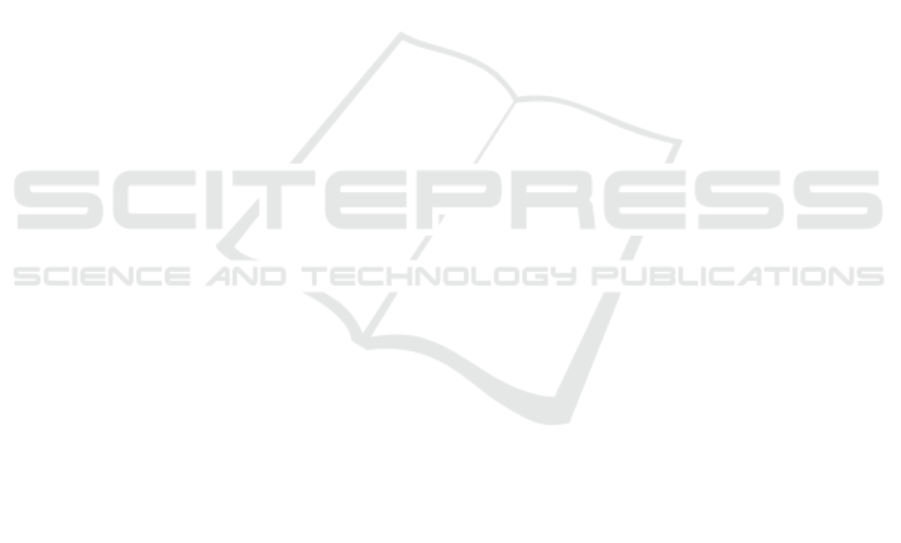
• Comparison with Prevailing Systems: It is
essential to note that pre-existing systems using
both Random Forest and Convolutional Neural
Networks exhibited lower accuracy rates of
85.2% and 79.3% respectively. The evident
improvements in the present study, therefore,
indicate a substantial enhancement in the
technological approach.
• Potential Applications: The significant accuracy
achieved by the Random Forest algorithm in
converting soft murmurs can have wide-ranging
applications, particularly in security or
healthcare sectors where whispered commands
or murmured patient responses need to be
deciphered accurately.
• Future Considerations: Even though Random
Forest has displayed commendable results, it's
pertinent to remember its few limitations when
handling sequential data. Future research might
focus on optimising these aspects or combining
it with other algorithms for even more refined
results.
In conclusion, the transformation of Soft Spoken
Murmur to Normal Speech attained an impressive
level of accuracy, particularly with the Random
Forest algorithm registering a 99.86% accuracy rate.
This clearly overshadowed the performance of the
Convolutional Neural Network algorithm, which
marked an accuracy of 95.89%. The findings not only
advocate for the potential superiority of ensemble
methods in certain contexts but also underscore the
value of continual research and iteration in the
evolving field of speech technology.
REFERENCES
AS, Vickram, Raja Das, Srinivas MS, Kamini A. Rao, and
Sridharan TB. "Prediction of Zn concentration in
human seminal plasma of Normospermia samples by
Artificial Neural Networks (ANN)." Journal of assisted
reproduction and genetics 30 (2013): 453-459.
Babani, Denis, Tomoki Toda, Hiroshi Saruwatari, and
Kiyohiro Shikano. 2011. “Acoustic Model Training for
Soft Spoken Murmur Recognition Using Transformed
Normal Speech Data.” 2011 IEEE International
Conference on Acoustics, Speech and Signal
Processing (ICASSP).
https://doi.org/10.1109/icassp.2011.5947535.
Enireddy, Vamsidhar, C. Karthikeyan, Kumar T. Rajesh,
and Ashok Bekkanti. 2021. “Compressed Medical
Image Retrieval Using Data Mining and Optimized
Recurrent Neural Network Techniques.” Machine
Vision Inspection Systems, Volume 2.
https://doi.org/10.1002/9781119786122.ch13.
Ganapathy, Sriram, and Vijayaditya Peddinti. 2018. “3-D
CNN Models for Far-Field Multi-Channel Speech
Recognition.” 2018 IEEE International Conference on
Acoustics, Speech and Signal Processing (ICASSP).
https://doi.org/10.1109/icassp.2018.8461580.
Heracleous, Panikos, and Norihiro Hagita. 2010. “Non-
Audible Murmur Recognition Based on Fusion of
Audio and Visual Streams.” Interspeech 2010.
https://doi.org/10.21437/interspeech.2010-717.
Heracleous, Panikos, and Akio Yoneyama. 2019. “A
Comprehensive Study on Bilingual and Multilingual
Speech Emotion Recognition Using a Two-Pass
Classification Scheme.” PloS One 14 (8): e0220386.
Kumar, R., C. M. Velu, C. Karthikeyan, S. Sivakumar, S.
Nimmagadda, and D. Haritha. 2020. “Taylor Dirichlet
Process Mixture For Speech Pdf Estimation And
Speech Recognition.” Advances in Mathematics:
Scientific Journal.
https://doi.org/10.37418/amsj.9.10.93.
Kumar, T. Rajesh, T. Rajesh Kumar, Dama Anand, G.
Rama Krishna Srinivas, Debnath Bhattacharyya, and
Hye-Jin Kim. 2016. “Effort Monitoring and Tracking
System.” International Journal of Multimedia and
Ubiquitous Engineering.
https://doi.org/10.14257/ijmue.2016.11.12.34.
Kumar, T. Rajesh, T. Rajesh Kumar, S. Padmapriya, V.
Thulasi Bai, P. M. Beulah Devamalar, and G. R. Suresh.
2015. “Conversion of Non-Audible Murmur to Normal
Speech through Wi-Fi Transceiver for Speech
Recognition Based on GMM Model.” 2015 2nd
International Conference on Electronics and
Communication Systems (ICECS).
https://doi.org/10.1109/ecs.2015.7125023.
Kishore Kumar, M. Aeri, A. Grover, J. Agarwal, P. Kumar,
and T. Raghu, “Secured supply chain management
system for fisheries through IoT,” Meas. Sensors, vol.
25, no. August 2022, p. 100632, 2023, doi:
10.1016/j.measen.2022.100632.
Kumar, T. Rajesh, T. Rajesh Kumar, G. R. Suresh, and S.
Kanaga Subaraja. 2019a. “Conversion of Non-Audible
Murmur to Normal Speech Based on FR-GMM Using
Non-Parallel Training Adaptation Method.” 2019
International Conference on Intelligent Sustainable
Systems (ICISS).
https://doi.org/10.1109/iss1.2019.8908045.
G. Ramkumar and M. Manikandan, "Uncompressed digital
video watermarking using stationary wavelet
transform," 2014 IEEE International Conference on
Advanced Communications, Control and Computing
Technologies, Ramanathapuram, India, 2014, pp. 1252-
1258, doi: 10.1109/ICACCCT.2014.7019299.
2019. “Conversion of Non-Audible Murmur to Normal
Speech Based on FR-GMM Using Non-Parallel
Training Adaptation Method.” 2019 International
Conference on Intelligent Sustainable Systems (ICISS).
https://doi.org/10.1109/iss1.2019.8908045.
“Conversion of Non-Audible Murmur to Normal Speech
Based on FR-GMM Using Non-Parallel Training
Adaptation Method.” 2019 International Conference
AI4IoT 2023 - First International Conference on Artificial Intelligence for Internet of things (AI4IOT): Accelerating Innovation in Industry
and Consumer Electronics
606