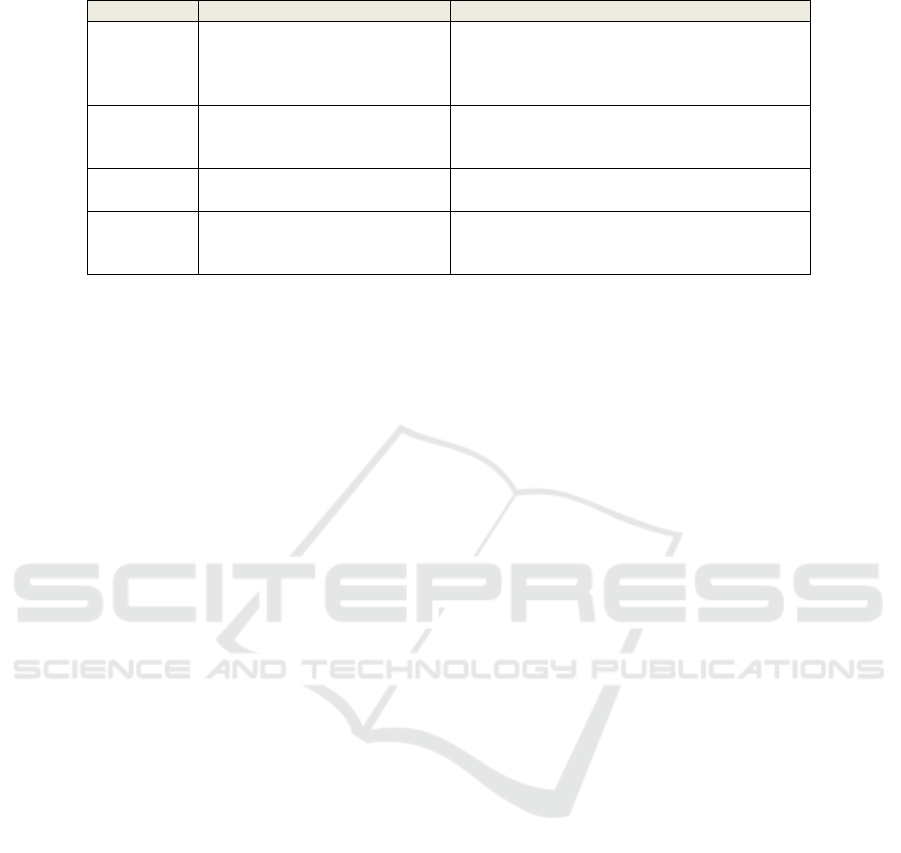
Table 2: Different Fusion Strategies.
MRI, CT, ULTRA SOUND,
MAMMOGRAM, PET
MORPHOLOGY FILTERA,
LEARNING SYSTEMS,
EXPERT SYSTEMS
CT, PET, MRI, ULTRA
SOUND, SPECT
DISCRETE WAVELET TRANSFORMS,
STATIONARY WAVELET, MULTI-
WAVELET TRANSFORM
CT, PET, MRI, ULTRA
SOUND, MRA, SPECT
NEURAL NETWORKS, CLUSTERING
NEURAL NETWORKS
CT, PET, MRI, ULTRA
SOUND, MRA, SPECT
IMAGE FUZZIFICATION,
DEFUZZIFICATION, NEURO FUZZY
NETWORKS,
3 CONCLUSION
The field of medical diagnostics and monitoring is
rapidly advancing with the growth of latest
technologies and scientific advancements. However,
the use of medical images to aid in these processes is
not without challenges. These challenges can be
technological, scientific, and societal in nature.
One of the challenges is related to the quality of
imaging features. In order to achieve a
comprehensive understanding of a medical condition,
multiple imaging modalities are often used. However,
these modalities may produce images with different
qualities and characteristics. Image fusion techniques
can be used to improve the quality of imaging features
by integrating information from multiple modalities.
However, the key challenge in applying image
fusion algorithms to medical images is to confirm that
the medical relevance is maintained and that they aid
in achieving enhanced clinical outcomes. This
requires careful consideration of the specific medical
application, as well as the imaging techniques used.
Despite these challenges, image fusion techniques
hold great promise for improving the quality of
medical imaging and aiding in diagnostics and
monitoring of medical conditions. As such, ongoing
research in this area is critical for the advancement of
medical science and for the betterment of patient care.
REFERENCES
Wang, Z., Ziou, D., Armenakis, C., Li, D., & Li, Q. (2005).
A comparative analysis of image fusion methods. IEEE
transactions on geoscience and remote sensing, 43(6),
1391-1402.
Prabu, S. M., & Ayyasamy, S. (2014). An efficient
watermarking algorithm based on DWT and FFT
approach. International Journal on Computer Science
and Engineering, 6(6), 211.
Zitova, B., & Flusser, J. (2003). Image registration
methods: a survey. Image and vision computing,
21(11), 977-1000.
http://ijariie.com/AdminUploadPdf/Medical_Image_Fusio
n_Using_Combine_Approach_of_DWT_and_DRT_ija
riie2635.pdf
Holende et al., A special issue on biologically inspired
information fusion, Inform. Fus. 11 (1) (2010) 1.
M.C. Casey, R.I. Damper, Editorial: special issue on
biologically-inspired information fusion, Inform. Fus.
11 (1) (2010) 2–3.
Aribi, W., Khalfallah, A., Bouhlel, M. S., & Elkadri, N.
(2012, March). Evaluation of image fusion techniques
in nuclear medicine. In 2012 6th International
Conference on Sciences of Electronics, Technologies of
Information and Telecommunications (SETIT) (pp.
875-880). IEEE.
Jing, L. (2009). Scrambling analysis of ciliates (Doctoral
dissertation, Master’s thesis, University of
Saskatchewan, Saskatoon).
J. Greensmith, U. Aickelin, G. Tedesco, Information fusion
for anomaly detection with the dendritic cell algorithm,
Inform. Fus. 11 (1) (2010) 21–34.
Lawson, K. A., Sousa, C. M., Zhang, X., Kim, E., Akthar,
R., Caumanns, J. J., ... & Moffat, J. (2020). Functional
genomic landscape of cancer-intrinsic evasion of
killing by T cells. Nature, 586(7827), 120-126.
Gomathi, P. S., & Kalaavathi, B. (2016). Multimodal
medical image fusion in non-subsampled contourlet
transform domain. Circuits and Systems, 7(8), 1598-
1610.
Mishra, H. O. S., & Bhatnagar, S. (2014). MRI and CT
image fusion based on wavelet transform. International
Journal of Information and Computation Technology,
4(1), 47-52.
Hill, P. R., Bull, D. R., & Canagarajah, C. N. (2005,
September). Image fusion using a new framework for
complex wavelet transforms. In IEEE International
Conference on Image Processing 2005 (Vol. 2, pp. II-
1338). IEEE.
A Comprehensive Analysis of Medical Image Fusion Techniques: A Detailed Review
151