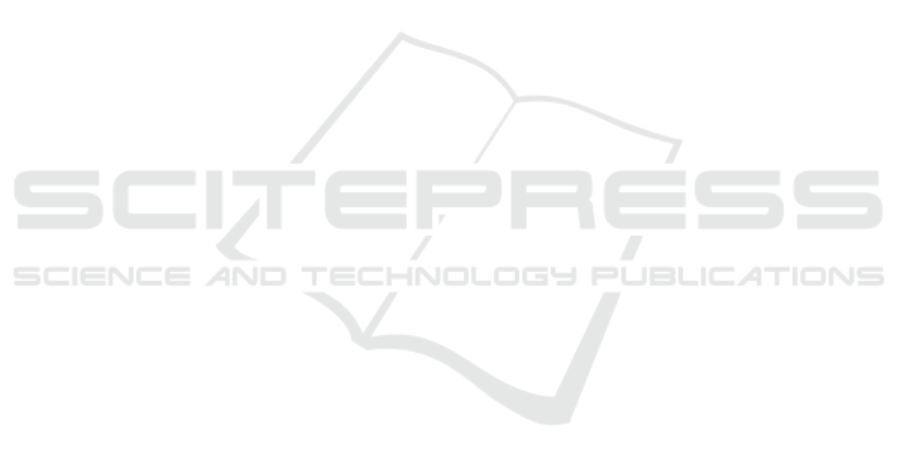
overlooked (Santamaria-Granados, Mendoza-
Moreno, and Ramirez-Gonzalez 2020). Moreover,
tourism recommendation platforms assist businesses
like hotels and tour providers in effectively pairing
travellers with appealing destinations or activities
(Mohamed Elyes Ben Haj Kbaier, 2018).
Collectively, these systems serve as a potent tool for
both travellers and travel-related businesses, fostering
effortless travel experience discovery and crafting
enriching journeys (Torres-Ruiz, 2018). Nonetheless,
some experts argue that these systems might not
consistently yield accurate or trustworthy
recommendations (Petrevska and Koceski, 2012).
This scepticism stems from the system's reliance on
data and algorithms that might not holistically
represent intricate individual preferences, sometimes
achieving accuracy as low as 79% (Shafqat and Byun
2019). Traditional tour planning systems generally
adopt a tripartite structure: delineating tourist
profiles, assessing Points of Interest (POIs), and route
optimisation. Parallel research showcases a
methodology that permits tourists to define their
interests via image collections, facilitating the
system's deduction of their profile. Following the
user's choices, the system consistently amends their
dynamic profile, reaching accuracy levels of 78.4%
(Konstantinos Pliakos, 2015). The system
subsequently curates a resource list, boasting 90%
accuracy, harmonised with both the user's profile and
destination tourist resources (Linaza 2011).
Nonetheless, there's potential system bias towards
specific destinations or activities, which may stem
from user demographic data or underlying
algorithmic biases (Mehrbakhsh Nilashi, 2017).
Tourism recommendation systems are not devoid
of challenges, including noise, erroneous or
unsuitable data. Effective recommendation systems
often necessitate copious user data. In data scarcity,
the recommendations might be imprecise. Future
potential for tourism recommendation systems lies in
furnishing personalised suggestions, interoperability
with various systems, synergy with social media, and
mobile-centric optimisation through innovative
categorisation techniques.
5 CONCLUSION
In conclusion, several salient points have emerged
from the examination of the tourism recommendation
system and the comparison of the AlexNet Classifier
with the SVM algorithm:
● The AlexNet Classifier has proven to be more
effective in tourism recommendations,
achieving a superior accuracy rate of 97.20%.
● The SVM algorithm, while still effective,
lagged behind with an accuracy rate of
92.45%.
● There is a broad consensus among researchers
and industry practitioners that tourism
recommendation systems, harnessing
innovative categorisation techniques,
significantly enhance the traveller experience
by providing tailored suggestions.
● Personalised recommendations, derived from
past behaviours and preferences, enable
travellers to discover novel destinations and
activities that might otherwise be overlooked.
● There's some caution within the industry, with
concerns regarding the potential inaccuracies
of recommendation systems, especially when
there is insufficient or noisy data.
● Future prospects for tourism recommendation
systems include their integration with social
media platforms, optimisation for mobile use,
and their potential to deliver increasingly
tailored recommendations to users.
REFERENCES
Changjie Tang, Charles X. Ling (2007), Advanced Data
Mining and Applications. Springer Berlin Heidelberg.
Mohamed Elyes Ben Haj Kbaier (2017), “A Personalized
Hybrid Tourism Recommender System.”
Mehrbakhsh Nilashi, Karamollah Bagherifard (2017). “A
Recommender System for Tourism Industry Using
Cluster Ensemble and Prediction Machine Learning
Techniques.”
Liangliang Cao; Jiebo Luo, (2010) “A worldwide Tourism
Recommendation System Based on Geotaggedweb
Photos.”
Karthik B and Ramkumar G (2022)" Comparison of
Feature Extraction Technique for Segmentation in
Human Iris Recognition Under Uncontrolled
Environment Using CNN Algorithm with SVM
Classifier" ECS Transactions, doi:
10.1149/10701.16785ecst
Kevin Meehan; Tom Lunney (2013), Context-Aware
Intelligent Recommendation System for Tourism.
Priyadarshiny Dhar, Saibal Dutta (2021)“Cross-Wavelet
Assisted Convolution Neural Network (AlexNet)
Approach for Phonocardiogram Signals
Classification.”
Eleonora Pantano, Constantinos-Vasilios Priporas,
(2019)“Facilitating Tourists’ Decision Making through
Open Data Analyses: A Novel Recommender System.”
Rula A. Hamid, A.S. Albahri, (2021). “How Smart Is E-
Tourism? A Systematic Review of Smart Tourism
Evaluating the Performance of AlexNet and SVM for Tourism Recommendation
49