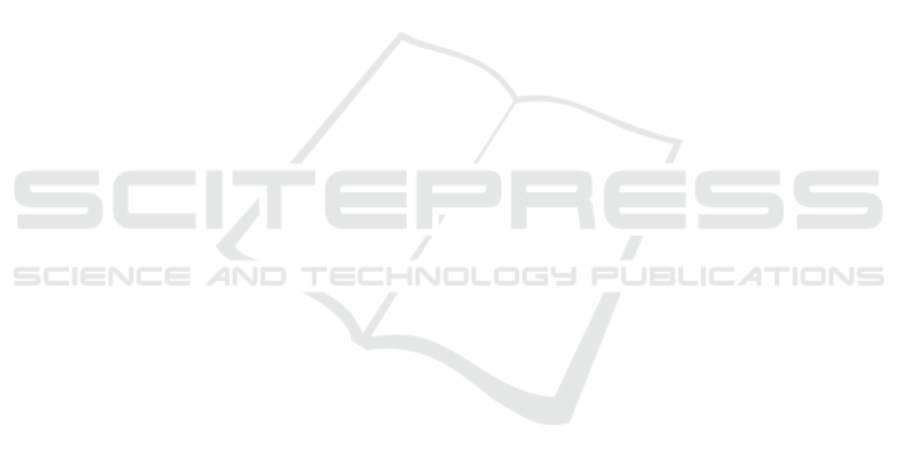
5 CONCLUSION AND FUTURE
WORK
In conclusion, the IoT/AI-based driver sleep detection
and alarm system is a powerful technology that
addresses the critical issue of drowsy driving. By
leveraging sensors, data processing techniques, and
machine learning algorithms, the system can monitor
the driver's behaviour in real-time, detect signs of
drowsiness, and provide timely alerts to prevent
accidents. The system collects data from various
sensors placed within the vehicle, including eye-
tracking cameras, steering angle sensors,
accelerometers, and heart rate monitors. This data is
processed and analyzed using AI algorithms to extract
relevant features indicative of drowsiness. Machine
learning models are trained to classify the driver's
alertness level based on these features.
When drowsiness is detected, the system activates
an alarming mechanism, alerting the driver through
audible, visual, or physical means. This prompt
intervention aims to awaken the driver, increase their
alertness, and prevent accidents caused by
drowsiness-related factors. The IoT/AI-based driver
sleep detection and alarming system not only
enhances driver safety but also contributes to overall
road safety. By proactively addressing the issue of
drowsy driving, it has the potential to save lives,
reduce accidents, and minimize the devastating
consequences of driver fatigue. With further
advancements in IoT and AI technologies, this system
holds promise for continued improvement and
refinement, making roads safer for everyone. By
combining technology and human vigilance, we can
create a future where drowsy driving becomes a thing
of the past, ensuring safer and more secure journeys
for all.
REFERENCES
Hussein, R. M., Miften, F. S., & George, L. E. (2022).
Driver drowsiness detection methods using EEG
signals: a systematic review. Computer Methods in
Biomechanics and Biomedical Engineering, 1–13.
https://doi.org/10.1080/10255842.2022.2112574
Knapik, M., & Cyganek, B. (2019). Driver’s fatigue
recognition based on yawn detection in thermal
images. Neurocomputing, 338, 274–292.
https://doi.org/10.1016/j.neucom.2019.02.014
Liu, W., Qian, J., Yao, Z., Jiao, X., & Pan, J. (2019).
Convolutional Two-Stream Network Using Multi-
Facial Feature Fusion for Driver Fatigue
Detection. Future Internet, 11(5), 115.
https://doi.org/10.3390/fi11050115
You, F., Gong, Y., Tu, H., Liang, J., & Wang, H. (2020). A
Fatigue Driving Detection Algorithm Based on Facial
Motion Information Entropy. Journal of Advanced
Transportation, 2020, 1–17. https://doi.org/10.1155/
2020/8851485
Gwak, J., Hirao, A., & Shino, M. (2020). An Investigation
of Early Detection of Driver Drowsiness Using
Ensemble Machine Learning Based on Hybrid
Sensing. Applied Sciences, 10(8), 2890.
https://doi.org/10.3390/app10082890
Tamanani, R., Muresan, R., & Al-Dweik, A. (2021).
Estimation of Driver Vigilance Status Using Real-Time
Facial Expression and Deep Learning. IEEE Sensors
Letters, 5(5), 1–4. https://doi.org/10.1109/
lsens.2021.3070419
Abbas, Q., & Alsheddy, A. (2020). Driver Fatigue
Detection Systems Using Multi-Sensors, Smartphone,
and Cloud-Based Computing Platforms: A
Comparative Analysis. Sensors (Basel,
Switzerland), 21(1). https://doi.org/10.3390/s21010056
Dong, N., Li, Y., Gao, Z., Ip, W. H., & Yung, K. L. (2019).
A WPCA-Based Method for Detecting Fatigue Driving
From EEG-Based Internet of Vehicles System. IEEE
Access, 7, 124702–124711. https://doi.org/10.1109/
access.2019.2937914
Enhancing Road Safety: An IoT Based Driver Sleep Detection and Alarming System for Accident Prevention
135