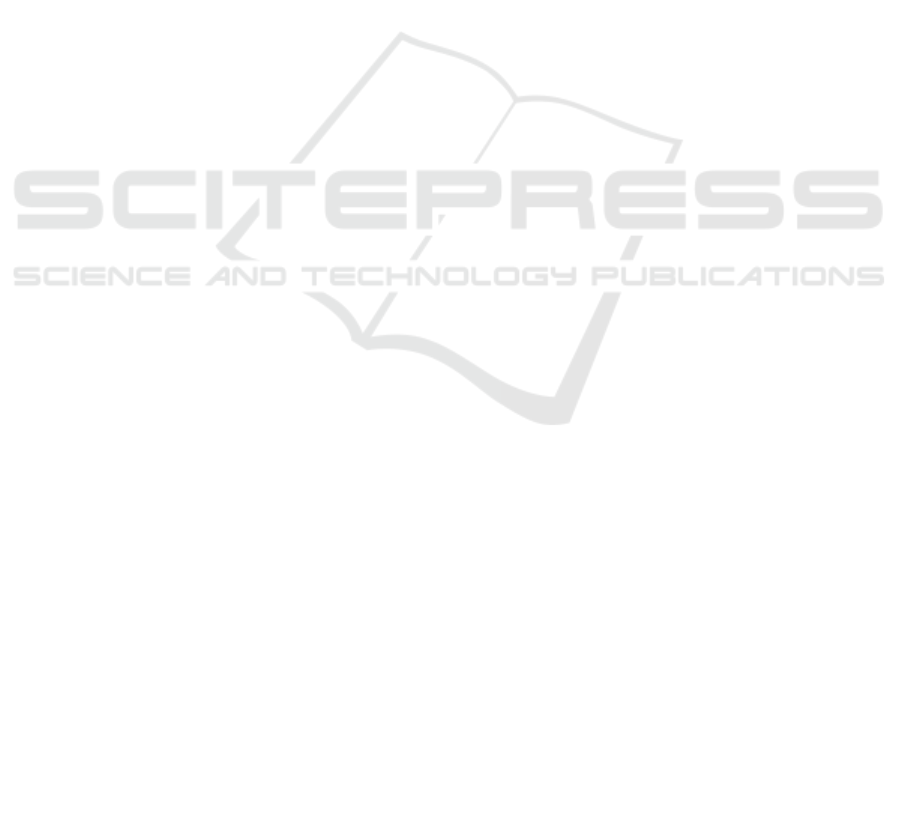
classifier emerged as the most effective, boasting an
accuracy of 96.10%. Chourib et al. (2022) highlighted
that a Tree-Based Method with Random Forest
(TBM-RF) yielded the best results among the feature
selection methods examined in their paper. The
TBM-RF method achieved an accuracy exceeding
85% and an F1-score over 88% when paired with a
decision tree classifier, emphasizing its potency as a
feature selection technique, especially when
combined with a decision tree classifier.
However, the NOKNN algorithm does come with
the drawback of relying on a fixed k value, which can
be computationally demanding, potentially resulting
in suboptimal runtime performance.
Notwithstanding, it offers a superior time complexity
when compared to several other machine learning
algorithms. Future research suggests a pivot towards
enhancing stroke prediction accuracy by integrating
image datasets and investigating methodologies
across various types of stroke and associated risk
levels.
5 CONCLUSION
In recent years, the integration of advanced
algorithms in medical diagnostics has transformed
patient care, making early detection and timely
interventions a reality. This paper's contributions
offer insights into the power of modern computational
techniques in the arena of stroke prediction. As we
move forward, six key points stand out in our
exploration:
● Advanced Techniques: The utilization of
state-of-the-art techniques and algorithms is
crucial in the rapid and precise prediction of
stroke, making early interventions possible.
● Superior Performance: The Novel Optimistic
K-Nearest Neighbor Algorithm (NOKNN)
consistently outperforms traditional methods
such as Logistic Regression, showcasing its
potential in real-world applications.
● Ensemble Techniques: Combining different
methods, as seen with the NOKNN ensemble
approach, further refines prediction accuracy,
leveraging the strengths of multiple
algorithms.
● Risk Factor Identification: Early identification
of risk factors can lead to preventative
measures, drastically reducing the incidence
of stroke in vulnerable populations.
● Comprehensive Analysis: The comprehensive
nature of this analysis, which encompasses
diverse datasets and conditions, speaks to the
versatility of the proposed system.
● Potential for Scalability: With such promising
results, there's significant potential to scale
and adapt this model across various healthcare
settings, offering widespread benefits.
In conclusion, our proposed system marks a
pivotal advancement in the realm of stroke prediction.
By harnessing the power of sophisticated algorithms,
such as NOKNN, we stand at the precipice of a
transformative phase in medical diagnostics. Not only
does this hold the potential to drastically improve
patient outcomes, but it also paves the way for a
reduction in the overall death rate. Embracing these
advanced techniques, our study underscores the
effectiveness of the Novel Optimistic K-Nearest
Neighbor Algorithm, achieving a remarkable
accuracy of 98.5%. This highlights the necessity of
continual research and integration of these tools in the
broader medical community.
REFERENCES
Alqadami, Abdulrahman S. M., Konstanty S. Bialkowski,
Ahmed Toaha Mobashsher, and Amin M. Abbosh.
2019. “Wearable Electromagnetic Head Imaging
System Using Flexible Wideband Antenna Array Based
on Polymer Technology for Brain Stroke Diagnosis.”
IEEE Transactions on Biomedical Circuits and Systems
13 (1): 124–34.
Bandi, Vamsi. 2020. “Stroke_Analysis.” Mendeley Data.
https://doi.org/10.17632/jpb5tds9f6.1.
Bisio, Igor, Claudio Estatico, Alessandro Fedeli, Fabio
Lavagetto, Matteo Pastorino, Andrea Randazzo, and
Andrea Sciarrone. 2018. “Brain Stroke Microwave
Imaging by Means of a Newton-Conjugate-Gradient
Method in <inline-Formula> L^p </inline-Formula>
Banach Spaces.” IEEE Transactions on Microwave
Theory and Techniques 66 (8): 3668–82.
Cheng, Nicholas, Kok Soon Phua, Hwa Sen Lai, Pui Kit
Tam, Ka Yin Tang, Kai Kei Cheng, Raye Chen-Hua
Yeow, Kai Keng Ang, Cuntai Guan, and Jeong Hoon
Lim. 2020. “Brain-Computer Interface-Based Soft
Robotic Glove Rehabilitation for Stroke.” IEEE
Transactions on Bio-Medical Engineering 67 (12):
3339–51.
Coli, Vanna Lisa, Pierre-Henri Tournier, Victorita Dolean,
Ibtissam El Kanfoud, Christian Pichot, Claire
Migliaccio, and Laure Blanc-Feraud. 2019. “Detection
of Simulated Brain Strokes Using Microwave
Tomography.” IEEE Journal of Electromagnetics, RF
and Microwaves in Medicine and Biology 3 (4): 254–
60.
Cui, Yu, Yan Zhang, Xiaolong Zhao, Liming Shao,
Guoping Liu, Chengjian Sun, Rui Xu, and Zhaolong
Zhang. 2021. “ACSL4 Exacerbates Ischemic Stroke by
AI4IoT 2023 - First International Conference on Artificial Intelligence for Internet of things (AI4IOT): Accelerating Innovation in Industry
and Consumer Electronics
42