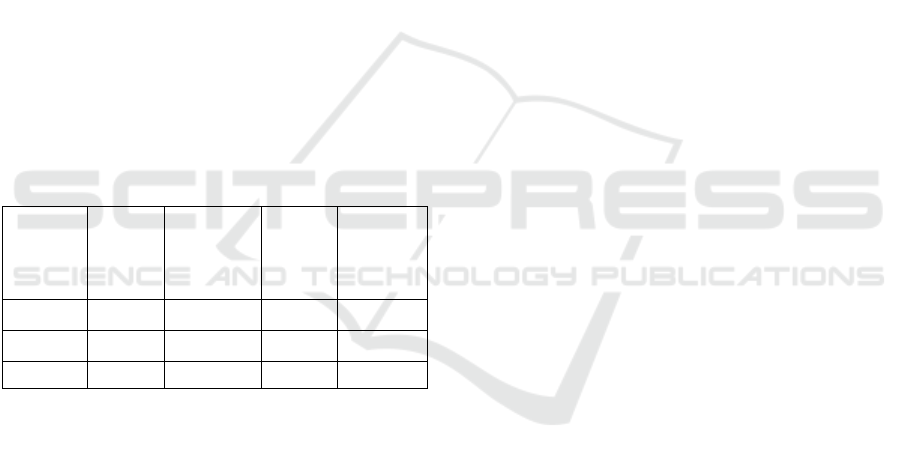
include measures such as system availability,
Accuracy, and busy period in show table 2 included.
Data preprocessing: Clean and preprocess the
data, splitting it into training, validation, and test sets.
Normalize the input variables to ensure that they are
on the same scale.
Model selection: Choose appropriate deep
learning optimization techniques (Adam, SGD, RMS
prop) for the sensitivity analysis. Some options
contain feed forward neural systems, convolutional
neural systems, and regular neural networks.
Consider influences such as the size of the dataset, the
difficulty of the input-output connection, and the
computational capitals existing.
Model training: Train the selected model on the
training data. Use techniques such as stochastic
gradient descent and back propagation to minimize
the bust time. Monitor the performance of the model
on the validation data, and adjust the hyper
parameters as needed.
Model evaluation: Assess the qualified model on
the test data. Calculate metrics such as mean absolute
bust time and mean squared error to assess the
model's performance of deep learning optimization in
show table 1 and table 2.
Table 2: Performance of model.
F1 Score
(Expected
Number of
serverby the
repair man)
Sensitivity analysis: Use the trained model to
perform sensitivity analysis on the input parameters.
Vary the value of one input parameter at a time while
holding the others constant. Record the effect on the
output metric of interest. Repeat this process for each
participation parameter to determine the sensitivity of
the output metric to changes in each parameter.
Interpretation of results: Analyze the fallouts of
the sensitivity examination to identify which input
limits must the utmost impact on the output metric of
interest. Use practices such as article importance and
incomplete dependence plots to advance insights into
the association amid the input limits and output
metric.
Overall, performing reliability and availability of
non markovian single unit redundant system using
deep learning involves a combination of data
collection, preprocessing, model selection, training,
evaluation, and analysis.
It can be a commanding tool for understanding the
influences that underwrite to the reliability of the
system.
The results and discussion of a reliability and
availability of non markovian single unit redundant
system using deep learning will depend on the
specific system and dataset analysed. However, here
are some general insights that could be gained from
such an analysis:
Identification of critical system parameters: The
sensitivity analysis could reveal which input
parameters require the greatest effect on the output
metric of interest.
Understanding of the non-linear relationship
amongst input strictures and output metrics: The deep
learning model used in the analysis can capture non-
linear relationships amongst input restrictions and
output metrics, which could not be detected using
traditional statistical methods.
Validation of existing models and assumptions:
The results of the sensitivity analysis can be used to
validate or challenge existing models and
assumptions about the system.
Prediction of system behavior under different
scenarios: The deep learning model can be used to
predict system performance under different setups,
such as vagaries in operating conditions or
maintenance schedules.
Overall, sensitivity analysis of system parameters
of a reliability and availability of non markovian
single unit redundant system using deep learning can
provide valuable insights into the factors that affect
system performance, Accuracy (MTSF), Expected
Number of Check-ups by the repair man, Busy
Period and Availability of the System and results
in show in figure 2, 3, 4 and 5.
Accuracy between the different model is Adam is
best performance among them. And busy time of
Adam is better among them of model.
6 CONCLUSION
The results of the sensitivity analysis can be used to
validate or challenge existing models and
assumptions about the system. For example, the
analysis could show that a certain parameter has a
much greater impact on system performance than
previously thought. It can help optimize maintenance
strategies, improve system design, and reduce
downtime and maintenance costs.
Reliability and Availability Analysis of Non-Markovian Single Unit Redundant System with Server Failure
575