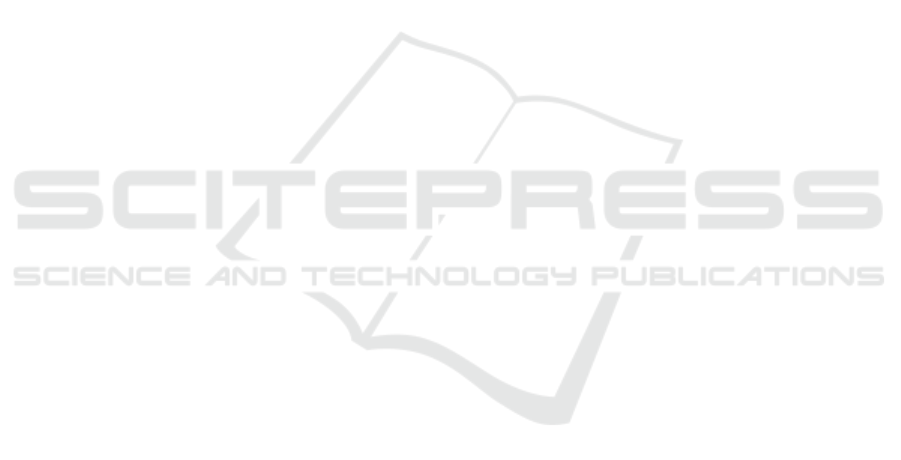
According to a study by Pavlov in 2020 (Pavlov
and New Economic School 2020), the accuracy rate
for Ridge regression is approximately 74.956%. In
contrast, the Novel LASSO delivers more accurate
values with an accuracy rate of 81.95%. With a
comprehensive database spanning 60 years and
covering 268 countries with recorded CPIs, accurate
prediction becomes more feasible. The proposed
model boasts superior accuracy coupled with a lower
processing rate, attributable to the use of extensive
databases. For improved speed and accuracy, smaller
databases are favoured (Ho 1982). Despite the wealth
of data, many researchers have argued that various
predictive models are not designed for accurately
forecasting a country's CPI for a particular year.
Ridge regression's drawbacks include its time-
consuming nature and its less intuitive user interface,
especially when compared to the Novel Least
Absolute Shrinkage and Selection Operator. This
implies that implementing the Ridge regression
algorithm is cumbersome, time-intensive, and
generally inferior to the Novel LASSO regression
algorithm. Looking forward, the Novel LASSO
regression algorithm is poised to be the go-to tool for
running ML models, aiming to forecast inflation rates
and predict economic stability.
5 CONCLUSION
The intricate dynamics of inflation prediction,
especially in the context of the Cost-push inflation
rate, represent a vital area of study in economic
research. The stakes are high: accurate prediction
methodologies can inform policy decisions,
streamline financial forecasting, and foster economic
stability. Our exploration into this domain yielded
several key observations that shaped the findings and
contributed to our understanding of the topic.
● Database Depth: The expansive database
spanning over 60 years and covering 268
countries provided a robust foundation for
analysis. Such a comprehensive data set
ensures that the algorithms can train and test
on varied and representative data, enhancing
the generalisability of the results.
● Impact of Raw Materials: The volatility in the
prices of essential raw materials, such as gold
and petrol, underscores the importance of
accurate inflation prediction. These
fluctuations have a ripple effect on the
economy, affecting wage demands, consumer
prices, and corporate profitability.
● Economic Implications: A misstep in
predicting Cost-push inflation can lead to
adverse economic repercussions. Failing to
account for inflationary pressures can imperil
economic health, affecting trade balances,
purchasing power, and even leading to
recessions.
● Algorithmic Advantages: The Novel LASSO
algorithm's inherent design, which focuses on
both feature selection and regularisation, lends
it an edge over other algorithms. Its ability to
reduce model complexity while retaining
significant variables makes it particularly
effective for complex economic predictions.
● Usability Concerns: Beyond mere accuracy,
the ease of use and processing time of an
algorithm play a significant role in its real-
world application. As observed, Ridge
regression, despite its merits, is more time-
consuming and less user-friendly compared to
Novel LASSO.
● Future Scope: The continual evolution of
machine learning models hints at the
possibility of even more refined and accurate
prediction models in the future. Keeping
abreast of these developments will be crucial
for maintaining the edge in economic
forecasting.
In light of the above points, the outcome of the
present study of Cost-push inflation prediction is
encouraging. With the Ridge regression algorithm
delivering a mean accuracy of 67.86% and the Novel
LASSO algorithm presenting a commendable mean
accuracy of 81.95%, the latter clearly stands out.
Hence, it is concluded that the Novel LASSO
Algorithm exhibits superior accuracy when
juxtaposed with the Ridge regression algorithm. This
distinction, coupled with the aforementioned insights,
paves the way for more informed decisions in
economic modelling and forecasting.
REFERENCES
Bryan, Michael F., and Stephen G. Cecchetti. 1993. “The
Consumer Price Index as a Measure of Inflation.”
w4505. National Bureau of Economic Research.
https://doi.org/10.3386/w4505.
Campos, Chris, Michael McMain, and Mathieu Pedemonte.
2022. “Understanding Which Prices Affect Inflation
Expectations.” Economic Commentary (Federal
Reserve Bank of Cleveland).
https://doi.org/10.26509/frbc-ec-202206.
Choi, Sun, Young Jin Kim, Simon Briceno, and Dimitri
Mavris. 2016. “Prediction of Weather-Induced Airline
Forecasting Cost-Push Inflation with LASSO over Ridge Regression
69