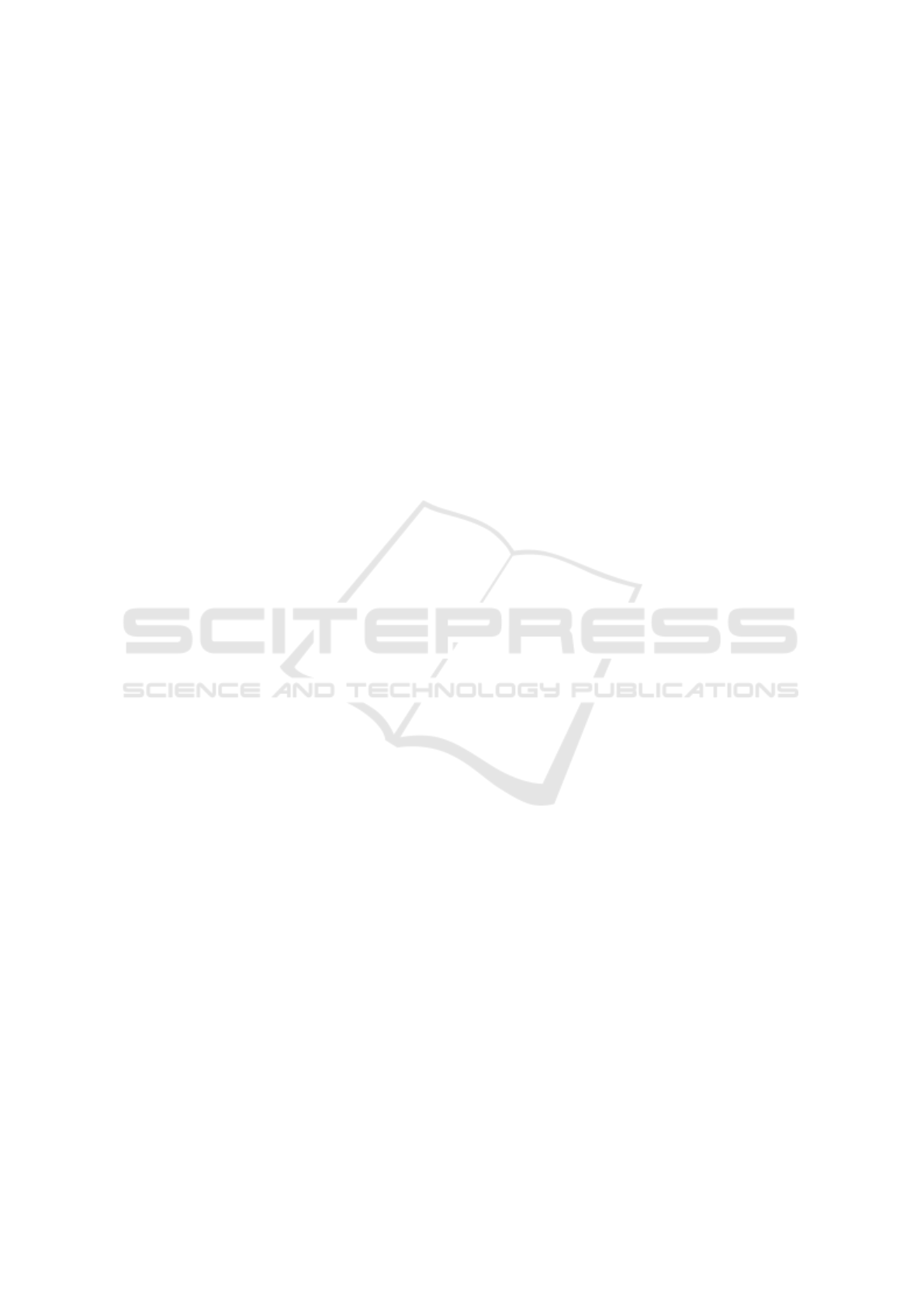
An inherent assumption of LASSO regression is
that asset returns follow a normal distribution, which
might not accurately mirror real-world market
behaviour. Consequently, this assumption can lead to
less precise results in portfolio optimization, which
could be a drawback for investors aiming for higher
accuracy (Hyndman, R.J. and Athanasopoulos, G.,
2018).
On a positive note, LASSO regression is often
appreciated for its simplicity and ease of
comprehension. This aspect is advantageous for
investors who might not be well-versed in more
complex models, as indicated in Table 1.
Furthermore, LASSO regression is
computationally efficient and can perform well even
with smaller datasets. This efficiency could be an
advantage for investors with limited access to
extensive data or computational resources, as
highlighted in Table 3. The study's scope might be
limited due to data availability, as both modern
portfolio theory using time series models and LASSO
regression demand substantial data to yield accurate
outcomes. Both approaches also rest on specific
assumptions about market behaviour, which may not
always hold true in real-world scenarios.
Generalizing the study's findings to different markets
or time periods might be challenging, given the
substantial variations in market conditions, as
depicted in Figure 2.
A prospective avenue for LASSO Regression's
expansion is its application to other asset classes like
commodities or real estate. This extension could
assess the models' effectiveness in diverse markets.
Moreover, exploring the integration of alternative
machine learning techniques, such as neural networks
or different forms of LASSO Regressions, could
provide insights into their comparative performance.
Comparative evaluations of the proposed models
against other risk management strategies, like value
at risk or conditional value at risk, could offer insights
into the most efficient risk management approach.
While various predictive models have been
developed (Hyndman and Athanasopoulos, 2018),
not all of them accurately predict favourable stock
market movements. Despite their potential, many
time series models, like the one in "Predicting
portfolio returns using the distributions of efficient set
portfolios" (2003a), tend to be less user-friendly and
time-consuming. This suggests that while time series
models have the potential to enhance stock market
forecasts, their computational demands and
complexity might remain limiting factors (2003b,
"Predicting portfolio returns using efficient set
portfolio distributions").
6 CONCLUSION
The study's results revealed that Modern Portfolio
Theory using time series models exhibited superior
performance over LASSO regression in both risk
management and portfolio optimization accuracy.
The Time Series Model demonstrated a notably
higher accuracy rate of 90.1252% compared to the
LASSO Regression algorithm's accuracy of
80.1423%. The statistical significance of these
accuracy values, coupled with the associated p-value
of 0.000 (p<0.05), underscores the substantial
advantage of the Time Series Model in terms of
accuracy and predictive capability.
REFERENCES
Chatfield, C. (2013) The Analysis of Time Series: Theory
and Practice. Springer.
Dey, N., Vickram, S., Thanigaivel, S., Subbaiya, R., Kim,
W., Karmegam, N., & Govarthanan, M. (2022).
Nanomaterials for transforming barrier properties of
lignocellulosic biomass towards potential applications–
A review. Fuel, 316, 123444.
G. R et al, "Uncompressed digital video watermarking
using stationary wavelet transform," (2014) IEEE
International Conference on Advanced
Communications, Control and Computing
Technologies, Ramanathapuram, India, 2014, pp. 1252-
1258, doi: 10.1109/ICACCCT.2014.7019299.
Hyndman, R. J. and Athanasopoulos, G. (2018)
Forecasting: principles and practice. OTexts.
Kassambara, A. (2018) Machine Learning Essentials:
Practical Guide in R. STHDA.
Lohmeyer and Lohmeyer (no date) ‘Time series analysis
under model uncertainty’. Available at:
https://doi.org/10.26481/dis.20190524jl.
Madsen, H. (2007) Time Series Analysis. CRC Press.
Md. Ehsanes Saleh, A., Arashi, M. and Golam Kibria, B.M.
(2019) Theory of Ridge Regression Estimation with
Applications. John Wiley & Sons.
Parzen, E. (1981) ‘Time Series Model Identification and
Prediction Variance Horizon’, Applied Time Series
Analysis II, pp. 415–447. Available at:
https://doi.org/10.1016/b978-0-12-256420-8.50019-8.
Parzen, E. (1983) ‘Time Series Model Identification By
Estimating Information’, Studies in Econometrics, Time
Series, and Multivariate Statistics, pp. 279–298.
Available at: https://doi.org/10.1016/b978-0-12-
398750-1.50019-x.
‘Predicting portfolio returns using the distributions of
efficient set portfolios’ (2003a) Advances in Portfolio
Construction and Implementation, pp. 342–355.
Available at: https://doi.org/10.1016/b978-075065448-
7.50018-5.
Precise Portfolio Optimization Based on Novel Modern Portfolio Theory Using Time Series Model Compared with LASSO Regression
139