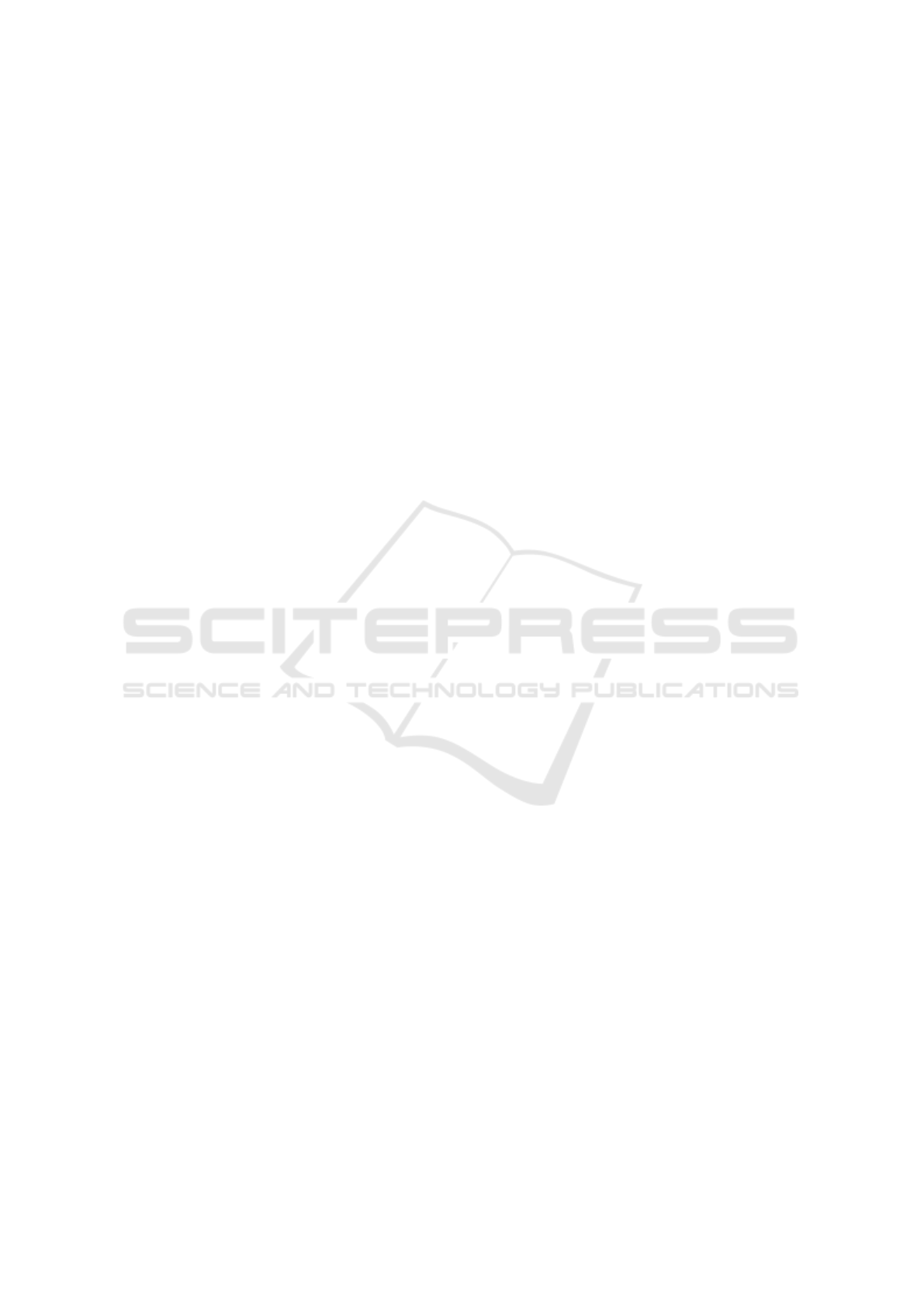
2. Computational Efficiency: Beyond just
accuracy, the computational efficiency of the
Multi-Channel N-gram CNN model was
observed to be noteworthy. This is crucial for
real-time analysis, especially in disaster
management scenarios where time is of the
essence.
3. Generalisation: The higher accuracy suggests
that the Multi-Channel N-gram CNN model
might possess better generalisation
capabilities, making it robust across diverse
datasets.
4. Integration Possibilities: The potential for
integrating the Multi-Channel N-gram CNN
model with other systems or platforms, such
as GIS tools for disaster mapping, emerges as
a promising avenue.
5. Model Evolution: The rapid evolution of the
Multi-Channel N-gram CNN model in recent
years highlights the significance of continuous
research and adaptation in the field of disaster
tweet analysis.
6. Future Enhancements: There's a palpable
potential for further enhancing the Multi-
Channel N-gram CNN model with additional
layers or integrating advanced Natural
Language Processing techniques to better
understand and predict disaster scenarios.
In conclusion, this research work underscores the
prowess of the Multi-Channel N-gram CNN model in
the realm of disaster tweet analysis. The results
unambiguously point to its superior performance,
boasting an impressive accuracy of 97.84%, a marked
improvement over the Glove with Keras Word
embedding model, which recorded an accuracy of
55.06%. This investigation paves the way for future
studies, highlighting the vast possibilities and the
pressing need for optimal tools in disaster
management.
REFERENCES
Asif, Amna, Shaheen Khatoon, Md Maruf Hasan, Majed A.
Alshamari, Sherif Abdou, Khaled Mostafa Elsayed, and
Mohsen Rashwan. (2021). “Automatic Analysis of
Social Media Images to Identify Disaster Type and
Infer Appropriate Emergency Response.” Journal of
Big Data 8 (1): 1–28.
Deena, S. R., Kumar, G., Vickram, A. S., Singhania, R. R.,
Dong, C. D., Rohini, K., ... & Ponnusamy, V. K. (2022).
Efficiency of various biofilm carriers and microbial
interactions with substrate in moving bed-biofilm
reactor for environmental wastewater treatment.
Bioresource technology, 359, 127421.
“Dormant Disaster Organizing and the Role of Social
Media.” 2019. New Media in Times of Crisis.
https://doi.org/10.4324/9780203703632-14.
Ji, Yaguang, Songnian Yu, and Yafeng Zhang. (2011). “A
Novel Naive Bayes Model: Packaged Hidden Naive
Bayes.” 2011 6th IEEE Joint International Information
Technology and Artificial Intelligence Conference.
https://doi.org/10.1109/itaic.2011.6030379.
Kim, Yoon. (2014). “Convolutional Neural Networks for
Sentence Classification,” August.
https://doi.org/10.48550/arXiv.1408.5882.
Kumar, Shamanth, Geoffrey Barbier, Mohammad Abbasi,
and Huan Liu. (2011). “TweetTracker: An Analysis
Tool for Humanitarian and Disaster Relief.”
Proceedings of the International AAAI Conference on
Web and Social Media 5 (1): 661–62.
Kishore Kumar, M. Aeri, A. Grover, J. Agarwal, P. Kumar,
and T. Raghu, (2022) “Secured supply chain
management system for fisheries through IoT,” Meas.
Sensors, vol. 25, no. August, p. 100632, 2023, doi:
10.1016/j.measen.2022.100632.
Mathur, Prerak, Tanu Sharma, and Karan Veer. (2022).
“Analysis of CNN and Feed Forward ANN Model for
the Evaluation of ECG Signal.” Current Signal
Transduction Therapy.
https://doi.org/10.2174/1574362417666220328144453
Maulana, Iqbal, and Warih Maharani. (2021). “Disaster
Tweet Classification Based On Geospatial Data Using
the BERT-MLP Method.” 2021 9th International
Conference on Information and Communication
Technology (ICoICT).
https://doi.org/10.1109/icoict52021.2021.9527513.
“Multimodal Analysis of Disaster Tweets.” n.d. Accessed
December 19, (2022).
https://ieeexplore.ieee.org/document/8919468.
Ninan, Johan. (2022). “The Past, Present and Future of
Social Media in Project Management.” Social Media
for Project Management.
https://doi.org/10.1201/9781003215080-1.
Ningsih, A. K., and A. I. Hadiana. (2021). “Disaster Tweets
Classification in Disaster Response Using Bidirectional
Encoder Representations from Transformer (BERT).”
IOP Conference Series: Materials Science and
Engineering 1115 (1): 012032.
Ramkumar, G. et al. (2021). “An Unconventional Approach
for Analyzing the Mechanical Properties of Natural
Fiber Composite Using Convolutional Neural
Network” Advances in Materials Science and
Engineering vol. 2021, Article ID 5450935, 15 pages,
2021. https://doi.org/10.1155/2021/5450935
Shekhar, Himanshu, and Shankar Setty. (2015). “Disaster
Analysis through Tweets.” In 2015 International
Conference on Advances in Computing,
Communications and Informatics (ICACCI). IEEE.
https://doi.org/10.1109/icacci.2015.7275861.
Sitaula, Chiranjibi, and Tej Bahadur Shahi. (2022). “Multi-
Channel CNN to Classify Nepali Covid-19 Related
Tweets Using Hybrid Features,” March.
https://doi.org/10.48550/arXiv.2203.10286.
AI4IoT 2023 - First International Conference on Artificial Intelligence for Internet of things (AI4IOT): Accelerating Innovation in Industry
and Consumer Electronics
56