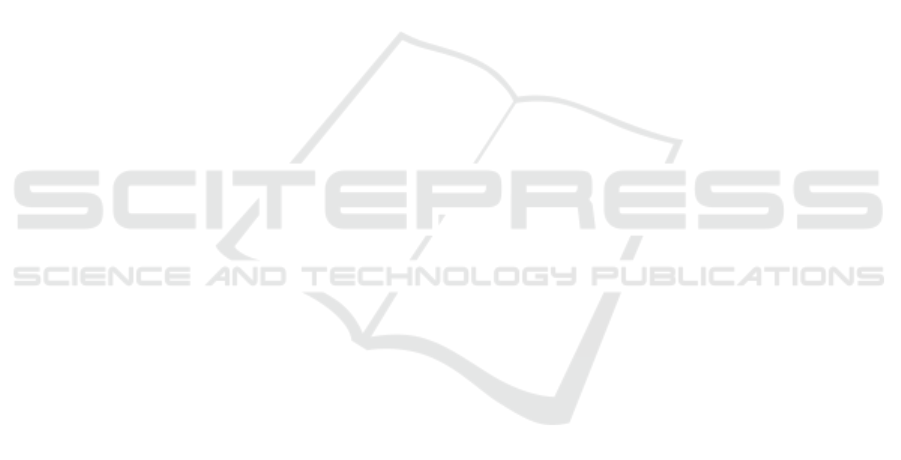
assessment with correlation-based segmentation, the
Superpixel technique exhibited superior performance
in enhancing segmentation accuracy, yielding an
average accuracy score of 88% (Sera Tort. 2020). In
the SPSS statistical analysis, the resultant data
showcased a significantly lower p-value of 0.001%
(p<0.05), indicative of its statistical significance.
Employing the Superpixel method yielded a
segmentation accuracy of 87.06% for white blood
cells in images, whereas the utilization of the
correlation-based segmentation approach resulted in
an accuracy of 74%. These outcomes distinctly
underscore the superior performance of the
Superpixel algorithm (Shahzad et al. 2020). With the
application of the superpixel technique, the accuracy
was determined to be 87.06%, signifying a 13%
enhancement. Comparatively, the accuracy obtained
from a different algorithm (clustering) was measured
at 85% (Deshpande et al. 2022). The segmentation
process, implemented through the Superpixel
algorithm, exhibited a heightened accuracy of 87%,
consequently bolstering precision. Regarding this
research, no contradictory findings were encountered
(Al-Dulaimi et al. 2021).
A limitation of this study lies in the fact that
correlation-based segmentation might necessitate a
substantial volume of training data to achieve precise
WBC segmentation. This requirement could
potentially lead to a time-consuming and costly
process. Furthermore, correlation-based
segmentation could encounter challenges when
dealing with intricate objects, including overlapping
cells or cells exhibiting irregular shapes. In contrast,
super pixel algorithms might demand less training
data and prove to be more time-efficient. Thus, the
super pixel algorithm exhibits superiority over
correlation-based segmentation (Fatichah et al.
2022).
For future endeavours, the focus is on developing
algorithms that can attain high-accuracy
segmentation within a reduced timeframe. The goal is
to produce algorithms capable of achieving optimal
accuracy levels while streamlining the time required
for the segmentation process.
6 CONCLUSION
Through an evaluation of performance metrics and a
comparison of two algorithms, two different
techniques for calculating the accuracy of WBC
segmentation were scrutinized. The results revealed
that the super pixel algorithm achieved a notably
higher mean accuracy of 87% in comparison to the
correlation-based segmentation, which yielded a
mean accuracy of 74%. Consequently, the super pixel
algorithm demonstrated enhanced efficacy in
enhancing image accuracy. Additionally, the standard
deviation for the super pixel algorithm was
determined to be 2.16385, while the correlation-based
segmentation exhibited a higher standard deviation of
2.99625.
REFERENCES
Al-Dulaimi, Khamael, Jasmine Banks, Kien Nguyen,
Aiman Al-Sabaawi, Inmaculada Tomeo-Reyes, and
Vinod Chandran. (2021). “Segmentation of White
Blood Cell, Nucleus and Cytoplasm in Digital
Haematology Microscope Images: A Review-
Challenges, Current and Future Potential Techniques.”
IEEE Reviews in Biomedical Engineering 14
(January): 290–306.
Alharbi, Amal H., C. V. Aravinda, Meng Lin, P. S.
Venugopala, Phalgunendra Reddicherla, and Mohd
Asif Shah. (2022). “Segmentation and Classification of
White Blood Cells Using the UNet.” Contrast Media &
Molecular Imaging 2022 (July): 5913905.
Almassri, Hasan, Tim Dackermann, and Norbert Haala.
(2019). “mDBSCAN: Real Time Superpixel
Segmentation by DBSCAN Clustering Based on
Boundary Term.” Proceedings of the 8th International
Conference on Pattern Recognition Applications and
Methods. https://doi.org/10.5220/0007249302830291.
AS, Vickram, Raja Das, Srinivas MS, Kamini A. Rao, and
Sridharan TB. (2013)"Prediction of Zn concentration in
human seminal plasma of Normospermia samples by
Artificial Neural Networks (ANN)." Journal of assisted
reproduction and genetics 30 (2013): 453-459.
Axel, Leon, Puneet Bhatla, Dan Halpern, Silvia Magnani,
Jadranka Stojanovska, and Chirag Barbhaiya. (2023).
“Correlation of MRI Premature Ventricular Contraction
Activation Pattern in Bigeminy with Electrophysiology
Study-Confirmed Site of Origin.” The International
Journal of Cardiovascular Imaging 39 (1): 145–52.
Burch, Jane, and Sera Tort. (2020). “What Is the Accuracy
of Changes in White Blood Cell (WBC) Count for
Diagnosing COVID-19?” Cochrane Clinical Answers.
https://doi.org/10.1002/cca.3398.
Casler, Kelly Small. (2022). “White Blood Cells and the
Differential Count.” Laboratory Screening and
Diagnostic Evaluation.
https://doi.org/10.1891/9780826188434.0165.
“Classification of Acute Lymphoblastic Leukemia on
White Blood Cell Microscopy Images Based on
Instance Segmentation Using Mask R-CNN.” (2022).
International Journal of Intelligent Engineering and
Systems. https://doi.org/10.22266/ijies2022.1031.54.
Deshpande, Nilkanth Mukund, Shilpa Gite, Biswajeet
Pradhan, Ketan Kotecha, and Abdullah Alamri. (2022).
“Improved Otsu and Kapur Approach for White Blood
Comparison of Superpixel and Correlation Based Segmentation for Improved WBC Segmentation in Microscopic Images
35