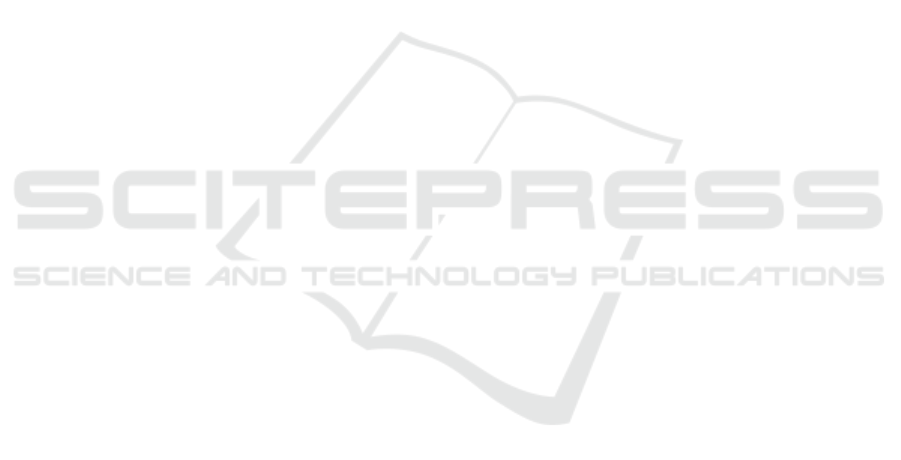
Sannino 2018). The study's lens is trained on a
spectrum of acoustic characteristics derived from
vocal fold signals, chiefly zeroing in on pitch.
Experimentally, the earmarked features have been
adjudged to be of immense import, as the
classification algorithm, even in its unadorned form,
touches an apex accuracy rate of 91.5% (Umapathy et
al. 2005). The VGG-16 CNN model, together with the
Convolutional Neural Network, have been utilised in
this endeavour. The experiment exploited hundreds of
PVD audio files from the Respiratory Sound
Database, exploring the CNN's prowess in
pinpointing aberrant speech. The diagnosis of voice
pathology was discerned with a precision of 92.03%
(Gumelar et al. 2020). The overarching aim of this
scrutiny is to evaluate and draw parallels between
machine learning methods tailored for the precocious
detection of Voice Disorders, even before the
symptoms unfurl. The proposed paradigm has been
validated to clock a staggering 93% accuracy in the
allotted endeavour, employing a conglomerate of
learning models (Hussain and Sharma 2022).
The research methodology wends its way through
data amassed from variegated reservoirs, contending
with the challenge of voice data recognition. Yet, the
study doesn't emerge unscathed from constraints; a
conspicuous drawback is the protracted span
earmarked for dataset training. Envisioning the road
ahead, the research aspires to amplify the system's
ambit, embracing an enlarged cadre of subjects,
whilst concurrently curtailing the duration expended
on dataset training.
7 CONCLUSION
Voice disorders, often neglected in the broader
spectrum of medical issues, are essential for
diagnostics, given the significant role voice plays in
human communication. The advanced machine
learning algorithms we've discussed in this study,
especially the Novel ResNet-50 and ResNet-18, have
the potential to revolutionize this area of diagnosis.
The insights derived from our comparison not only
spotlight the competencies of these algorithms but
also delineate the path ahead for further exploration.
Summarizing the findings, we can highlight six
cardinal points:
• Depth of Algorithm: The layer configuration
in the Novel ResNet-50, with its 50 layers,
provides a depth that seems conducive to
intricate voice analysis, besting the shallower
ResNet-18.
• Handling Vanishing Gradient: The ingenuity
of the Novel ResNet-50 resides in its inventive
approach of adding more convolutional layers
without facing the vanishing gradient
problem, constraint often limiting deep neural
networks.
• Pre-trained Networks: The availability of
pretrained versions, especially for ResNet-18
on extensive databases like ImageNet,
indicates their potential adaptability to diverse
tasks, including voice disorder detection.
• Feature Representation: The networks' ability
to categorise and represent a multitude of
features ensures that they capture the
intricacies of voice patterns, making the
diagnosis precise and accurate.
• Training Time: One trade-off for the increased
accuracy observed in Novel ResNet-50 could
be the training time. As the layers increase, so
does the computation demand, an area where
ResNet-18 might have an advantage.
• Future Applications: Given the efficacy of the
Novel ResNet-50 in voice disorder detection,
it offers promising prospects in other domains
requiring meticulous pattern recognition.
In conclusion, this study pivots around the
comparative analysis of the Novel ResNet-50 and
ResNet-18 in the context of voice disorder detection.
Evidently, the Novel ResNet-50, with an accuracy
metric of 88.70%, outshines the ResNet-18, which
clocks an accuracy of 70.81%. This differential
underscores the robustness and superiority of the
Novel ResNet-50 paradigm over its ResNet-18
counterpart. The comprehensive exploration
furnished in this study not only underscores the
inherent strengths and limitations of each algorithm
but also offers a clarion call to researchers to further
delve into this promising arena.
REFERENCES
Al-Nasheri, Ahmed, Ghulam Muhammad, Mansour
Alsulaiman, Zulfiqar Ali, Khalid H. Malki, Tamer A.
Mesallam, and Mohamed Farahat Ibrahim. 2018.
“Voice Pathology Detection and Classification Using
Auto-Correlation and Entropy Features in Different
Frequency Regions.” IEEE Access 6: 6961–74.
Alvarez, Mauricio, Ricardo Henao, Germán Castellanos,
Juan I. Godino, and Alvaro Orozco. 2006. “Kernel
Principal Component Analysis through Time for Voice
Disorder Classification.” Conference Proceedings: ...
Annual International Conference of the IEEE
Engineering in Medicine and Biology Society. IEEE
AI4IoT 2023 - First International Conference on Artificial Intelligence for Internet of things (AI4IOT): Accelerating Innovation in Industry
and Consumer Electronics
506