentioned earlier, it's worth noting that
algorithms like XGBoost are often designed for
optimized computational efficiency, which can be
crucial for large datasets.
● Collinearity Handling: One inherent strength of
Enhanced XGBoost Regression is its ability to
manage collinear variables more effectively
compared to some other algorithms.
● Integration with Other Tools: The modularity of
the Enhanced XGBoost Regression allows for its
seamless integration with other machine learning
algorithms, enhancing its predictive capabilities
further.
In conclusion, our analysis clearly indicates the
superior performance of the Enhanced XGBoost
Regression over the Light Gradient Boosting
Machine Regressor in predicting Black Friday sales.
While the latter algorithm has an accuracy of 73%,
the former impressively scores 93%. This disparity in
accuracy underscores the potential of the Enhanced
XGBoost Regression, rendering it a more reliable tool
for precise forecasting in the retail industry,
especially for monumental sales events like Black
Friday.
REFERENCES
Arora, Ashish, Bhupesh Bhatt, Divyanshu Bist, Rachna
Jain, and Preeti Nagrath (2021). “Predicting Customer
Spent on Black Friday.” Proceedings of Second
International Conference on Computing,
Communications, and Cyber-Security, 183–201.
AS, Vickram, Raja Das, Srinivas MS, Kamini A. Rao, and
Sridharan TB (2013). "Prediction of Zn concentration
in human seminal plasma of Normospermia samples by
Artificial Neural Networks (ANN)." Journal of assisted
reproduction and genetics 30: 453-459.
Habel, Johannes, Sascha Alavi, and Nicolas Heinitz (2022).
“A Theory of Predictive Sales Analytics Adoption,”
December. https://doi.org/10.2139/ssrn.3994561.
Jandera, Ales, and Tomas Skovranek (2022). “Customer
Behavior Hidden Markov Model.” Science in China,
Series A: Mathematics 10 (8): 1230.
Kishore Kumar, M. Aeri, A. Grover, J. Agarwal, P. Kumar,
and T. Raghu, (2023) “Secured supply chain
management system for fisheries through IoT,” Meas.
Sensors, doi: 10.1016/j.measen.2022.100632.
Lin, Chuyang, Yiwei Huang, Yibing Tan, Xiaocun Lu,
Yujie Zhou, Yifei Liu, Paul Wescott, and Sasha Stoikov
(2020).” February.
https://doi.org/10.2139/ssrn.3533358.
Liu, Zekun, Weiqing Zhang, Xiao Liu, Eitan Muller, and
Feiyu Xiong (2022). “Success and Survival in
Livestream Shopping,” February.
https://doi.org/10.2139/ssrn.4028092.
Lopes, Gustavo (2022). “The Wisdom of Crowds in
Forecasting at High-Frequency for Multiple Time
Horizons: A Case Study of Brazilian Retail Sales.”
Brazilian Review of Finance 20 (2): 77–115.
Manko, Barbara A., and Susheel Tom Jose (2022).
“Gender-Based Shopping And Consumer Purchasing:
‘Sale Ends Today.’” European Journal of Management
and Marketing Studies 7 (4).
https://doi.org/10.46827/ejmms.v7i4.1299.
Moon, Sangkil, Yoonseo Park, and Yong Seog Kim (2019).
“The Impact of Text Product Reviews on Sales.”
European Journal of Marketing 48 (11/12): 2176–97.
AI4IoT 2023 - First International Conference on Artificial Intelligence for Internet of things (AI4IOT): Accelerating Innovation in Industry
and Consumer Electronics
478