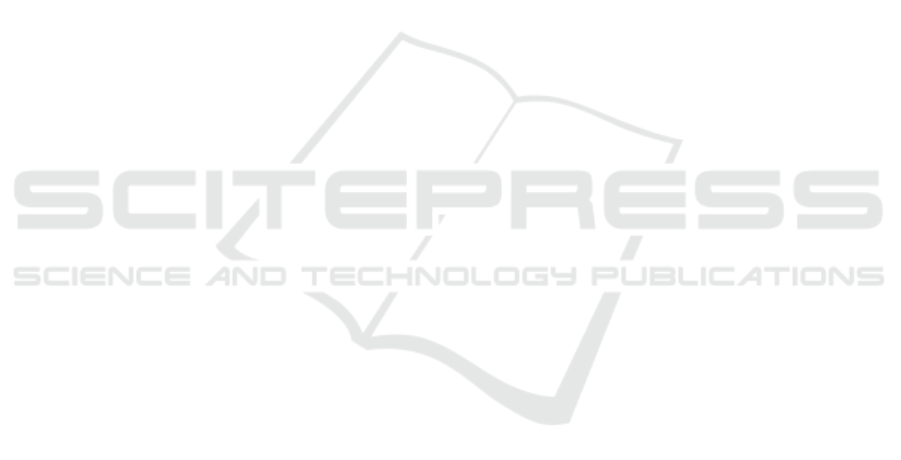
5 DISCUSSION
A substantial accuracy discrepancy of 97.22% was
observed between the SVM classifier and the Novel
HLS algorithm in accurate data prediction. The Novel
HLS approach outperformed the SVM classifier
notably. In contrast to the SVM classifier's accuracy
rate of 70.816%, the Novel HLS method achieved a
significantly higher accuracy rate of 97.221%. The
observed variation in accuracy holds statistical
significance, as indicated by a significance value of
0.012 (P < 0.05) derived from an independent
variable test conducted using the SPSS IBM tool.
This outcome lends weight to the inference that the
observed distinction is statistically meaningful.
Other researchers have reported similar findings,
and the goal of this study is to highlight the latest
advancements in employing neural networks for the
detection and diagnosis of dental caries. The study
delved into research on diverse aspects of neural
networks, including network types, database
attributes, and outcomes. Moreover, the assessment
explored how each study defined and categorised
caries, considering various parameters such as caries
type and the teeth examined (Nanmaran et al. 2022,
Thakur et al. 2024). A precise definition of caries and
the types of lesions under investigation is crucial
before evaluating and comparing research outcomes.
Caries refers to a form of dental decay. Studies
employing ICDAS II displayed accuracy ranging
from 80 to 88.9% (mean SD of 85.45 6.29%).
However, research that defined caries as the loss of
mineralization (radiolucent) achieved an accuracy of
97.1%. In this study, caries was defined as the loss of
mineralization. Nonetheless, 76% of the papers
assessed for this review omitted information about
caries lesion definitions. Another potential bias
source is the dataset used for training. The biomedical
images employed in training need specialist
annotations (Musri et al 2021). Seven of the analysed
studies acknowledged the involvement of examiners
in annotating images, though the level of expertise
and number of examiners varied between
investigations (Manzey et al 2006).
Studies have explored the correlation between
dental experience and caries identification. Bussaneli
et al. concluded that the examiner's expertise didn't
impact the detection of occlusal lesions in primary
teeth, but it did affect prioritization of treated lesions.
An artificial intelligence's performance is restricted
by the quality of input based on human observer
ratings. Articles in this review's scope of examiner-
assisted accuracy had a mean standard deviation of
88.7 8.55%, ranging from 80 to 97%. Results from
research involving four specialists examining images
yielded the second-best outcomes, followed by a
single examiner using standard criteria for caries
identification. Conversely, the least accurate findings
emerged from research with two different examiners
(Budd 2017). Only one study provided information
on researchers' years of expertise, but since these
findings weren't closely correlated with the total
number of examiners (Parziale 2016), it's vital to
consider other factors such as neural network usage,
dataset, and caries definition. Training images can be
time-intensive, potentially impacting accuracy in
some scenarios. This limitation of the study is
mitigated by selecting only necessary database image
features for classification, significantly reducing
training time. Consequently, the potential use of
larger datasets for research becomes viable.
6 CONCLUSION
Based on the results obtained, the Novel HLS
algorithm demonstrated superior accuracy compared
to the established SVM classifier. The research
findings clearly indicate that the Novel HLS
algorithm outperforms the SVM classifier in
accurately predicting data. The accuracy achieved by
the Novel HLS algorithm is notably higher, at
97.22%, whereas the SVM classifier achieved a
comparatively lower accuracy rate of 70.816%.
REFERENCES
V. Machiulskiene, “Nyvad Criteria(2019)for Assessment of
Caries Lesion Activity and Severity,” Detection and
Assessment of Dental Caries. pp. 35–43, 2019. doi:
10.1007/978-3-030-16967-1_5.
B. Abuzenada, (2019) “Detection of proximal caries with
digital intraoral bitewing radiography: An interobserver
analysis,” Saudi Journal for Health Sciences, vol. 8, no.
1. p. 38, 2019. doi: 10.4103/sjhs.sjhs_141_18.
N. Irfan, K. Anwar, A. Taimoor, M. A. Al Absi, M. I. Arain,
and S. Shahnaz, (2020) “Dental Caries assessment of
Rural Population of Sindh by DMFT (Decayed missing
and filled teeth) Index,” The Professional Medical
Journal, vol. 27, no. 01. pp. 100–103, 2020. doi:
10.29309/tpmj/2019.27.01.3454.
Z. Hu, X. Yan, Y. Song, S. Ma, J. Ma, and G. Zhu, (2014)
“Trends of dental caries in permanent teeth among 12-
year-old Chinese children: evidence from five
consecutive national surveys between 1995 and 2014,”
BMC Oral Health, vol. 21, no. 1. 2021. doi:
10.1186/s12903-021-01814-7.
R. M. Prakash, R. Meena Prakash, and R. Shantha Selva
Kumari, (2019)“Classification of MR Brain Images for
AI4IoT 2023 - First International Conference on Artificial Intelligence for Internet of things (AI4IOT): Accelerating Innovation in Industry
and Consumer Electronics
126