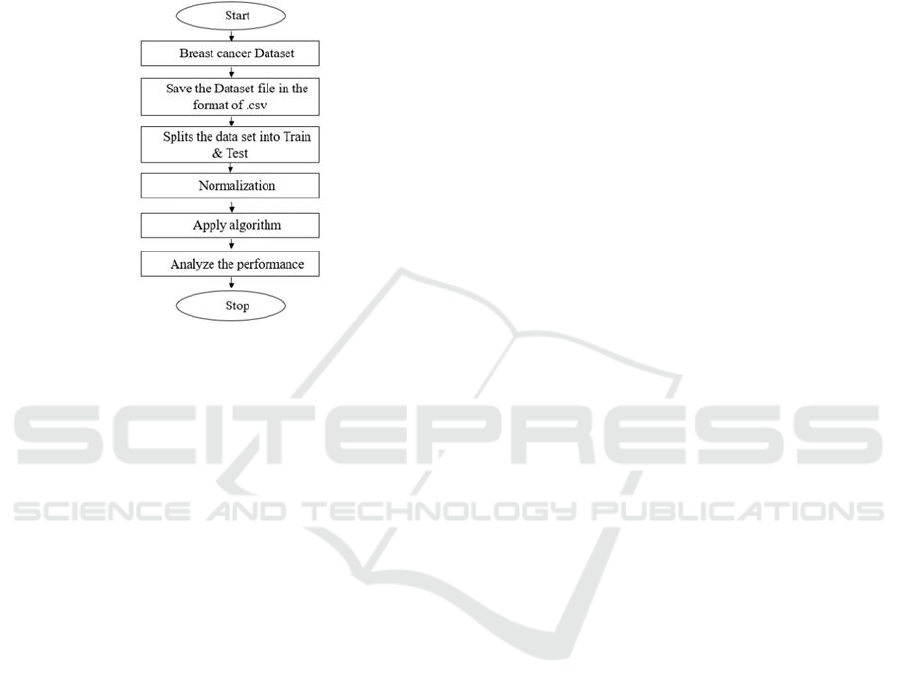
3 METHODOLOGY
The main objective of the work is to assess the
effectiveness of the two algorithms that were selected
for the investigation and identify which algorithm is
most suitable. The second objective is to conduct an
investigation into Breast cancer using particular data.
Figure 1: Flow chart of the proposed method.
The Breast Cancer dataset was used to implement
the study using SVM (Support Vector Machine
Algorithm). Among the R-based classification
approaches available, SVM was chosen because of its
quick findings and high accuracy level.
Here the data set for this classification has been
taken from Breast Cancer Wisconsin (Diagnostic)
Data Set (WBC), from UCI machine learning
repository is a classification dataset, which records
the measurements for Breast Cancer Cases.
4 RESULTS AND DISCUSSIONS
This research report analysed classification systems
and offered a basis for accuracy percentage
comparisons between them. The confusion matrix is
used to calculate the effectiveness level.
And the R programming language, which is
supported by the R core and used for statistical
computing and graphics, was utilized to implement it.
One of the most popular programming languages for
data research is R. R comes with a large number of
pre-installed packages, and each of these packages
contains a collection of functions for various analyses
and graphical displays.
Support Vector Machine (SVM) algorithm has
been applied since its accuracy level and working
process is better than the other algorithm used for the
study, The algorithm known as supervised machine
learning (SVM) evaluates and divides data into one
or more categories. Since SVM can handle both
classification and regression on both linear and non-
linear data, it is one of the machine learning
techniques we use. It is used in applications like
Recognition, Detection, and Classification. Naive
Bayes Algorithm is kind of simple probabilistic
Bayesian-Based classification technique. Statistical
independence is utilised by a family of machine
learning algorithms, not by a single one. This is
mainly used for text classification. Statistical
classifiers include, for example, Bayesian classifiers.
They are able to forecast the likelihood that a
given set of data will belong to a certain class. The
cornerstone of Bayesian classification is the Bayes
theorem. The results of the study are as follows from
the algorithm SVM. At first the overall frequency
level is analysed from the dataset, to know the
frequency level in both Benign & Malignant. Figure
2a shows the overall average of women affected in
Benign & Malignant. This means there is a 1in 10
chance a woman will have breast cancer and this also
means there is 8 in 10 chance a woman will won’t be
affected by Breast cancer. The incidence of
Malignant increases in 2
nd
decade to 5
th
decade and
100% in the 8
th
decade. The incidence of Malignant
increases with age with a maximum incidence in the
older age group. Figure 2b shows Correlation Matrix
is a graphical display to find potential relationships
between variables and to understand the strength of
these relationships. This is an effective tool for
compiling a sizable dataset and for finding and
displaying data trends. Figure 2c shows the Concavity
of Benign vs Malignant, where Concavity in medical
term mean the ranges from the stage 1 to stage 4,
which mean the cancer has spread more. So, this
result shows the range of Malignant is higher than the
range of Benign. Since the concavity mean is most
important attribute in breast cancer, indicates the
shape & color to identify the disease. Concave
represents the number of indentations present on the
nuclear border.
Figure 2d shows Fractional Dimension in breast
cancer analyses the breast tissue specimens provides
a clearance of tumor growth patterns. It is an
objective and measure of the complexity of the tissue
of specimen. This helps to shoe how scaling changes
a model or modelled object. This have been measured
using the formula D=log N/log S. This has to be
measured to see how completely the fractals embed
themselves.
Prediction of Breast Cancer Using Classification Algorithms
277